Abstract P1179: Evaluation of A Machine Learning-based Metabolic Marker for Coronary Artery Disease in the UK Biobank
Circulation research(2023)
摘要
A recent study has shown that an in silico quantitative score of CAD (ISCAD) can be built using machine learning and clinical data from electronic health records. ISCAD was shown to be associated with CAD risk, atherosclerosis, sequela and all-cause mortality. We developed a CAD-predictive learning model using only metabolic data from 93,642 individuals from the UK Biobank (median [IQR] age, 57 [14] years; 39,796 [42%] male; 5640 [6%] with diagnosed CAD), and assessed its probabilities as a quantitative metabolic risk score for CAD (M-CAD; range 0 [lowest probability] to 1 [highest probability]) in participants of the UK Biobank. The relationship of M-CAD with arterial stiffness index, ejection fraction, CAD sequelae, and key mortality measures was assessed. The model predicted CAD with an area under the receiver-operating-characteristic curve of 0.712 in the testing set. Arterial Stiffness Index increased by 0.19 and 0.27 meters/second and ejection fraction decreased by 0.2% and 1% per 0.1 increase in M-CAD in all subjects and CAD cases, respectively. Both incident and recurrent myocardial infarction increased stepwise over M-CAD quartiles when evaluated in all participants (odds ratio (OR) 15.3 [4.2%] and 12.5 [0.2%]) and participants with CAD (OR 1.78 [34%] and 2.32 [1.7%] in top quartiles as compared to the first quartile of incident and recurrent MI respectively). Likewise, the hazard ratio and prevalence of all-cause mortality, CVD-associated mortality, and CAD-associated mortality increased stepwise over M-CAD deciles when assessed in all participants (2.98 [14%], 9.34 [4.3%], 26.7 [2.7%] in the top deciles as compared to the first decile of all-cause, CVD, and CAD mortality respectively) and a subset of participants with CAD (2.32 [24%], 2.78 [14%]], 3.41 [10%] in the top deciles as compared to the first decile of all-cause, CVD, and CAD mortality respectively. Interpretation of the model with SHAP values demonstrated that features known to be CAD risk factors contributed to the model predictions in the direction expected with their documented biological effects. We demonstrate that metabolic-based machine learning can be used to build a quantitative risk score for CAD that is associated with subclinical atherosclerosis, CAD sequala and mortality.
更多查看译文
AI 理解论文
溯源树
样例
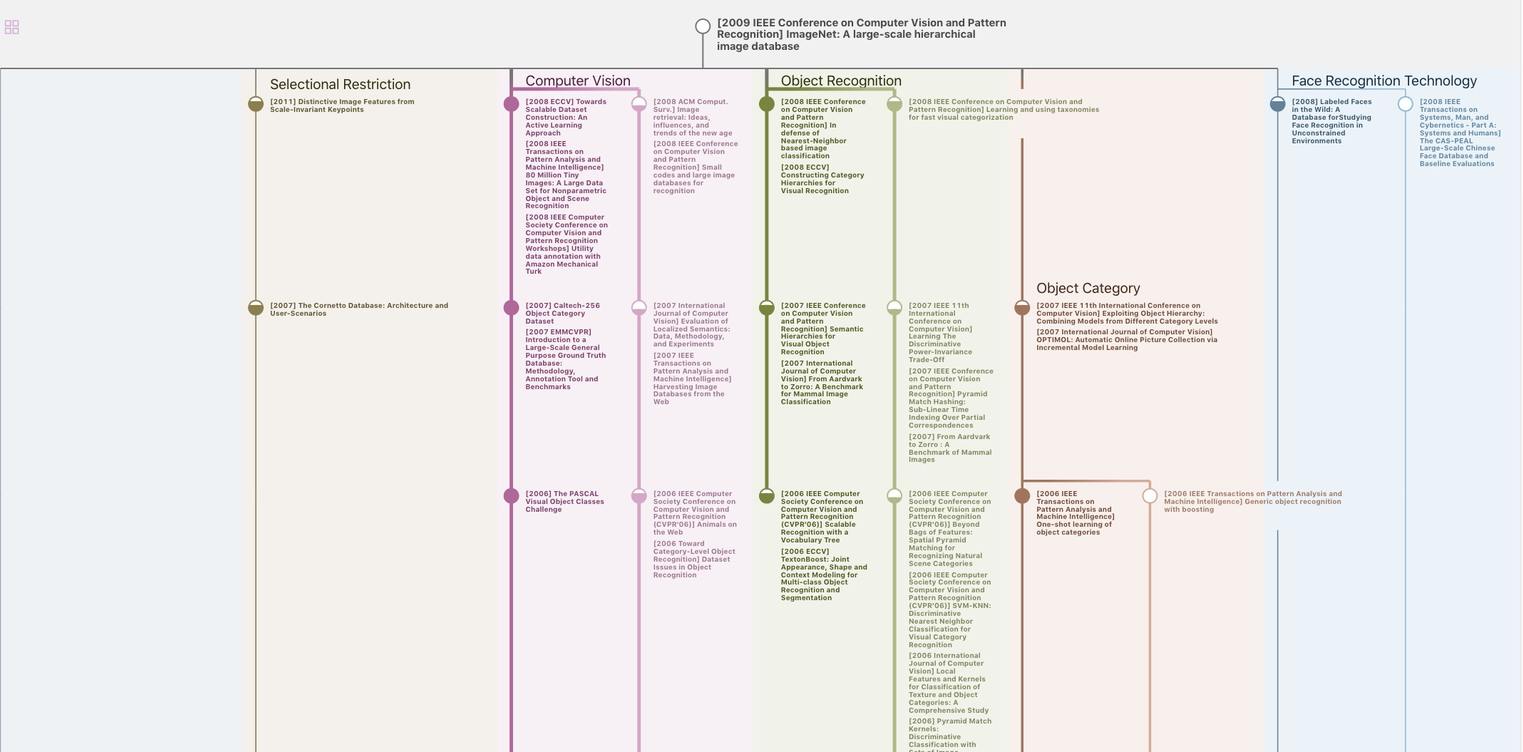
生成溯源树,研究论文发展脉络
Chat Paper
正在生成论文摘要