Deep Learning-Based Approaches Using Feature Selection Methods for Automatic Diagnosis of COVID-19 Disease from X-Ray Images
Studies in big data(2023)
摘要
The COVID-19 pandemic caused by the SARS-CoV-2 virus, which arose in Wuhan, Hubei province, China, on 31 December 2019 and rapidly spread to six continents and hundreds of countries, will go down in history as the first pandemic produced by coronaviruses. The World Health Organization (WHO) classified COVID-19 as a “pandemic” on March 11, 2020. To avoid the future spread of this pandemic and to promptly treat infected patients, it is crucial to find positive cases as early as feasible. To prevent or slow the spread of COVID-19 infection, an accurate early diagnosis is crucial. Medical imaging techniques, such as X-rays and computed tomography (CT), play a crucial role in the global fight against COVID-19. In this paper, preprocessing techniques have been applied to X-RAY images to improve classification performance. Gradient operator used as Sobel operator to highlight dot regions in X-RAY images and reduce the number of house gray tones The application of artificial intelligence and machine learning techniques to radiological images helps to detect this disease accurately and quickly. In this study, 13 different deep learning techniques were studied using Chi-square, NCA, mRMR and ReliefF feature selection algorithms. A detailed experimental study was carried out including AlexNet, EfficientNet B0, GoogleNet, Inception ResNet-v2, Inception-v3, DenseNet201, MobilevNet2, Nasnet-Large, ResNet18, ResNet50, ResNet101, VGG16 and VGG19 deep learning models and artificial neural networks, nearest neighbor algorithm and traditional machines such as support vector machine, decision tree and neural network including learning methods. Classifiers built using pre-trained models were trained and the accuracy rates of the resulting models for the test data was calculated as 96.42%, 96.61%, 96.51%, 96.06%, 95.00%, 96.14%, 96.14%, 96.32%, 96.04%, 97.08%, 96.04%, 96.42%, 95.66% and 98.21% for models based on AlexNet, DenseNet EfficientNet B0, GoogleNet, Inception ResNet-v2, Inception-v3, MobilevNet2, Nasnet-Large, ResNet18, ResNet50, ResNet101, VGG16, VGG19, and ResNet50 + AlexNet Cubic SVM with mRMR feature selections. In experimental studies, the highest accuracy value was obtained in Cubic SVM as 98.21% by applying mRMR feature selections to the features obtained from ResNet50 and AlexNet models. These results show that classifiers created using pre-trained models can assist physicians in the diagnosis of the COVID-19 pandemic.
更多查看译文
关键词
feature selection methods,automatic diagnosis,learning-based,x-ray
AI 理解论文
溯源树
样例
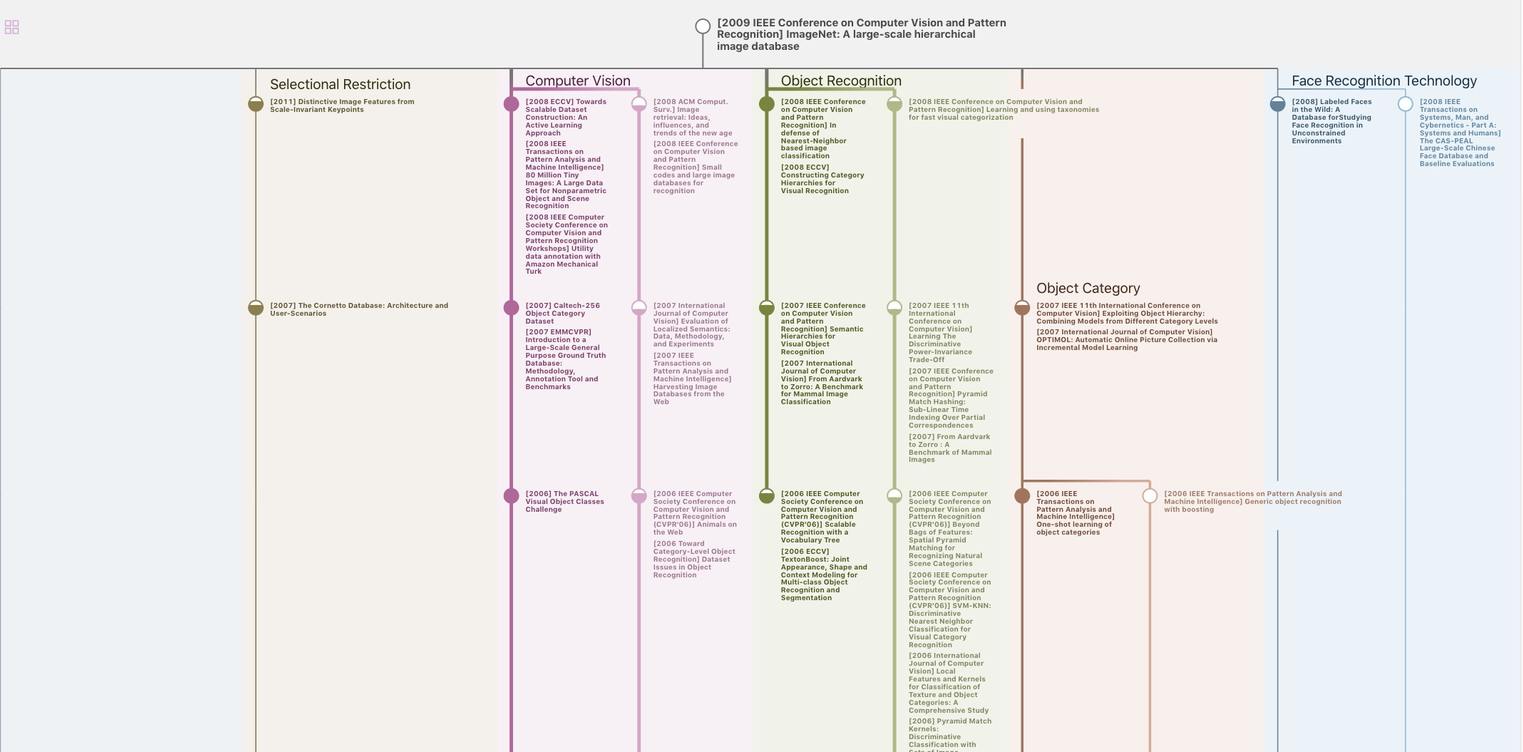
生成溯源树,研究论文发展脉络
Chat Paper
正在生成论文摘要