TL-P3GAN: an Efficient Temporal Learning-based Generative Adversarial Network for Precise P300 Signal Generation for P300 Spellers
IEEE TRANSACTIONS ON COGNITIVE AND DEVELOPMENTAL SYSTEMS(2024)
Abstract
Problem of data imbalance among target and non-target classes is inherent in the oddball paradigm-based P300 speller. This class imbalance is a critical issue and requires advanced rigorous learning to deal with. Conventionally, data level-like sampling approaches and algorithmic level-like ensemble approaches had been attempted in past research for data augmentation. However, information loss, overfitting and subject variability were their major pitfalls. Alternatively, generative adversarial network (GAN) based data augmentation managed to alleviate information loss but exhibits problems of overfitting and subject variability due to lack of temporal learning. To compensate for those problems, authors have proposed novel temporal learning-based GAN (TL-P3GAN) to generate precise P300 signals and augment the minority class i.e., P300. The TL-P3GAN comprises novel contribution of multiscale morphological learning in both generator and discriminator. Moreover, the multiscale hybrid model in generator learns multi-resolution morphological information and considers sample-wise latency information from original P300. The effectiveness of TL-P3GAN was confirmed by qualitative and quantitative evaluation metrics with two standard datasets. Further, the work is extended to analyze the effect of generated P300 signals on P300 classification and found significant performance improvement of 8-16% with both datasets in comparison with existing conventional and GAN-based data augmentation approaches.
MoreTranslated text
Key words
Generative Adversarial Network (GAN),P300 Speller,Temporal Learning,Brain-Computer Interface (BCI)
AI Read Science
Must-Reading Tree
Example
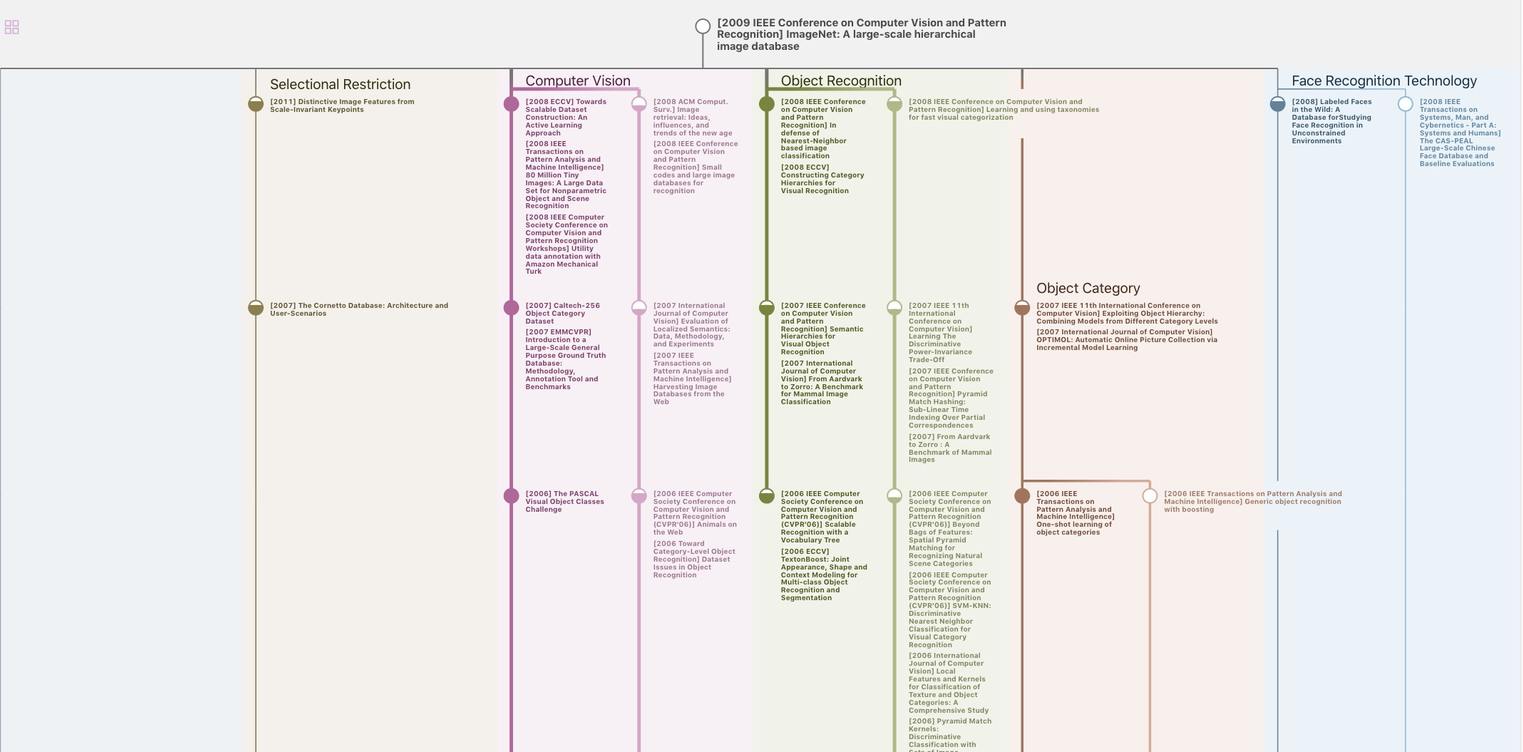
Generate MRT to find the research sequence of this paper
Chat Paper
Summary is being generated by the instructions you defined