Total effects with constrained features
Statistics and Computing(2024)
摘要
Recent studies have emphasized the connection between machine learning feature importance measures and total order sensitivity indices (total effects, henceforth). Feature correlations and the need to avoid unrestricted permutations make the estimation of these indices challenging. Additionally, there is no established theory or approach for non-Cartesian domains. We propose four alternative strategies for computing total effects that account for both dependent and constrained features. Our first approach involves a generalized winding stairs design combined with the Knothe-Rosenblatt transformation. This approach, while applicable to a wide family of input dependencies, becomes impractical when inputs are physically constrained. Our second approach is a U-statistic that combines the Jansen estimator with a weighting factor. The U-statistic framework allows the derivation of a central limit theorem for this estimator. However, this design is computationally intensive. Then, our third approach uses derangements to significantly reduce computational burden. We prove consistency and central limit theorems for these estimators as well. Our fourth approach is based on a nearest-neighbour intuition and it further reduces computational burden. We test these estimators through a series of increasingly complex computational experiments with features constrained on compact and connected domains (circle, simplex), non-compact and non-connected domains (Sierpinski gaskets), we provide comparisons with machine learning approaches and conclude with an application to a realistic simulator.
更多查看译文
关键词
Feature importance,Constrained features,Winding stairs,U-statistics
AI 理解论文
溯源树
样例
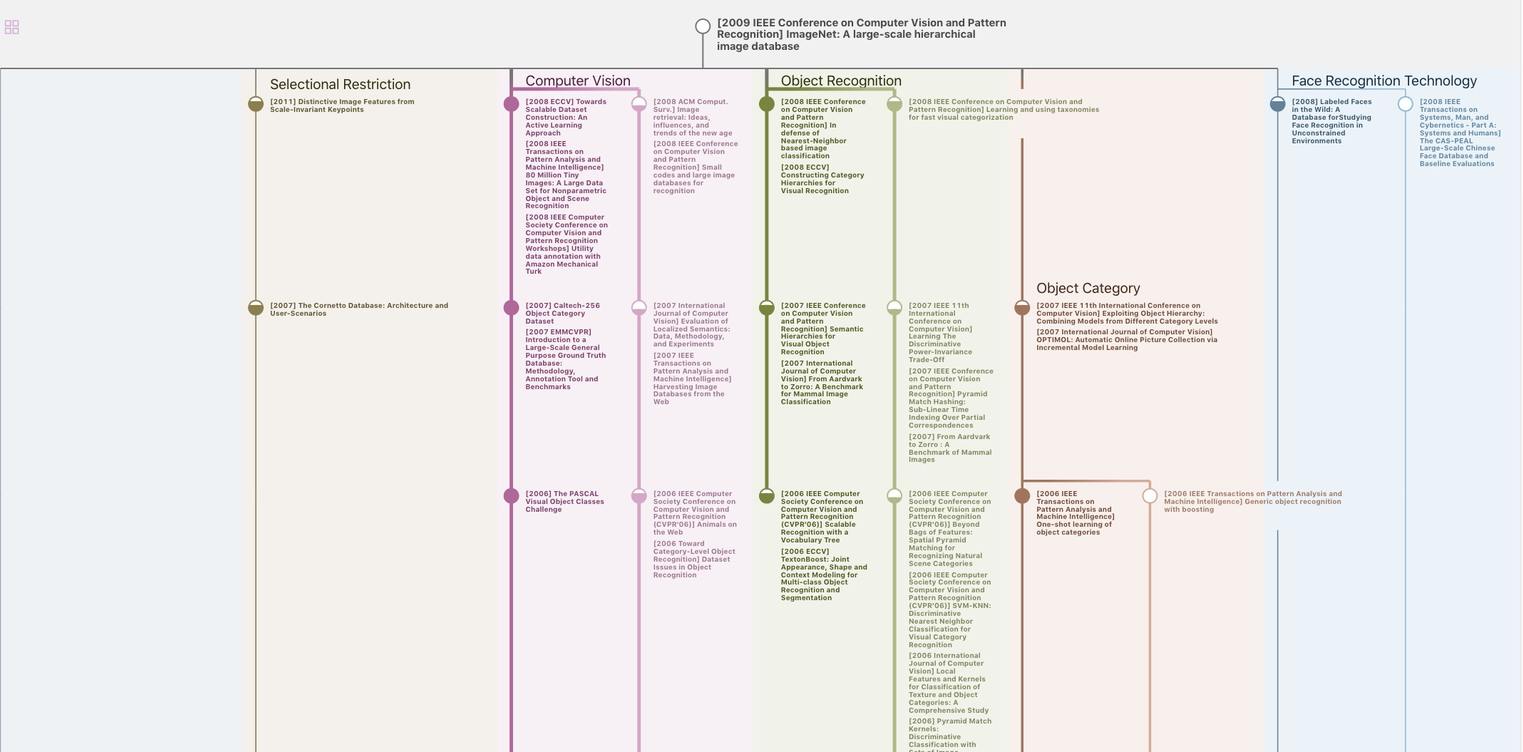
生成溯源树,研究论文发展脉络
Chat Paper
正在生成论文摘要