Learning Audio and Video Bitrate Selection Strategies via Explicit Requirements
IEEE TRANSACTIONS ON MOBILE COMPUTING(2024)
摘要
Mobile video streaming dominates today's network traffic, and adaptive bitrate (ABR) algorithms have been routinely adopted for transmitting media content across dynamic mobile networks. State-of-the-art ABR algorithms mainly alter video bitrate without considering audio bitrate as they consider the impact on the video negligible due to their small size. However, to bring users an immersive experience, recent content providers have applied high-quality audio with large sizes, like stereophonic sound. Therefore, improper audio bitrate selection will adversely affect video bitrate selection, leading to undesirable audio/video combinations (the highest video quality with the lowest audio quality, and vice versa) and frequent playback interruptions. To address these inefficiencies, we propose a Self-Play reinforcement learning-based Audio-aware ABR algorithm named SPA to learn strategies for audio and video bitrate selections. By learning from explicit goals, SPA can match the actual requirements and attain good performance. By conducting trace-driven and testbed-based experiments, we observe SPA's considerable superiority compared to existing approaches, including reducing the undesirable combinations by up to 34.17x and achieving zero stall time across 88.57% of traces. We also invite 35 volunteers to join a subjective test, and the result shows that 33/35 people consider SPA provides them with a satisfactory viewing experience.
更多查看译文
关键词
Bit rate,Streaming media,Reinforcement learning,Quality of experience,Mobile computing,Video on demand,Training,Bitrate adaption,DASH,self-play reinforcement learning
AI 理解论文
溯源树
样例
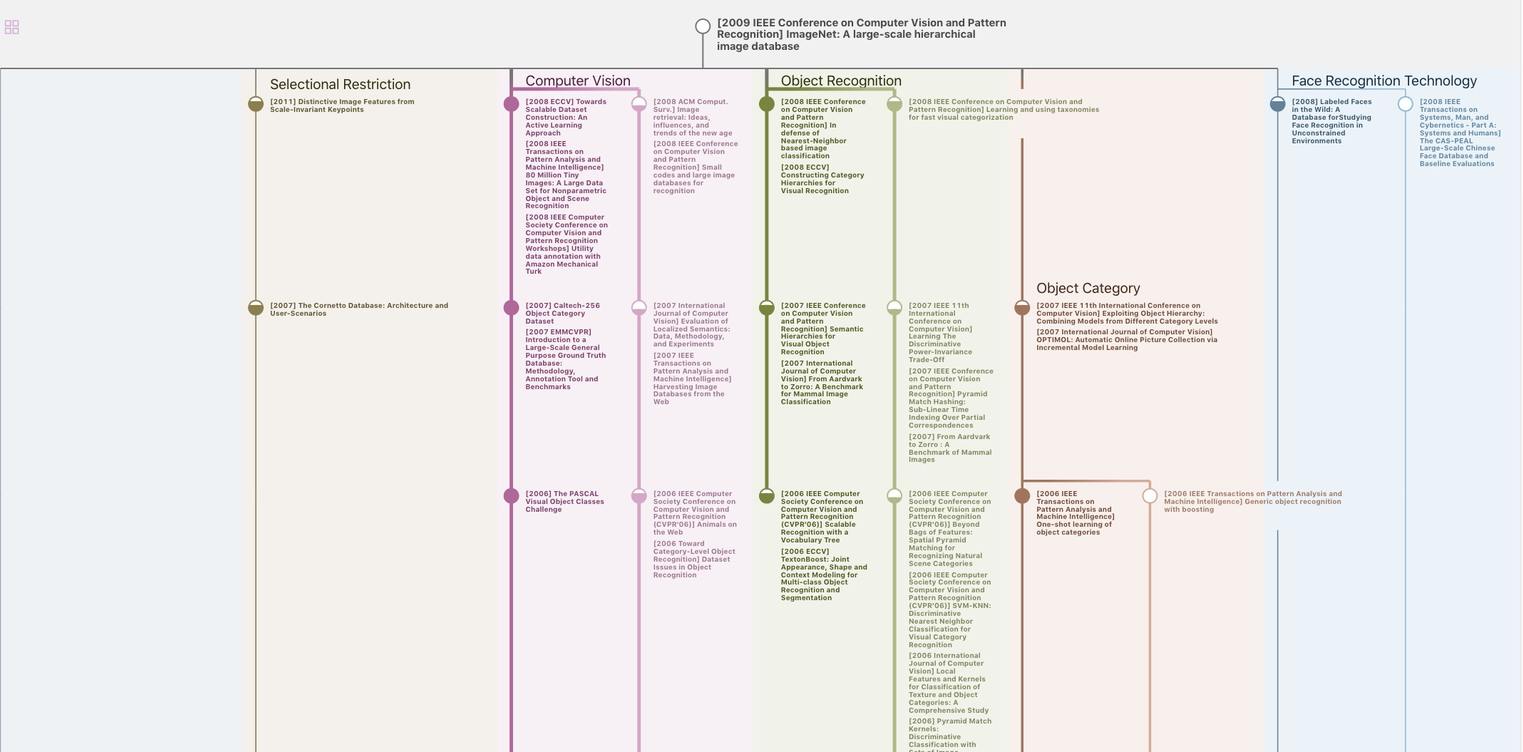
生成溯源树,研究论文发展脉络
Chat Paper
正在生成论文摘要