State Augmentation via Self-Supervision in Offline Multi-Agent Reinforcement Learning
IEEE Transactions on Cognitive and Developmental Systems(2023)
Abstract
The utilization of pre-collected offline datasets for learning in the absence of environmental interaction has enabled reinforcement learning (RL) to make significant strides in real-world circumstances. This approach is also attractive for multi-agent reinforcement learning (MARL) tasks, given the complex interactions that occur between agents and the environment. However, when compared to the single-agent approach, offline multi-agent RL faces more challenges due to the larger state and action space, particularly with regard to poor out-of-distribution generalization to the environment. The present study demonstrates the ineffectiveness of directly transferring conservative offline RL algorithms from single-agent settings to multi-agent environments, which is due to the accumulating extrapolation errors that increase in proportion to the number of agents. In this paper, we explore the efficacy of three types of data augmentation techniques that can be applied to the state representation in the context of MARL. By combining the proposed data augmentation techniques with a state-of-the-art offline multi-agent algorithm, we improve the function-approximation of centralized Q-networks. The experimental results conducted on StarCraft II strongly support the effectiveness of the data augmentation techniques in enhancing the performance of offline multi-agent reinforcement learning in the state space.
MoreTranslated text
Key words
Offline Reinforcement Learning,Multi-Agent Reinforcement Learning,Data Augmentation,Self-Supervision
AI Read Science
Must-Reading Tree
Example
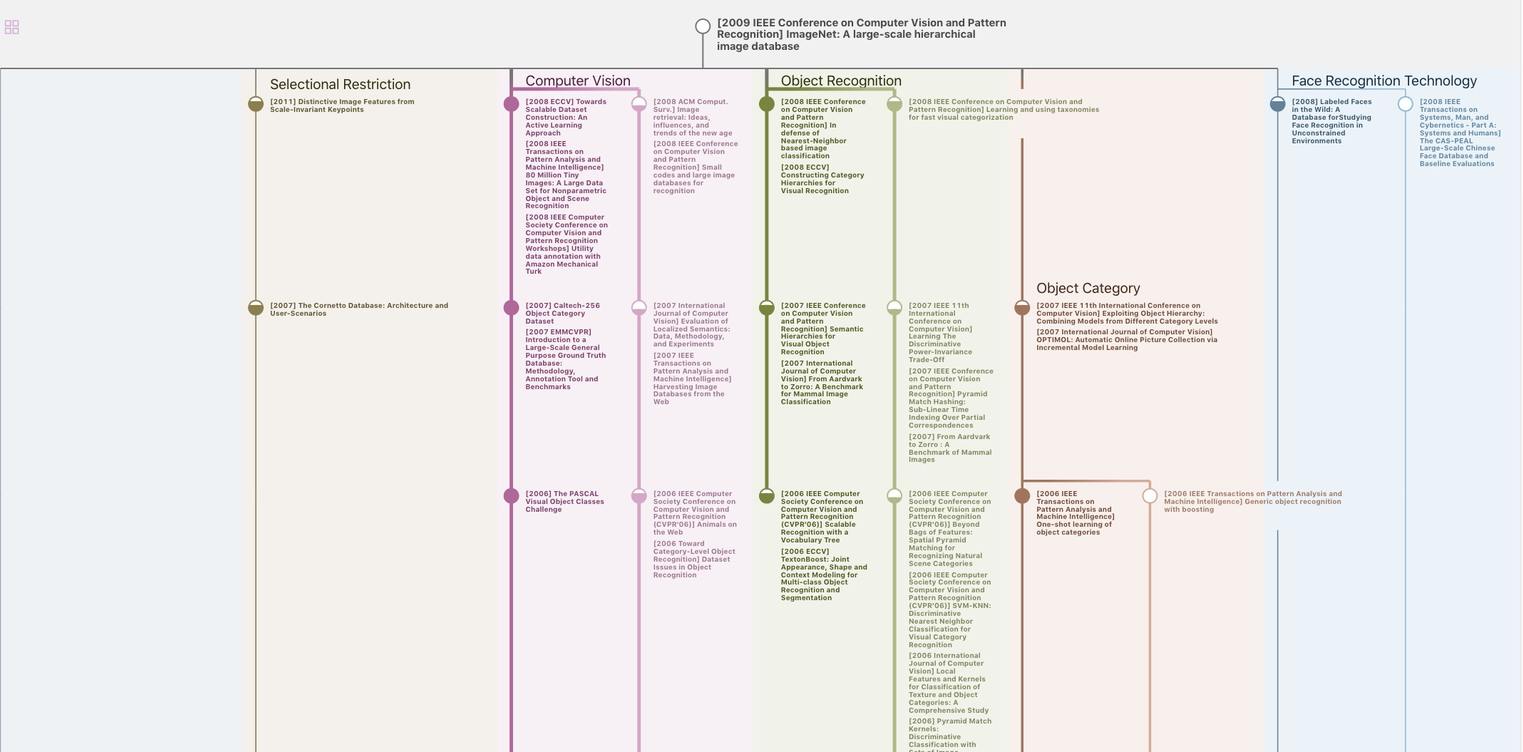
Generate MRT to find the research sequence of this paper
Chat Paper
Summary is being generated by the instructions you defined