XRand: Differentially Private Defense Against Explanation-Guided Attacks
THIRTY-SEVENTH AAAI CONFERENCE ON ARTIFICIAL INTELLIGENCE, VOL 37 NO 10(2023)
摘要
Recent development in the field of explainable artificial intelligence (XAI) has helped improve trust in Machine-Learning-as-a-Service (MLaaS) systems, in which an explanation is provided together with the model prediction in response to each query. However, XAI also opens a door for adversaries to gain insights into the black-box models in MLaaS, thereby making the models more vulnerable to several attacks. For example, feature-based explanations (e.g., SHAP) could expose the top important features that a black-box model focuses on. Such disclosure has been exploited to craft effective backdoor triggers against malware classifiers. To address this trade-off, we introduce a new concept of achieving local differential privacy (LDP) in the explanations, and from that we establish a defense, called XRand, against such attacks. We show that our mechanism restricts the information that the adversary can learn about the top important features, while maintaining the faithfulness of the explanations.
更多查看译文
关键词
XAI Concepts,Differential Privacy,Machine Learning Interpretability,Interpretable Models,Black Box Models
AI 理解论文
溯源树
样例
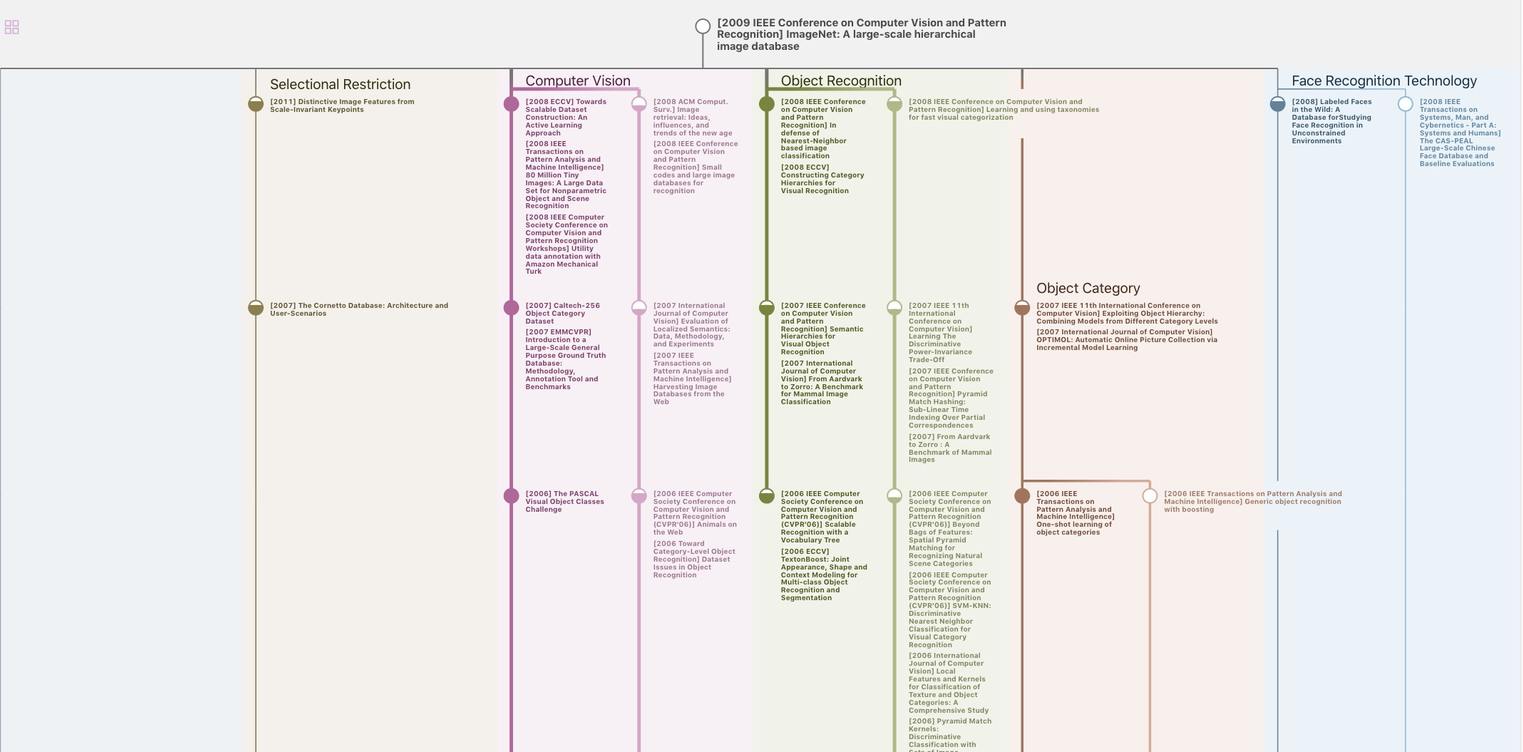
生成溯源树,研究论文发展脉络
Chat Paper
正在生成论文摘要