Advanced machine learning approach to increase diagnostic accuracy in atypical Alzheimer’s disease cases
Alzheimer's & Dementia(2023)
摘要
Abstract Background The early diagnosis of Alzheimer’s disease (AD) and other types of dementia is essential in clinical practice. Up to 50% of patients with any form of dementia may remain undiagnosed during their lifetime and the diagnosis of AD may often be inaccurate. In assessing patients, accurate diagnosis based on only clinical data and structural (MRI or CT) imaging is suboptimal in a subset of patients with atypical presentation. Although amyloid PET imaging and CSF biomarkers can provide incremental benefits, numerous obstacles preclude their wider use in clinical practice. In this work, a parametric study was performed to create a machine learning (ML) model to accurately diagnose hard‐to‐detect cases of AD, to mitigate the need for more expensive or invasive testing such as amyloid imaging or spinal tap. Method Data from 173 participants (MCI and mild dementia), including 105 patients (60%) with AD and 68 (40%) Non‐AD patients, were included from our memory clinic. The dataset for each participant included pertinent history, AD risk factors, scores on a neuropsychological testing battery, functional status, and MRI volumetric studies. Participants were divided into two groups: Group I (easy‐to‐detect) with a high probability of AD diagnosis based on clinical data (subjective and objective amnestic presentation) and volumetric studies (hippocampal and medial temporal lobe atrophy). Group II (hard‐to‐detect), who did not fit into the first group and the diagnosis was confirmed with either amyloid imaging or CSF analysis. The distribution of diagnosis by group: 1‐ Easy to detect (43 AD, 29 Non‐AD),2‐ Hard to detect (62 AD, 39 Non‐AD), a total of three experiments with different combinations of groups were conducted, 1‐ All Groups (Exp.1.), 2‐ Hard to detect (Exp.2.), 3‐ Easy to detect (Exp.3.). In all experiments, Leave‐One‐Out cross‐validation technique was performed. Result A total number of 132 features utilized, by employing feature selection approach, 13 features were selected. Our model attains the highest accuracy of 87.27% on classification of Exp.1., 83.28% on Exp.2. and 95.80% on Exp.3. Conclusion We investigated discrimination of AD from Non‐AD on hard‐to‐detect cases using ML. The proposed method obtains competitive performance and achieves improved accuracy results.
更多查看译文
关键词
atypical alzheimers,diagnostic accuracy,machine learning,advanced machine
AI 理解论文
溯源树
样例
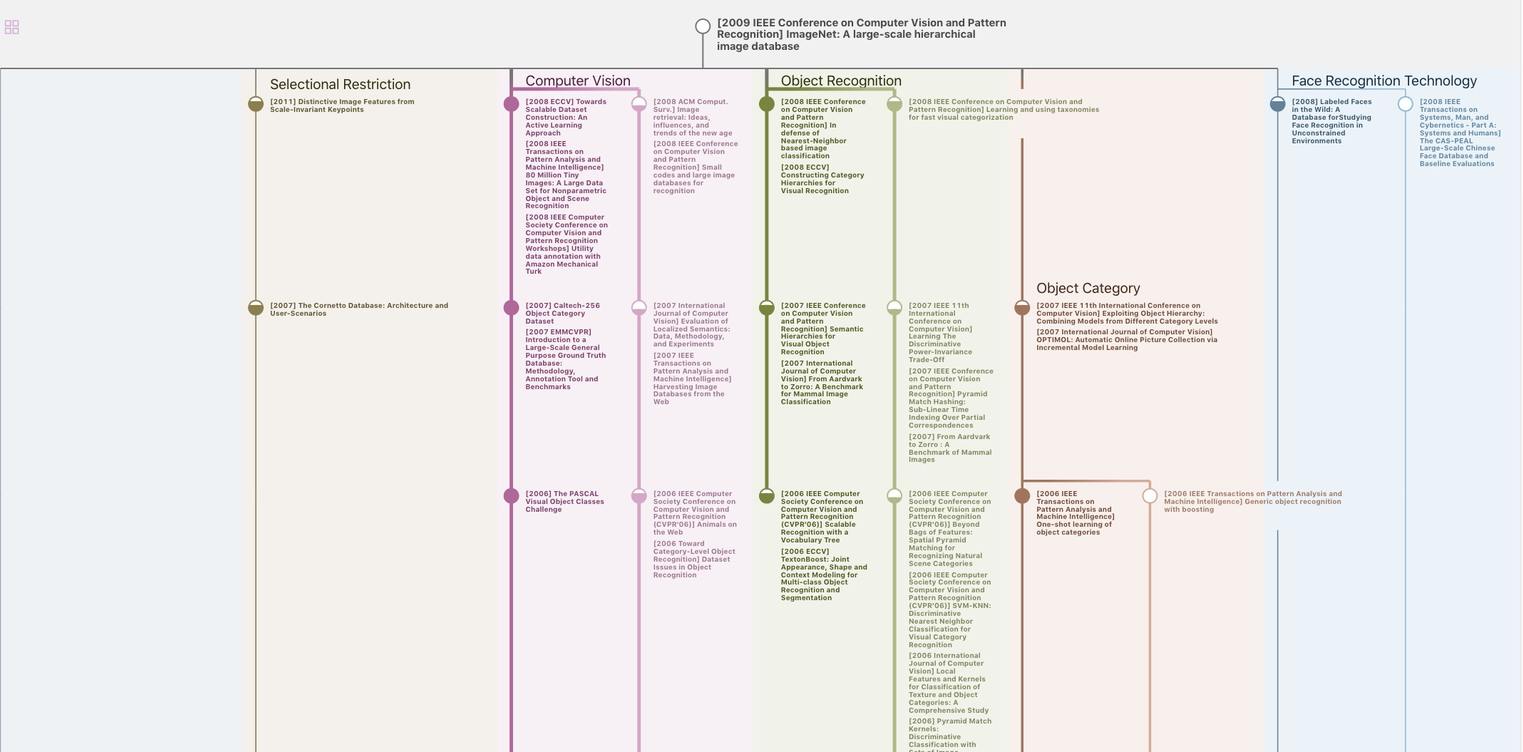
生成溯源树,研究论文发展脉络
Chat Paper
正在生成论文摘要