QoS Prediction and Adversarial Attack Protection for Distributed Services under DLaaS
IEEE TRANSACTIONS ON COMPUTERS(2024)
Hunan Univ | Shantou Univ | Providence Univ
Abstract
Deep-Learning-as-a-service (DLaaS) has received increasing attention due to its novelty as a diagram for deploying deep learning techniques. However, DLaaS faces performance and security issues that urgently need to be addressed. Given the limited computation resources and concern of benefits, Quality-of-Service (QoS) metrics should be revised to optimize the performance and reliability of distributed DLaaS systems. New users and services dynamically and continuously join and leave such a system, resulting in cold start issues, and additionally, the increasing demand for robust network connections requires the model to evaluate the uncertainty. To address such performance problems, we propose in this article a deep learning-based model called embedding enhanced probability neural network, in which information is extracted from inside the graph structure and then estimated the mean and variance values for the prediction distribution. The adversarial attack is a severe threat to model security under DLaaS. Due to such, the service recommender system's vulnerability is tackled, and adversarial training with uncertainty-aware loss to protect the model in noisy and adversarial environments is investigated and proposed. Extensive experiments on a large-scale real-world QoS dataset are conducted, and comprehensive analysis verifies the robustness and effectiveness of the proposed model.
MoreTranslated text
Key words
Quality of service,Internet of Things,Computational modeling,Deep learning,Security,Performance evaluation,Predictive models,Adversarial attacks,dlaas,graph neural network,probability forecast,qos prediction
求助PDF
上传PDF
View via Publisher
AI Read Science
AI Summary
AI Summary is the key point extracted automatically understanding the full text of the paper, including the background, methods, results, conclusions, icons and other key content, so that you can get the outline of the paper at a glance.
Example
Background
Key content
Introduction
Methods
Results
Related work
Fund
Key content
- Pretraining has recently greatly promoted the development of natural language processing (NLP)
- We show that M6 outperforms the baselines in multimodal downstream tasks, and the large M6 with 10 parameters can reach a better performance
- We propose a method called M6 that is able to process information of multiple modalities and perform both single-modal and cross-modal understanding and generation
- The model is scaled to large model with 10 billion parameters with sophisticated deployment, and the 10 -parameter M6-large is the largest pretrained model in Chinese
- Experimental results show that our proposed M6 outperforms the baseline in a number of downstream tasks concerning both single modality and multiple modalities We will continue the pretraining of extremely large models by increasing data to explore the limit of its performance
Upload PDF to Generate Summary
Must-Reading Tree
Example
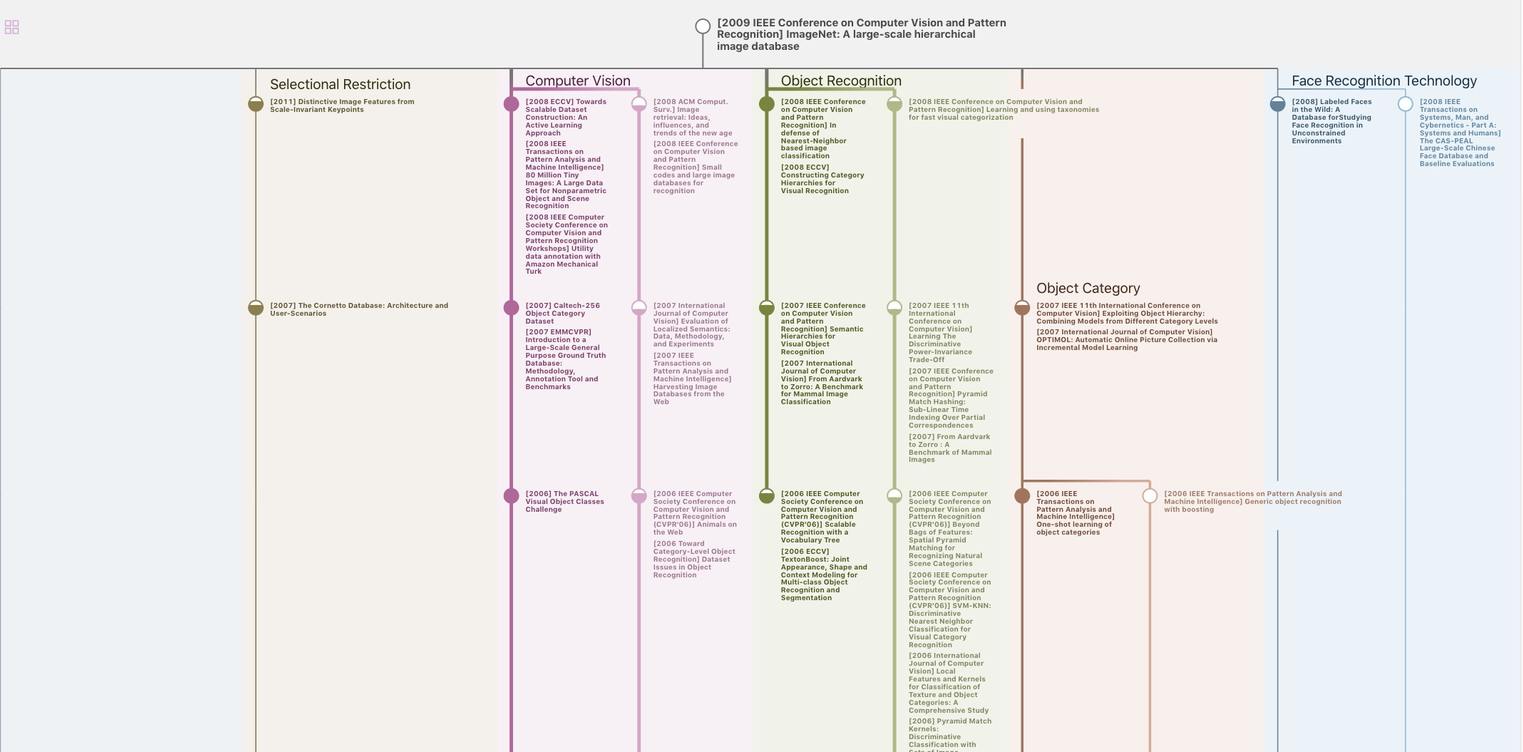
Generate MRT to find the research sequence of this paper
Related Papers
2012
被引用523 | 浏览
2014
被引用9 | 浏览
2015
被引用47 | 浏览
2015
被引用93 | 浏览
2015
被引用48 | 浏览
2017
被引用41 | 浏览
2019
被引用629 | 浏览
2019
被引用294 | 浏览
2020
被引用187 | 浏览
2020
被引用169 | 浏览
2020
被引用244 | 浏览
2020
被引用203 | 浏览
2022
被引用190 | 浏览
2019
被引用125 | 浏览
Data Disclaimer
The page data are from open Internet sources, cooperative publishers and automatic analysis results through AI technology. We do not make any commitments and guarantees for the validity, accuracy, correctness, reliability, completeness and timeliness of the page data. If you have any questions, please contact us by email: report@aminer.cn
Chat Paper