Sonar image segmentation framework based on semi-supervised learning
Research Square (Research Square)(2023)
摘要
Abstract Sonar image object detection, as a special image processing task, has been facing the two problems of severe noise impact and scarce data resources. In this paper, we propose a semi-supervised learning based sonar image object segmentation network (SISN). We use Mask RCNN as the base network and design a side flap noise generation module to make the model more robust to noise. In order to reduce the labeling of training samples, we introduce the concept of semi-supervised learning during the training process. We train the teacher model using a small amount of labeled data, thus generating pseudo-labels for the unlabeled samples. This is then supplemented with new data augmentation to improve the model’s resistance to sample perturbation and the prediction confidence of the pseudo-labeled data, thus optimizing the entire SISN training process. We conducted exhaustive experiments on the collected dataset, and the experimental results show that we can achieve high localization accuracy using less data for training, and show strong noise robustness on noisy samples.
更多查看译文
关键词
image segmentation,learning,semi-supervised
AI 理解论文
溯源树
样例
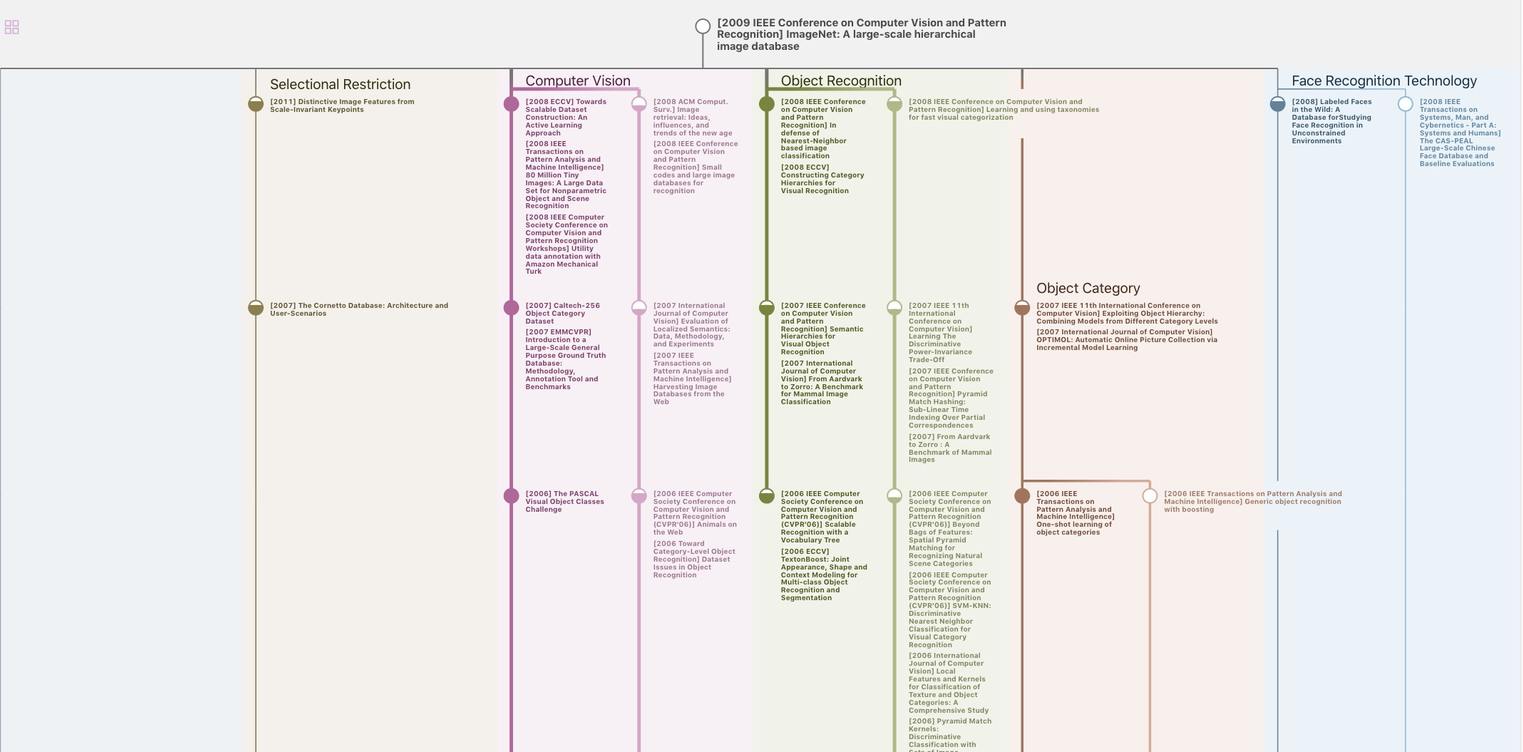
生成溯源树,研究论文发展脉络
Chat Paper
正在生成论文摘要