Efficient Open Domain Multi-Hop Question Answering with Few-Shot Data Synthesis
arXiv (Cornell University)(2023)
摘要
Few-shot learning for open domain multi-hop question answering typically relies on large language models (LLMs). While powerful, LLMs are inefficient at the inference time. We propose a data synthesis framework for multi-hop question answering that allows for improving smaller language models with less than 10 human-annotated question answer pairs. The framework is built upon the data generation functions parameterized by LLMs and prompts, which requires minimal hand-crafted features. Empirically, we synthesize millions of multi-hop questions and claims. After finetuning language models on the synthetic data, we evaluate the models on popular benchmarks on multi-hop question answering and fact verification. Our experimental results show that finetuning on the synthetic data improves model performance significantly, allowing our finetuned models to be competitive with prior models while being almost one-third the size in terms of parameter counts.
更多查看译文
关键词
multi-hop,few-shot
AI 理解论文
溯源树
样例
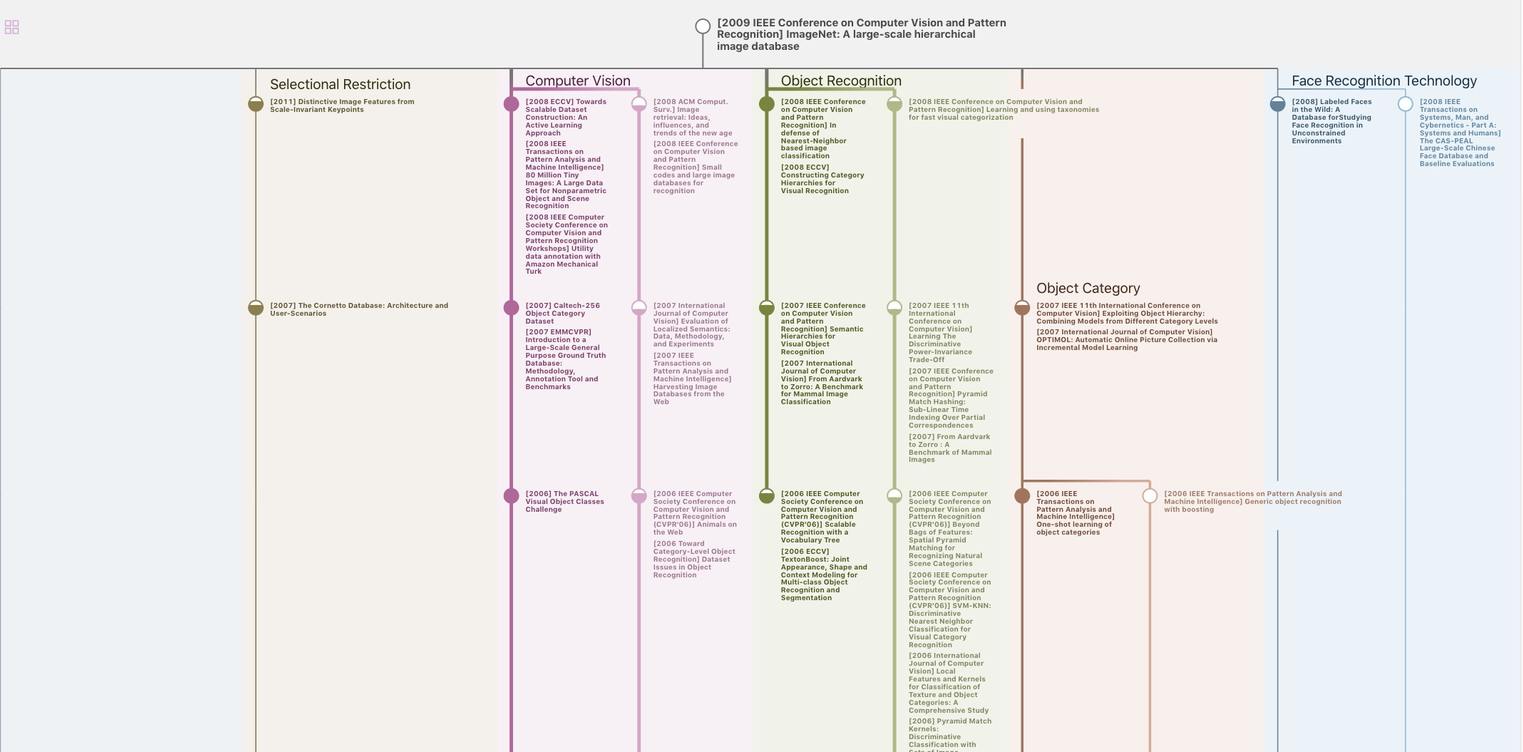
生成溯源树,研究论文发展脉络
Chat Paper
正在生成论文摘要