FL-GNNs: Robust Network Representation via Feature Learning Guided Graph Neural Networks
IEEE TRANSACTIONS ON NETWORK SCIENCE AND ENGINEERING(2024)
摘要
Graph Neural Networks (GNNs) have been widely developed and grown rapidly to address representation and learning for attribute graph data $G(A,X)$. However, existing studies on GNNs mainly focus on the message passing on graph $A$ for layer-wise propagation while pay less attention to the robust learning for the input features $X$, which thus make existing GNNs often perform susceptibility w.r.t feature noises and adversarial perturbations in $X$. In this article, we propose a novel Feature Learning guided Graph Neural Networks (FL-GNNs) by incorporating robust feature learning into GNNs. The core of FL-GNNs is trying to recover (or learn) a more clean and optimal feature data $Z$ from input features $X$ that better serves GNNs learning by jointly conducting feature reconstruction and GNNs' learning simultaneously. FL-GNNs is general and can be incorporated into any specific GNN models to enhance their robustness. An efficient algorithm has been derived to optimize FL-GNNs. Experimental results show that FL-GNNs can obviously enhance the robustness of existing GCN and GAT w.r.t feature noises and adversarial perturbations.
更多查看译文
关键词
Task analysis,Representation learning,Robustness,Graph neural networks,Convolution,Perturbation methods,Convolutional neural networks,feature learning,robust learning,graph attention networks
AI 理解论文
溯源树
样例
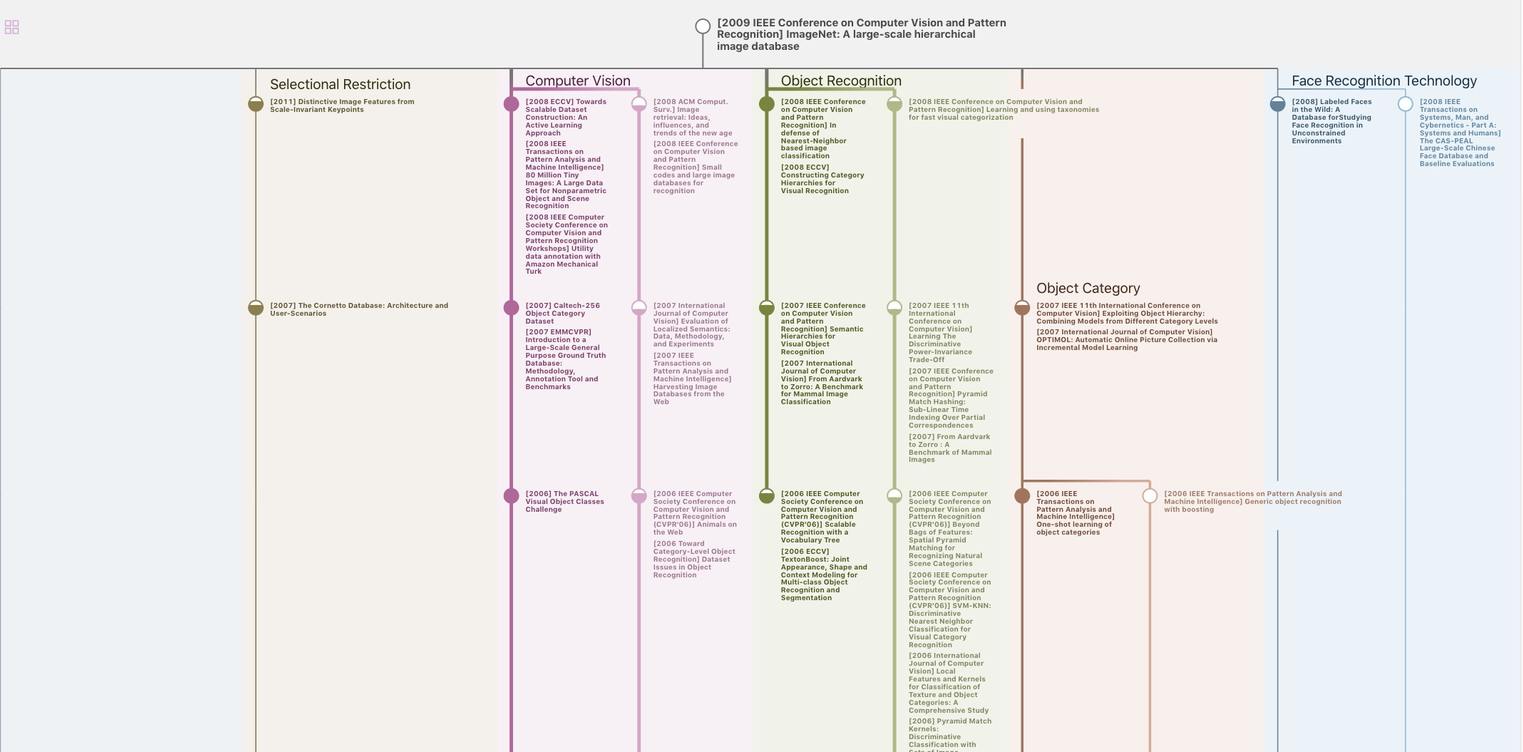
生成溯源树,研究论文发展脉络
Chat Paper
正在生成论文摘要