Using Deep Learning Models to Examine the Biological Impact of Polygenic Risks for Alzheimer’s Disease
Alzheimer's & Dementia(2023)
摘要
AbstractBackgroundAlzheimer’s disease (AD) is a leading cause of mortality in the elderly. Genetics studies suggest that AD is polygenic in nature; thus, an individual’s risk of AD can be predicted by polygenic risk score (PRS) models. Of these models, the deep learning models more accurately capture nonlinear interactions among different genetic risks and are more suitable for modeling disease polygenic risk.MethodWe used the neural network‐based deep learning models to evaluate the polygenic risk of AD. Meanwhile, we examined the associations of resultant PRSs and plasma protein biomarkers for AD by regression analysis. We also identified the biological pathways that contribute to the polygenic risk using Gene Set Enrichment Analysis.ResultWe found that the resultant PRSs of AD are associated with the levels of specific AD plasma protein biomarkers, including amyloid beta and p‐tau181, as well as changes in the activity of specific biological events, such as TNF‐α and cytokine‐related pathways.ConclusionOur findings suggest that AD polygenic risk is associated with AD progression and modulates immune‐associated pathways. They also highlight the potential use of deep learning methods in investigating the underlying genetic mechanisms of AD.
更多查看译文
AI 理解论文
溯源树
样例
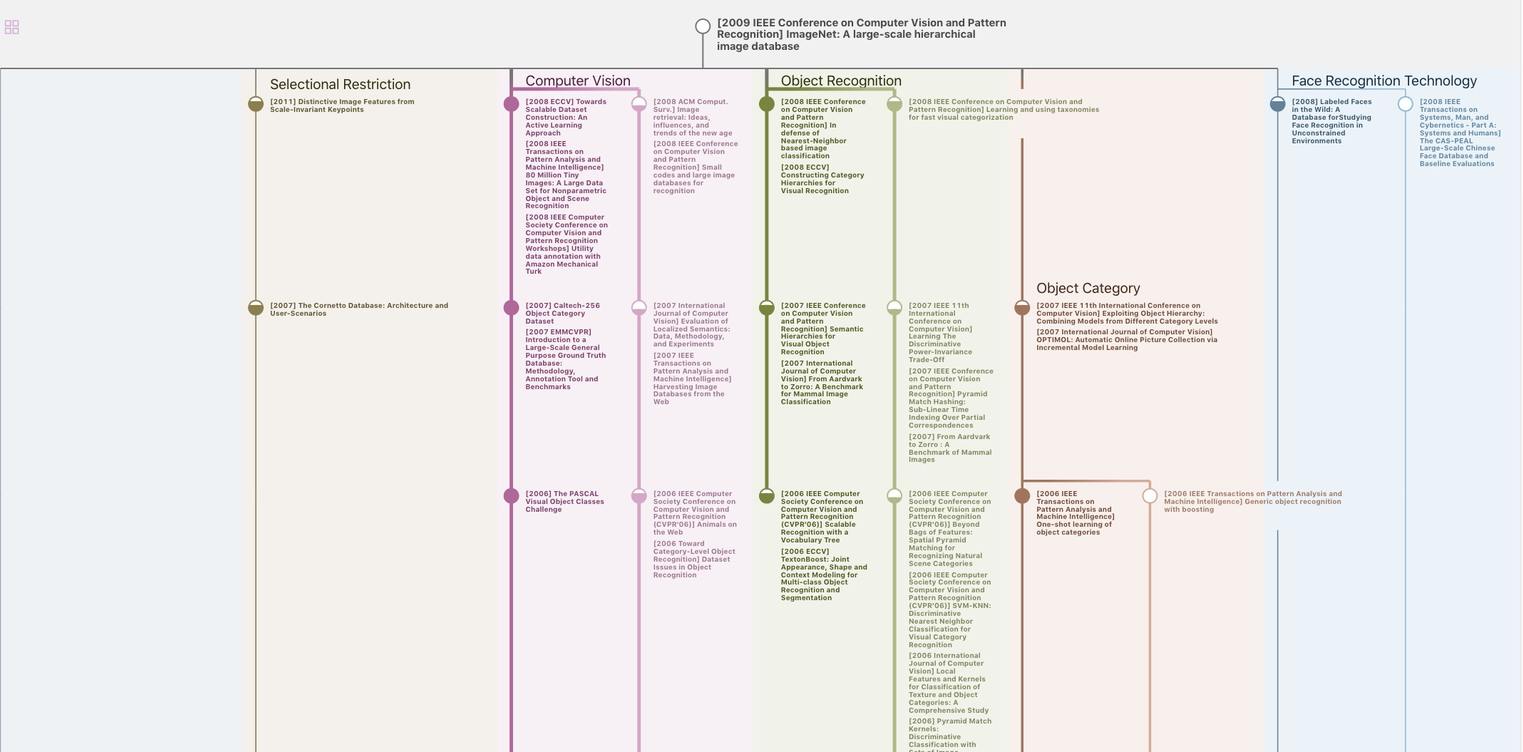
生成溯源树,研究论文发展脉络
Chat Paper
正在生成论文摘要