Tire-Road Friction Estimation for Four-Wheel Independent Steering and Driving EVs Using Improved CKF and FNN
IEEE transactions on transportation electrification(2024)
摘要
Estimation of the tire-road friction coefficient (TRFC) has always been a hotspot in the field of vehicle control. This problem is also significant in electric vehicles (EV) with four-wheel independent steering and driving (4WISD). In this paper, a novel scheme based on an improved cubature Kalman filter (CKF) and a fuzzy neural network (FNN) is proposed for estimating the TRFC. Firstly, a prior knowledge based CKF (PCKF) is designed to observe forces acting on tires. PCKF can perform good tracking under rapidly changing states by using prior knowledge. Simulations show that PCKF is more accurate than CKF and extended Kalman filters (EKF). Then, a self-organizing asymmetric FNN (SOAFNN) is designed to fit the characteristics of tires instead of tire models. An asymmetric membership function (AMF) is used to reduce the number of parameters, and the self-organizing method is used to get the best size of a network. The TRFC value can be estimated by combining PCKF and SOAFNN. Apart from a common condition, we also study two more conditions unique to 4WISD EVs, which were simply ignored in past studies. The experimental results show that the proposed scheme has higher accuracy than those of the baselines under the three conditions. The root-mean-square error (RMSE) values of PCKF and SOAFNN are reduced by 21.80% and 19.71% than those of CKF and recursive least squares (RLS), respectively.
更多查看译文
关键词
Tires,Wheels,Estimation,Fuzzy neural networks,Vehicle dynamics,Torque,Sensors,Cubature Kalman filter (CKF),electric vehicle (EV),four-wheel independent steering and driving (4WISD),self-organizing asymmetric fuzzy neural network (SOAFNN),tire-road friction coefficient (TRFC)
AI 理解论文
溯源树
样例
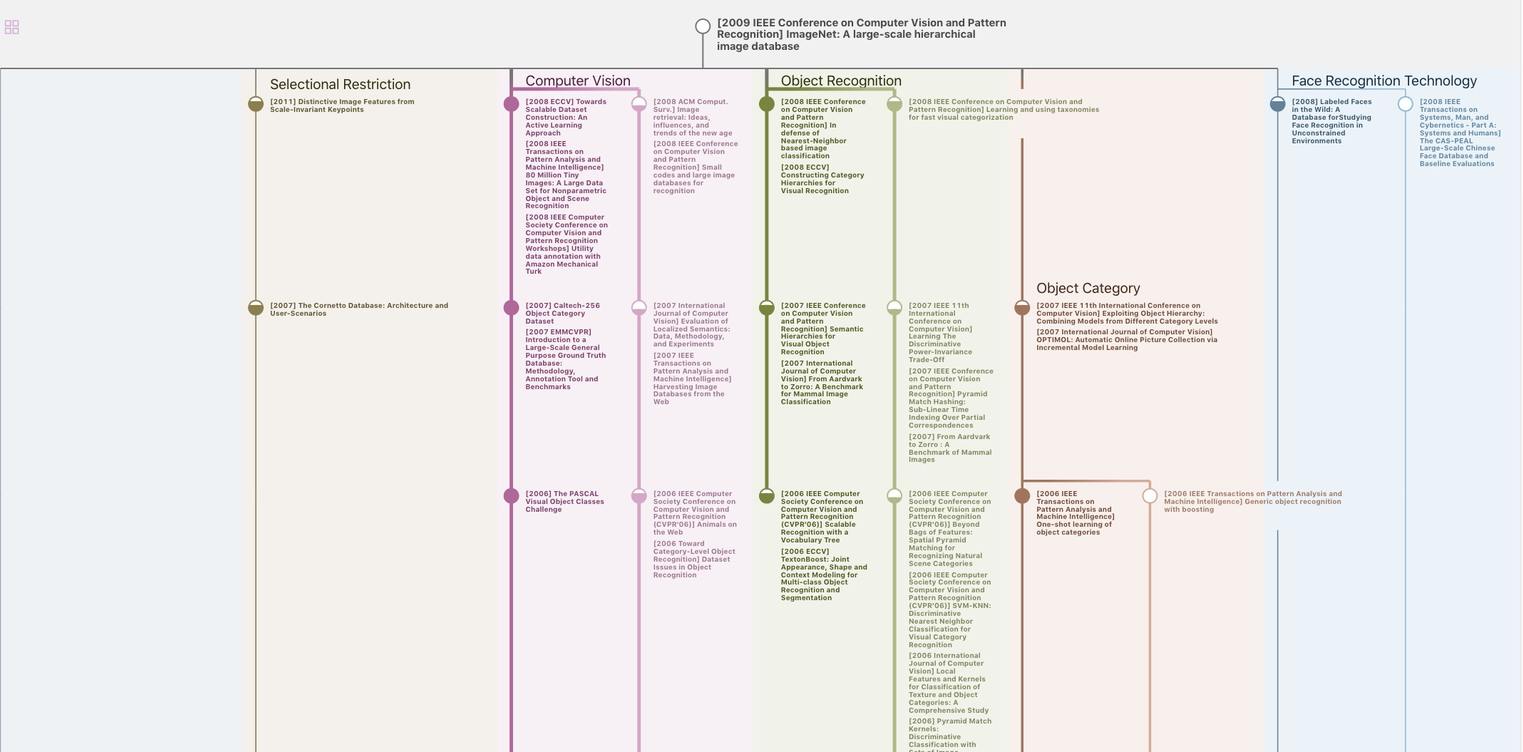
生成溯源树,研究论文发展脉络
Chat Paper
正在生成论文摘要