Malaria Parasite Classification from RBC Smears Using Lightweight Parallel Depthwise Separable CNN and Ridge Regression ELM by Integrating SHAP Techniques
Research Square (Research Square)(2023)
摘要
Abstract Malaria is a significant health concern worldwide, and early detection and accurate classification are essential for better treatment. This study proposes a new method that combines a lightweight parallel depth-wise separable convolutional neural network (LPDCNN) with a hybrid ridge regression extreme learning machine (RELM) to classify images of infected and uninfected patients' red blood cells (RBCs). We include a hybrid pre-processing step that uses contrast-limited adaptive histogram equalization (CLAHE) and Dilation operation to enhance image quality, reduce cell noise, and improve visual acuity. The LPDCNN extracts discriminative features efficiently with only 0.36 million parameters and 8 layers, minimizing computational complexity. The hybrid RELM model improves classification performance and replaces the traditional pseudoinverse of the ELM approach. Rigorous five-fold cross-validation (CV) for binary class classifications shows that the framework has impressive average precision, recall, f1, accuracy, and AUC scores of 99.86±0.08%, 99.88±0.084%, 99.84±0.089%, 99.85±0.071%, and 99.96±0.037%, respectively, surpassing state-of-the-art (SOTA) models. The proposed framework is exceptionally efficient, with an average training and testing time of 0.1376 and 0.00255 seconds, respectively. Additionally, the framework is integrated SHAP (Shapley Additive Explanations) to enhance interpretability, providing valuable insights into decision-making and instilling confidence in malaria diagnosis for real-world applications. This comprehensive approach holds promise in improving malaria diagnosis and patient outcomes worldwide.
更多查看译文
关键词
ridge regression elm,rbc smears,classification
AI 理解论文
溯源树
样例
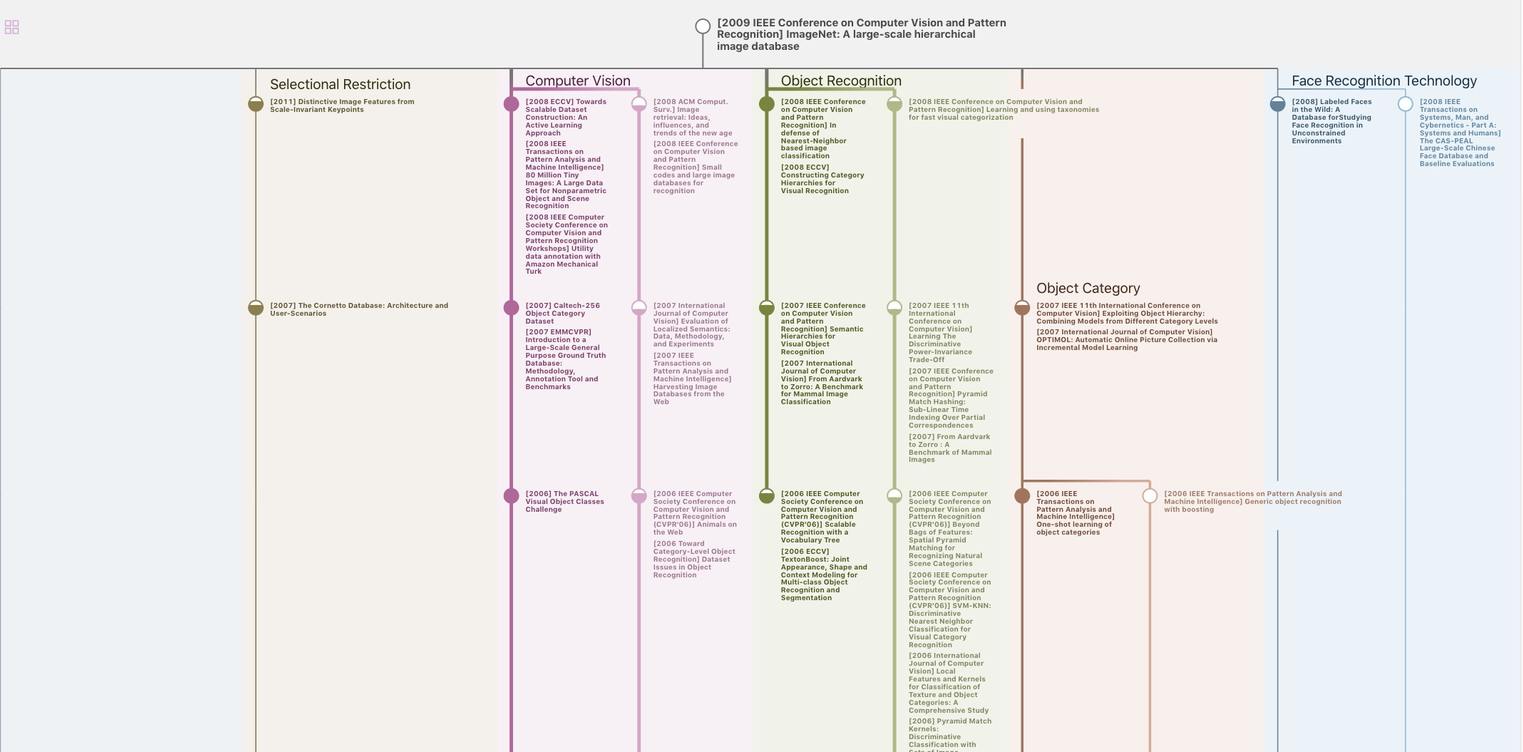
生成溯源树,研究论文发展脉络
Chat Paper
正在生成论文摘要