Kernel Ridge Regression Inference
arXiv (Cornell University)(2023)
摘要
We provide uniform inference and confidence bands for kernel ridge regression (KRR), a widely-used non-parametric regression estimator for general data types including rankings, images, and graphs. Despite the prevalence of these data -- e.g., ranked preference lists in school assignment -- the inferential theory of KRR is not fully known, limiting its role in economics and other scientific domains. We construct sharp, uniform confidence sets for KRR, which shrink at nearly the minimax rate, for general regressors. To conduct inference, we develop an efficient bootstrap procedure that uses symmetrization to cancel bias and limit computational overhead. To justify the procedure, we derive finite-sample, uniform Gaussian and bootstrap couplings for partial sums in a reproducing kernel Hilbert space (RKHS). These imply strong approximation for empirical processes indexed by the RKHS unit ball with logarithmic dependence on the covering number. Simulations verify coverage. We use our procedure to construct a novel test for match effects in school assignment, an important question in education economics with consequences for school choice reforms.
更多查看译文
关键词
kernel ridge regression inference
AI 理解论文
溯源树
样例
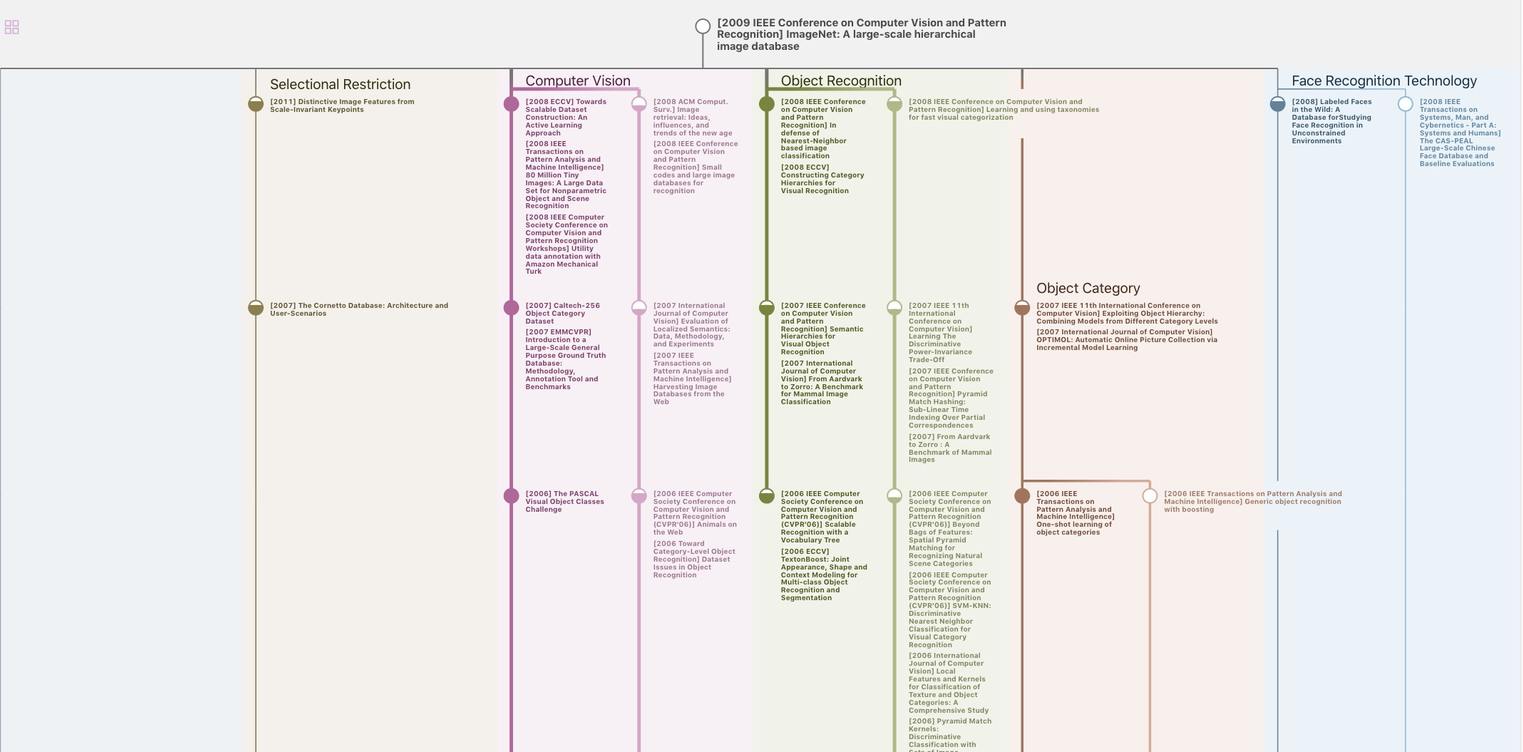
生成溯源树,研究论文发展脉络
Chat Paper
正在生成论文摘要