Infer and Adapt: Bipedal Locomotion Reward Learning from Demonstrations via Inverse Reinforcement Learning
arXiv (Cornell University)(2023)
摘要
Enabling bipedal walking robots to learn how to maneuver over highly uneven, dynamically changing terrains is challenging due to the complexity of robot dynamics and interacted environments. Recent advancements in learning from demonstrations have shown promising results for robot learning in complex environments. While imitation learning of expert policies has been well-explored, the study of learning expert reward functions is largely under-explored in legged locomotion. This paper brings state-of-the-art Inverse Reinforcement Learning (IRL) techniques to solving bipedal locomotion problems over complex terrains. We propose algorithms for learning expert reward functions, and we subsequently analyze the learned functions. Through nonlinear function approximation, we uncover meaningful insights into the expert's locomotion strategies. Furthermore, we empirically demonstrate that training a bipedal locomotion policy with the inferred reward functions enhances its walking performance on unseen terrains, highlighting the adaptability offered by reward learning.
更多查看译文
关键词
bipedal locomotion reward learning,demonstrations
AI 理解论文
溯源树
样例
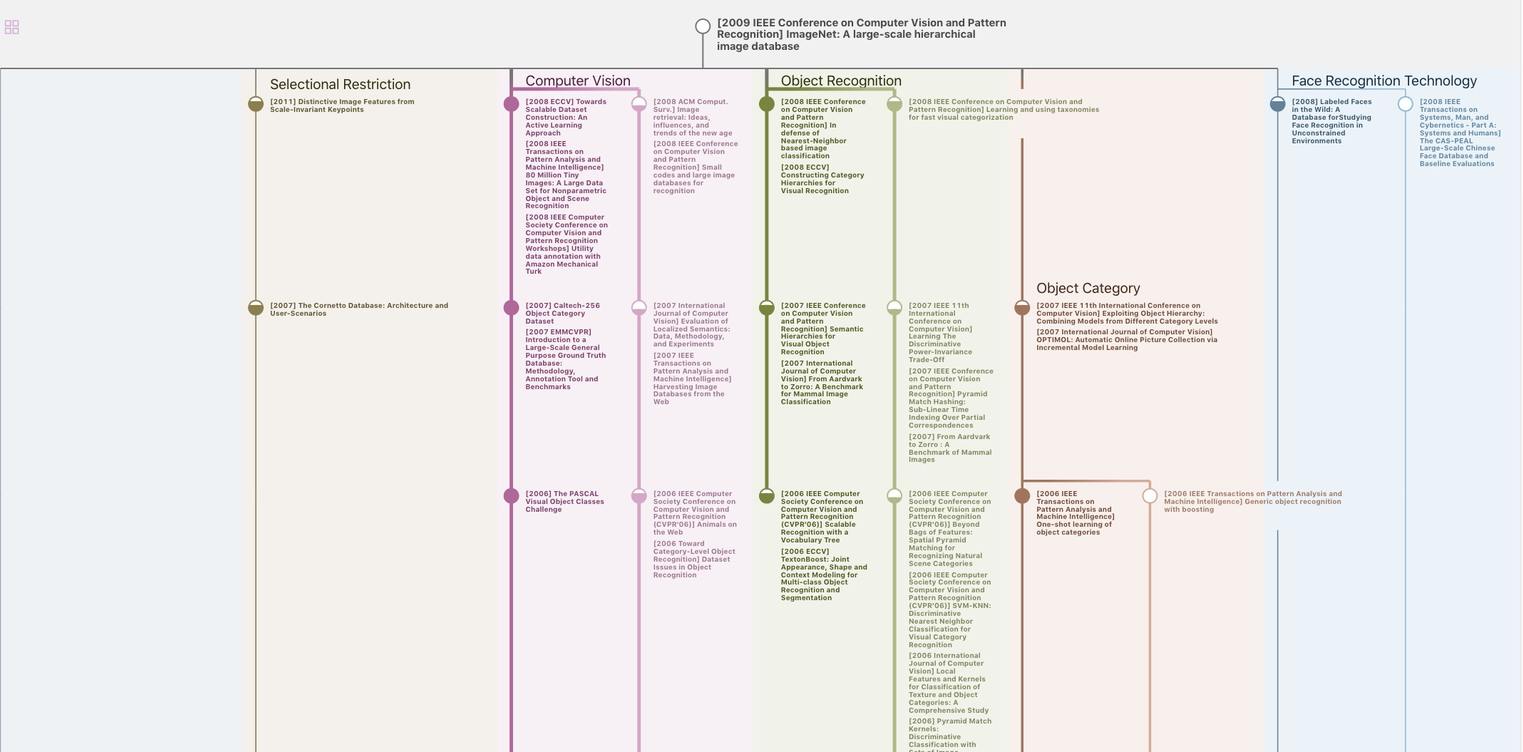
生成溯源树,研究论文发展脉络
Chat Paper
正在生成论文摘要