Enhancing Sample Efficiency in Black-box Combinatorial Optimization via Symmetric Replay Training
arXiv (Cornell University)(2023)
摘要
Black-box combinatorial optimization (black-box CO) is frequently encountered in various industrial fields, such as drug discovery or hardware design. Despite its widespread relevance, solving black-box CO problems is highly challenging due to the vast combinatorial solution space and resource-intensive nature of black-box function evaluations. These inherent complexities induce significant constraints on the efficacy of existing deep reinforcement learning (DRL) methods when applied to practical problem settings. For efficient exploration with the limited availability of function evaluations, this paper introduces a new generic method to enhance sample efficiency. We propose symmetric replay training that leverages the high-reward samples and their under-explored regions in the symmetric space. In replay training, the policy is trained to imitate the symmetric trajectories of these high-rewarded samples. The proposed method is beneficial for the exploration of highly rewarded regions without the necessity for additional online interactions - free. The experimental results show that our method consistently improves the sample efficiency of various DRL methods on real-world tasks, including molecular optimization and hardware design.
更多查看译文
关键词
optimization,sample efficiency,black-box black-box
AI 理解论文
溯源树
样例
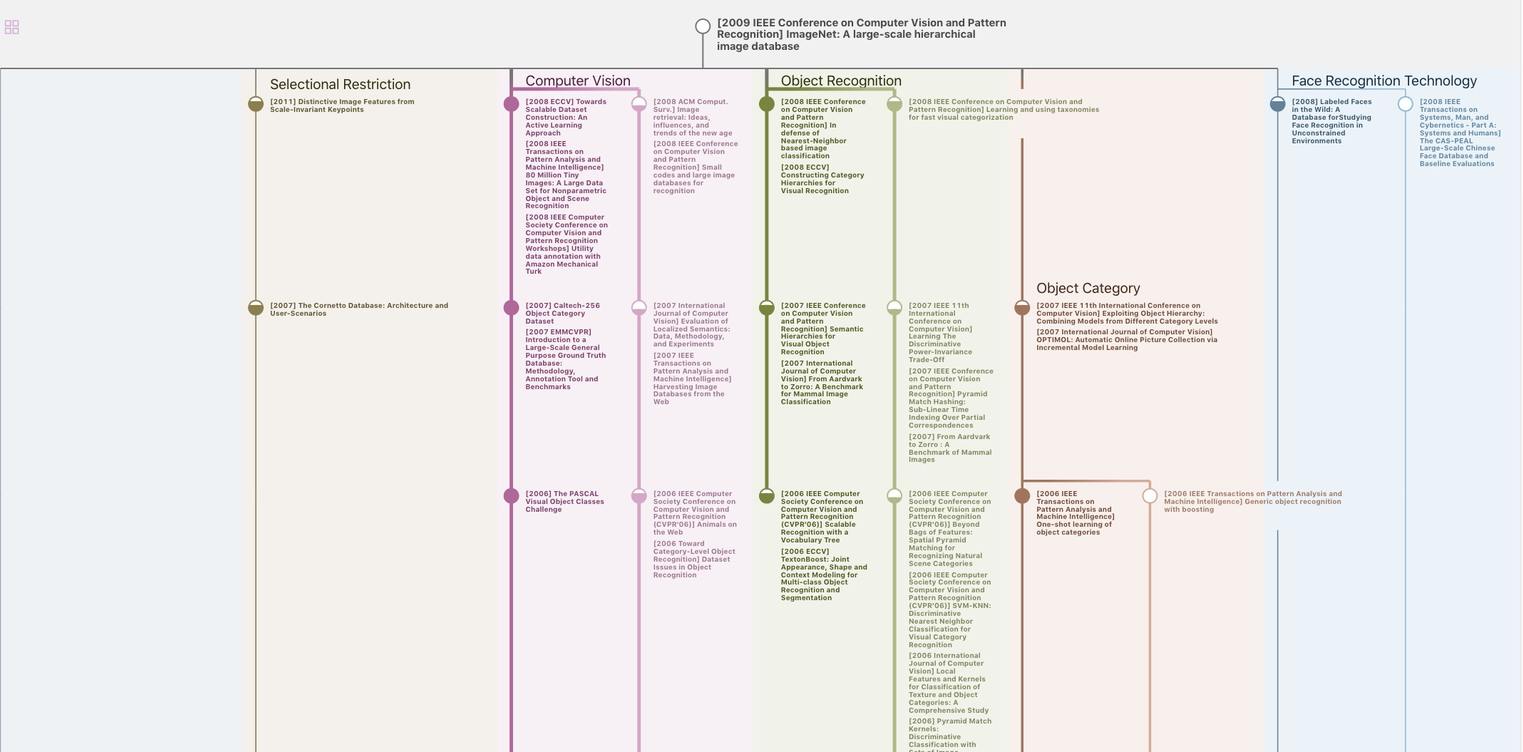
生成溯源树,研究论文发展脉络
Chat Paper
正在生成论文摘要