SCLert: A Span-Based Joint Model for Measurable Quantitative Information Extraction from Chinese Texts
IEEE transactions on consumer electronics(2023)
摘要
Measurable quantitative information is a fundamental set of information related to the quantity of objects, which widely exists in various texts including medical records, national standards and technical reports. In recent years, some researchers have recognized the importance of measurable quantitative information in helping to analyze healthcare and have proposed pipeline-based approaches, but there are still many challenging issues with measurable information extraction. This paper proposes a span-based joint model (SCLert) for measurable quantitative information extraction, which incorporates Chinese generic features and lexicon information for improving the effectiveness of measurable quantitative information recognition. Compared to pipeline extraction methods that require two independent models, our joint extraction model combines both measurable quantitative information recognition and association within one model. The span-based representation enhances the boundary information of entity and enables more accurate extraction of measurable quantitative information. We evaluated the model on a Standard Quantitative Information dataset with 3106 Chinese text sentences and a Clinical Quantitative Information dataset with 1359 Chinese electronic medical records. Experiment results show that our joint model outperforms baseline models by an improvement of 3.67% on recognition and 6.61% on association in F1-score on the standard datasets.
更多查看译文
关键词
Measurable quantitative information,joint model,span,information extraction
AI 理解论文
溯源树
样例
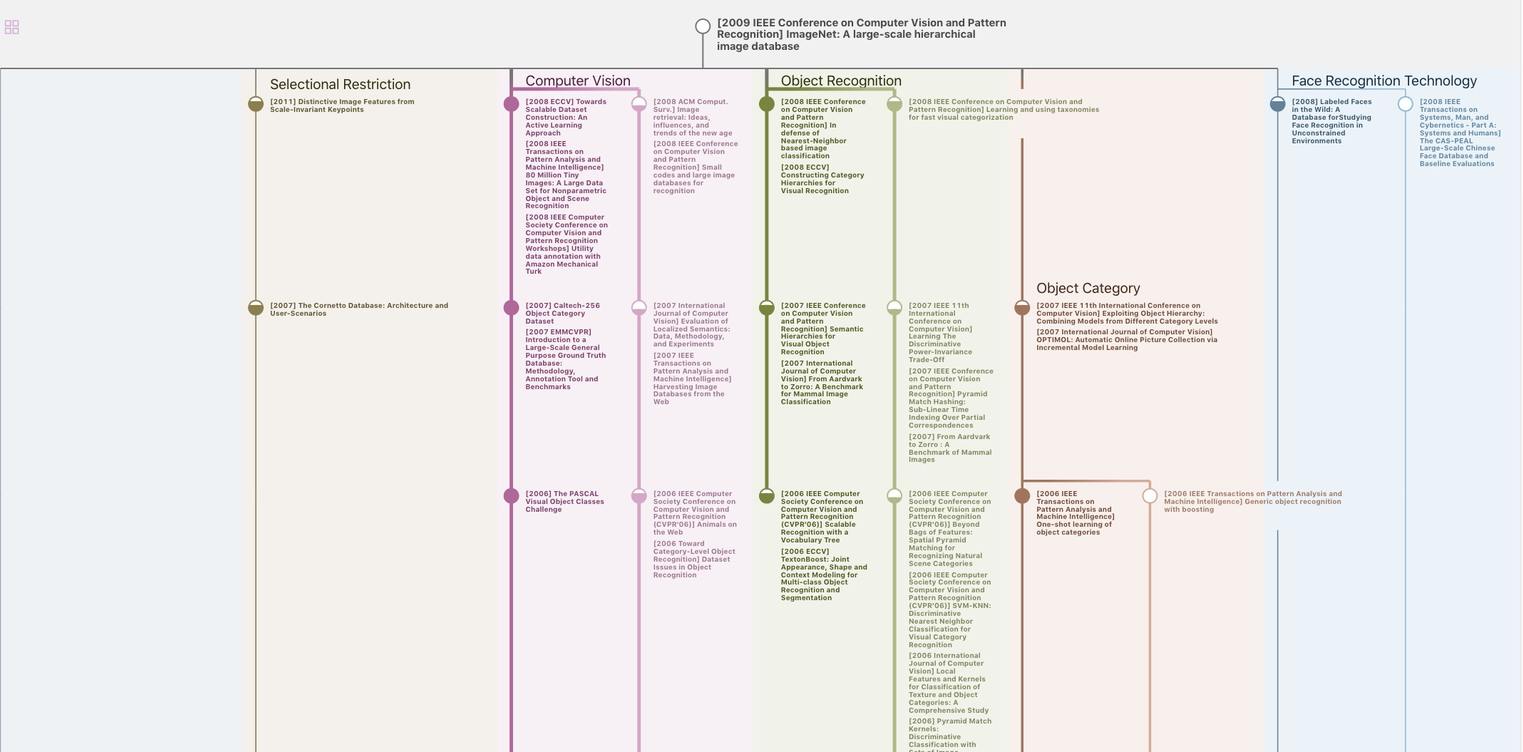
生成溯源树,研究论文发展脉络
Chat Paper
正在生成论文摘要