Modelling of Compression Ignition Engine by Soft Computing Techniques (ANFIS-NSGA-II and RSM) to Enhance the Performance Characteristics for Leachate Blends with Nano-additives
Research Square (Research Square)(2023)
摘要
Abstract The focus of this study is on the potential of using oils extracted from food waste that ended up in landfills. These waste oils were tested to see how they affected performance and emissions in diesel engines. The study's results are analysed and compared with models created using intelligent hybrid prediction approaches including adaptive neuro-fuzzy inference system (ANFIS), response surface methodology (RSM) - genetic algorithm (GA), and ANFIS - non sorting genetic algorithm (NSGA-II). The analysis takes into account engine load, blend percentage, nanoadditive concentration, and injection pressure, and the desired responses are the thermal efficiency and specific energy consumption of the brakes, as well as the concentrations of carbon monoxide, unburned hydrocarbon, and oxides of nitrogen. Root-mean-square error (RMSE) and the coefficient of determination (R2) were used to assess the predictive power of the model (R2). Comparitevely to AI and the RSM-GA model, the results provided by ANFIS-NSGA-II are superior. This is because it achieved a pareto optimum front of 24.45 kW, 2.76, 159.54 ppm, 4.68 ppm, and 0.020243% for BTE, BSEC, NOX, UBHC, and CO. Combining the precision of ANFIS's prediction with the efficiency of NSGA-optimization II's gives a reliable and thorough evaluation of the engine's settings.
更多查看译文
关键词
compression ignition engine,leachate blends,soft computing techniques,anfis-nsga-ii,nano-additives
AI 理解论文
溯源树
样例
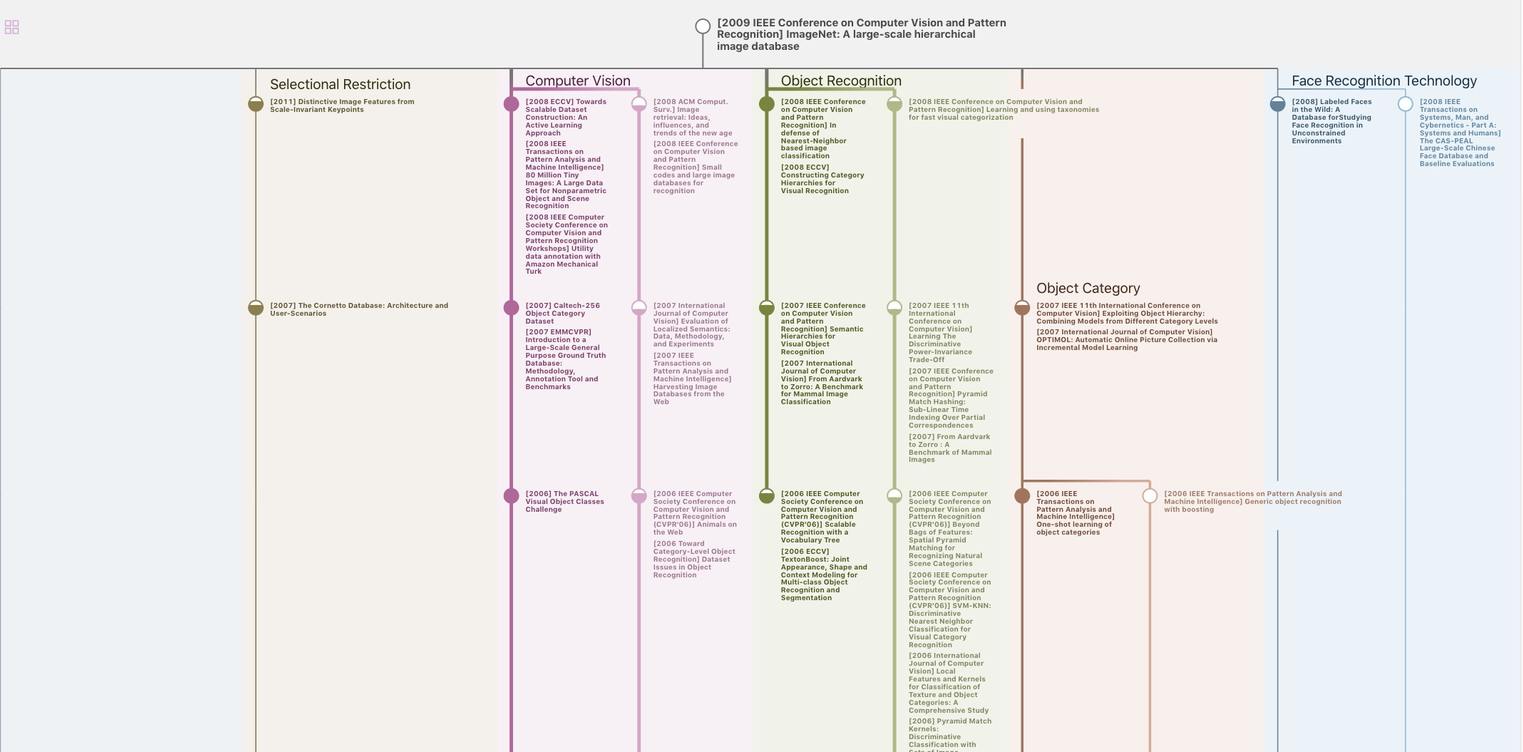
生成溯源树,研究论文发展脉络
Chat Paper
正在生成论文摘要