Triplet-Branch Network with Contrastive Prior-Knowledge Embedding for Disease Grading
ARTIFICIAL INTELLIGENCE IN MEDICINE(2024)
摘要
Since different disease grades require different treatments from physicians, i.e., the low-grade patients may recover with follow-up observations whereas the high-grade may need immediate surgery, the accuracy of disease grading is pivotal in clinical practice. In this paper, we propose a Triplet-Branch Network with ContRastive priOr-knoWledge embeddiNg (TBN-CROWN) for the accurate disease grading, which enables physicians to accordingly take appropriate treatments. Specifically, our TBN-CROWN has three branches, which are implemented for representation learning, classifier learning and grade-related prior-knowledge learning, respectively. The former two branches deal with the issue of class-imbalanced training samples, while the latter one embeds the grade-related prior-knowledge via a novel auxiliary module, termed contrastive embedding module. The proposed auxiliary module takes the features embedded by different branches as input, and accordingly constructs positive and negative embeddings for the model to deploy grade-related prior-knowledge via contrastive learning. Extensive experiments on our private and two publicly available disease grading datasets show that our TBN-CROWN can effectively tackle the class-imbalance problem and yield a satisfactory grading accuracy for various diseases, such as fatigue fracture, ulcerative colitis, and diabetic retinopathy.
更多查看译文
关键词
Disease grading,Class imbalance,Fatigue fracture,Ulcerative colitis,Diabetic retinopathy
AI 理解论文
溯源树
样例
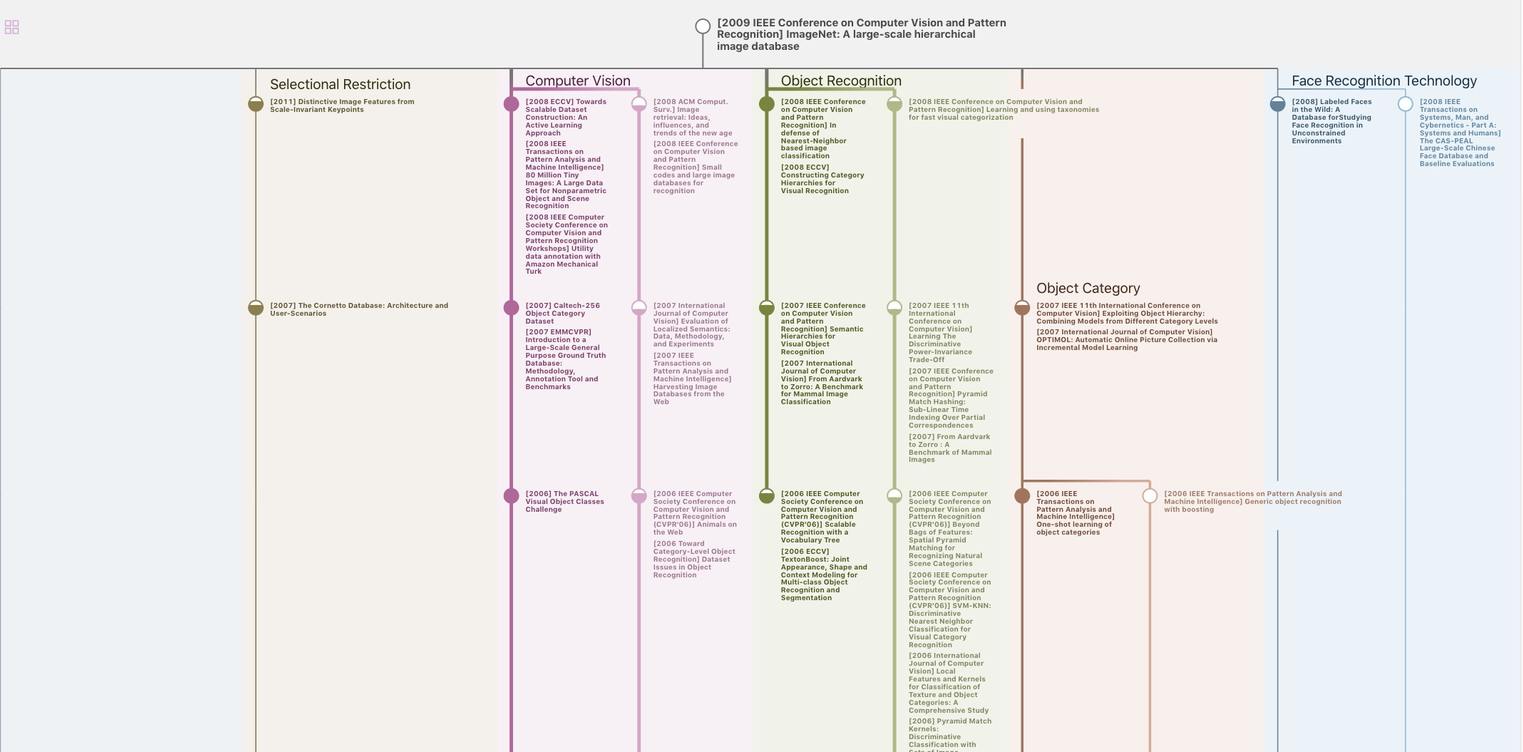
生成溯源树,研究论文发展脉络
Chat Paper
正在生成论文摘要