Advancing thermoelectric materials discovery through semi-supervised learning and high-throughput calculations
APPLIED PHYSICS LETTERS(2023)
摘要
The data-driven machine learning technique is widely used to assist in accelerating the design of thermoelectric materials. In this study, we proposed a positive and unlabeled learning (PU learning) method, a semi-supervised learning, to train a classifier to distinguish the positive samples from the unlabeled samples, in which the positive class was labeled by matching the formulas in our dataset with the published article titles. The probabilities that the unlabeled materials belong to the positive class were predicted by PU learning, and 40 candidate thermoelectric materials were determined. The transport properties were calculated by high-throughput first-principles calculations, among which 8 p-type and 12 n-type materials have the maximum theoretical zT values greater than 1. Specifically, a series of AX(2) binary compounds, (Cd/Zn)(GaTe2)(2) ternary compounds, and Cs(Dy/Ho/Tb)(2)Ag3Te5 quaternary compounds deserve further investigations in the future.
更多查看译文
关键词
thermoelectric materials,discovery,learning,semi-supervised,high-throughput
AI 理解论文
溯源树
样例
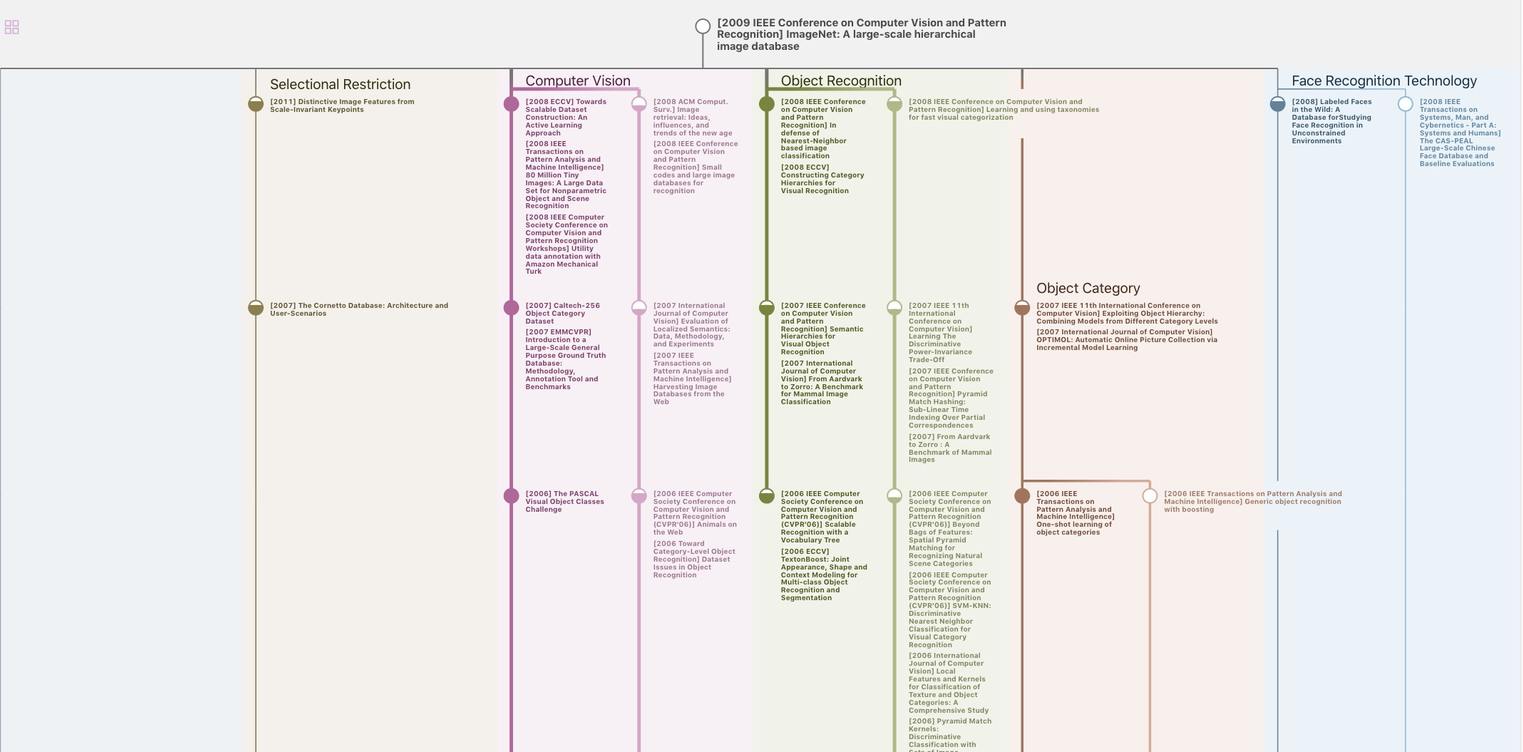
生成溯源树,研究论文发展脉络
Chat Paper
正在生成论文摘要