The Application of the Depth Residual Network Denoising Method in the Complex Mountainous Seismic Exploration
Springer series in geomechanics and geoengineering(2023)
摘要
Due to topographical changes, the SNR for seismic data in complex mountainous areas is low, making complex structural interpretation more challenging. Seismic data from complex mountain exploration has entered the era of massive data volume with the development of complex mountain exploration. It is critical to create efficient, high-precision seismic data denoising methods. Seismic data denoising processing is the first step in seismic data processing; denoising processing based on different noise is the purpose of the formation mechanism, characteristics, choose a different denoising method, to achieve the optimal signal-to-noise ratio of seismic data, thereby reducing the uncertainty of seismic data interpretation. The denoising effect has an immediate impact on the quality of subsequent seismic data processing. This paper proposes a kind of based on the depth of the residual convolution denoising of seismic data processing method of neural network, and build the residual convolutional neural network model, output and noise of the L2 norm for loss function, using the deeper structure and learning algorithm of residual, in order to efficiently remove noise. The addition of the feedforward conduction process of the normalized layer and network layer improves network training speed and denoising performance while effectively suppressing random noise in seismic data. Experiments on real seismic data show that the residual convolutional neural network can improve deep learning performance and produce good denoising results, demonstrating the deep residual neural network’s excellent performance in seismic data denoising.
更多查看译文
AI 理解论文
溯源树
样例
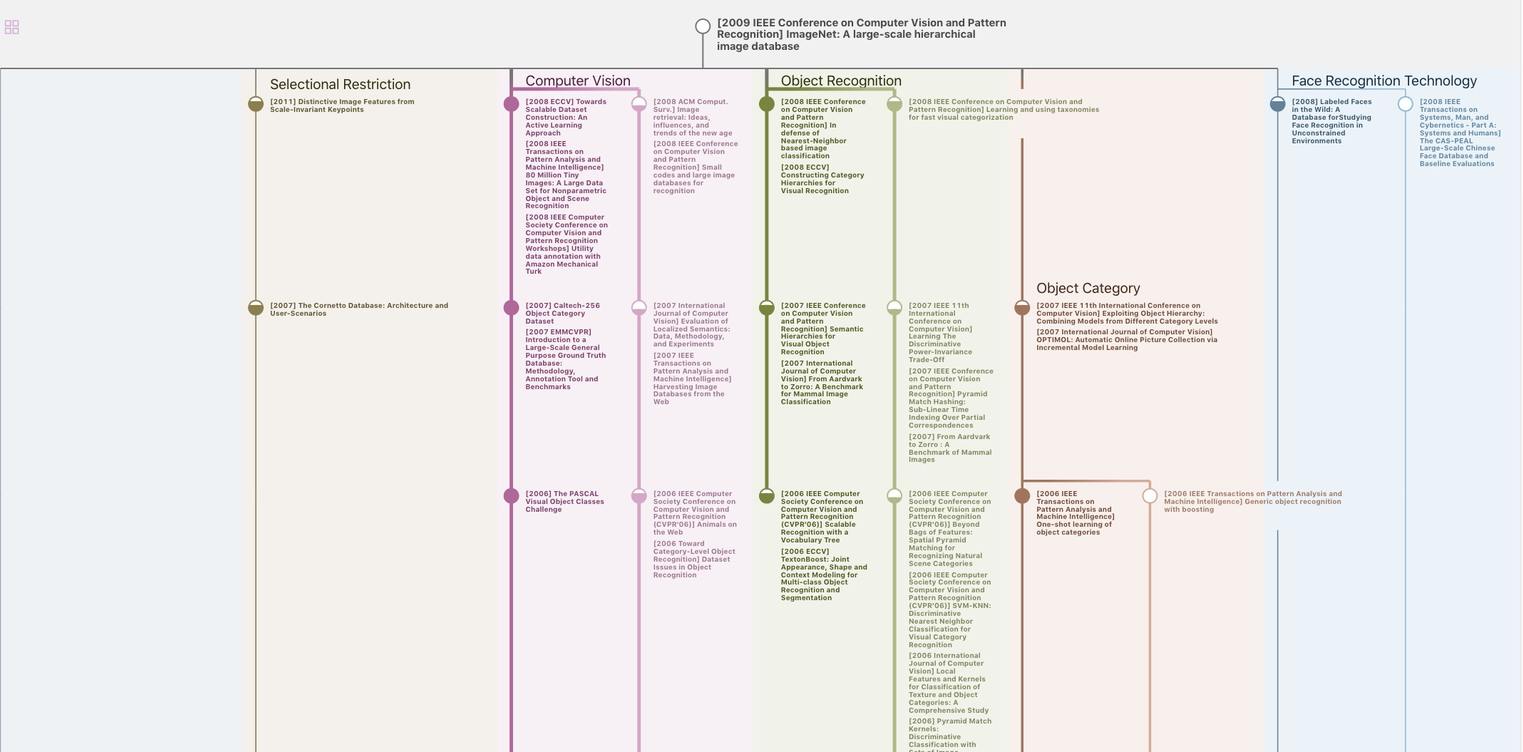
生成溯源树,研究论文发展脉络
Chat Paper
正在生成论文摘要