Application of Mutual Information Maximization Convolutional Neural Network in Bearing Feature Extraction
IEEE sensors journal(2023)
摘要
Deep learning models can automatically capture the state in a device from complex data. However, it is typically used for end-to-end fault diagnosis, and the visualization results of the convolutional layer are abstract. A convolutional neural network model with a maximization of mutual information (MMI) between inputs and outputs is proposed. It is the basic idea to enhance the feature extraction capability of the model through improved loss deconstraints and improve the interpretability of the model at the visualization level. First, the bearing vibration data are converted to an envelope spectrum signal, which is used as an input to the model. This process can be viewed as the model conducting feature extraction on the patterns of distinct characteristic spectral lines. Then, the multilayer convolution used for feature extraction is treated as a system; and the output of the system is designed to be the same size as the input of the model, so that the model is easy to understand; second, an attention mechanism (AM) is added to enhance the obvious features. Finally, a loss function is designed to drive the convolutional system based on the mutual information (MI) rule between the signal before and after noise reduction to extract more frequency components of the fault features. The simulated signals and real cases show that the proposed method has achieved certain effect in the fault feature extraction of bearings and also has this certain interpretability in the level of feature visualization.
更多查看译文
关键词
Convolutional neural networks (CNNs),fault feature extraction,mutual information,rolling bearing
AI 理解论文
溯源树
样例
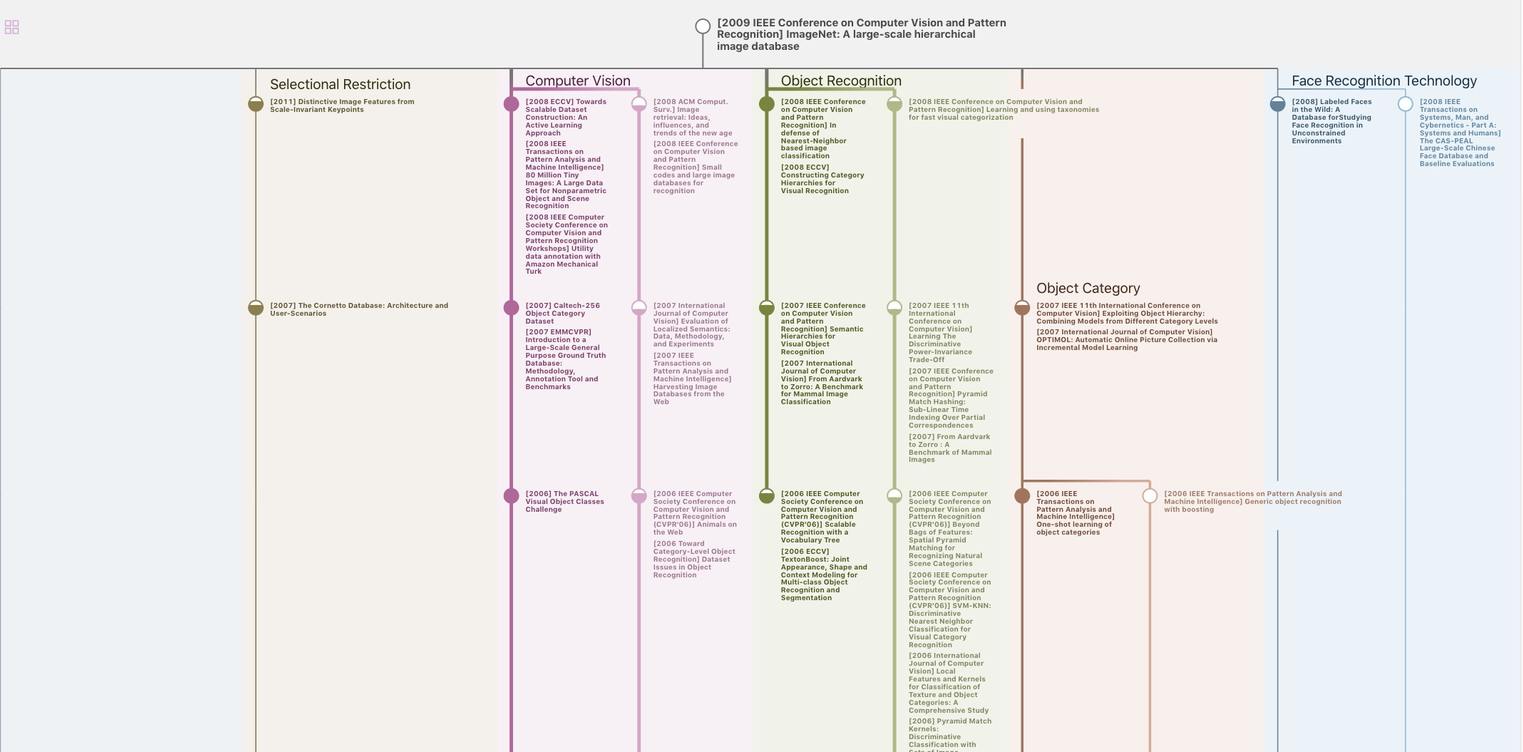
生成溯源树,研究论文发展脉络
Chat Paper
正在生成论文摘要