Abstract 12554: Machine Learning-Based Detection of Intraoperative Ischemia Utilizing the VitalDB Database
Circulation(2023)
Abstract
Introduction: Myocardial ischemia, characterized by insufficient blood supply to the heart, is a critical condition often associated with adverse cardiac events, including myocardial infarction. Detecting intraoperative ischemia holds substantial clinical significance. This study aims to develop a machine-learning (ML) model to detect intraoperative hypotension as a marker of ischemia using electrocardiogram (ECG) features. Hypothesis: We hypothesize that machine-learning algorithms can detect changes in ECG episodes during ischemic or subischemic events associated with prolonged hypotension. Methods: The study utilized the VitalDB database, a comprehensive repository of intraoperative data of 6,388 patients, including ECG recordings and other vital signs. The ML model was a binary classifier of a hypotensive event (MBP <65 mm Hg) or a non-hypotensive event (MBP >75 mm Hg) by analyzing ECG-II and ECG-V5 waveforms. We extracted relevant ECG features such as ST-deviation (ST-elevation or ST-depression), as clinical indicators of myocardial ischemia. The adaptive boosting (AdaBoost), Gradient boosting (GB), and Extreme gradient boosting (XGB) methods, ML boosting techniques, were employed to develop a hypotension predictive model for detecting ischemic episodes. The primary outcomes were the accuracy and the area under the receiver operating characteristic (AUROC) curve. Additionally, we utilized the Shapley Additive Explanations (SHAP) to determine the contribution of the ECG features to the ML model. Results: The GB machine learning model exhibited the best results (Accuracy: 89%, AUROC: 0.92) in predicting hypotensive events. Furthermore, the model achieved high sensitivity and specificity in identifying ischemic episodes. SHAP analysis revealed that ST-deviation is the most significant contributing ECG feature in predicting intraoperative hypotension. Conclusions: These results demonstrate the potential utility of ML-based identification of ECG features in accurately identifying ischemic episodes. Implementation of an improved diagnostic methodology for intraoperative ischemia detection can have a significant impact on patient care, enhancing patient safety and improving surgical outcomes.
MoreTranslated text
Key words
Cardiovascular Evaluation
AI Read Science
Must-Reading Tree
Example
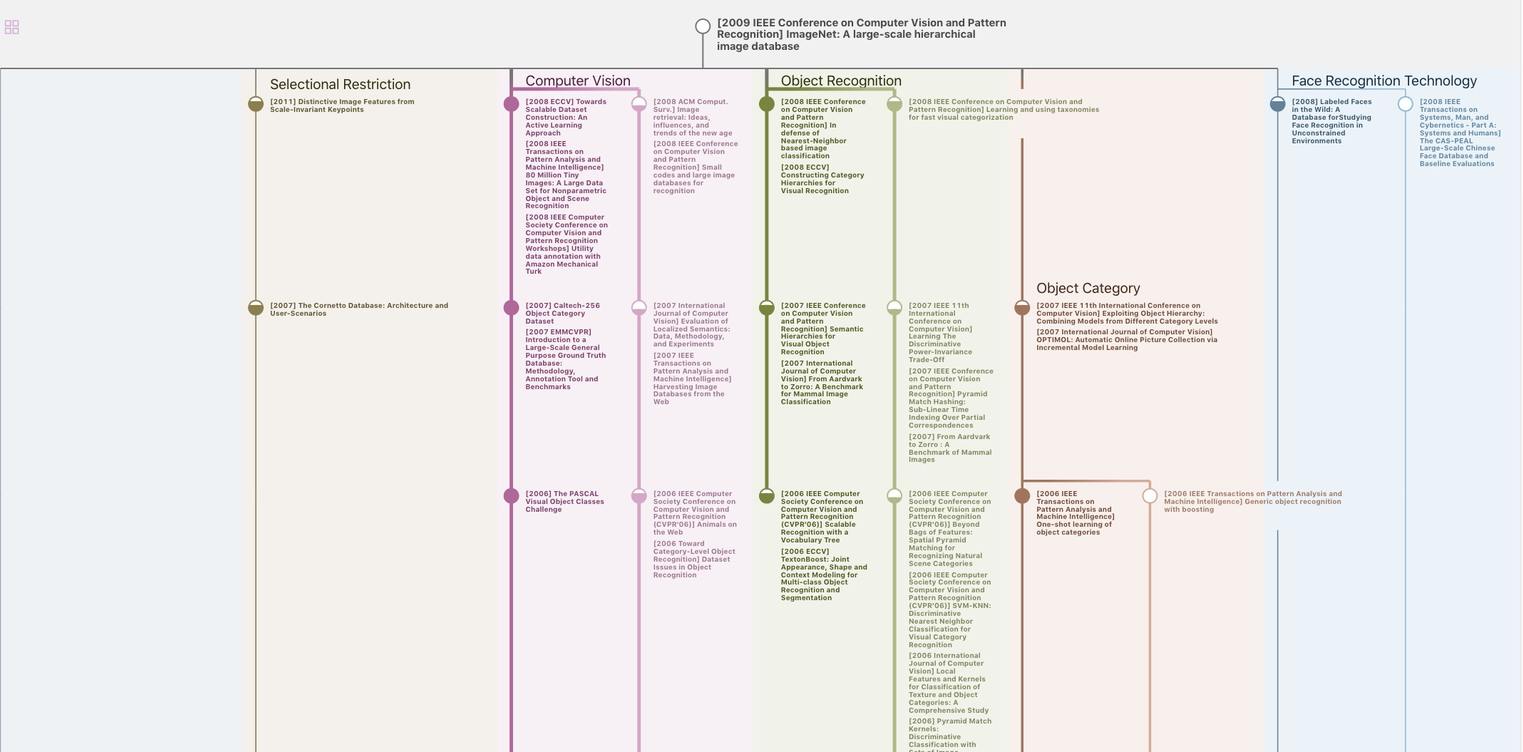
Generate MRT to find the research sequence of this paper
Chat Paper
Summary is being generated by the instructions you defined