Exploring validation metrics for offline model-based optimisation
arXiv (Cornell University)(2022)
摘要
In offline model-based optimisation (MBO) we are interested in using machine learning to design candidates that maximise some measure of desirability through an expensive but real-world scoring process. Offline MBO tries to approximate this expensive scoring function and use that to evaluate generated designs, however evaluation is non-exact because one approximation is being evaluated with another. Instead, we ask ourselves: if we did have the real world scoring function at hand, what cheap-to-compute validation metrics would correlate best with this? Since the real-world scoring function is available for simulated MBO datasets, insights obtained from this can be transferred over to real-world offline MBO tasks where the real-world scoring function is expensive to compute. To address this, we propose a conceptual evaluation framework that is amenable to measuring extrapolation, and apply this to conditional denoising diffusion models. Empirically, we find that two validation metrics -- agreement and Frechet distance -- correlate quite well with the ground truth. When there is high variability in conditional generation, feedback is required in the form of an approximated version of the real-world scoring function. Furthermore, we find that generating high-scoring samples may require heavily weighting the generative model in favour of sample quality, potentially at the cost of sample diversity.
更多查看译文
关键词
validation metrics,optimisation,model-based
AI 理解论文
溯源树
样例
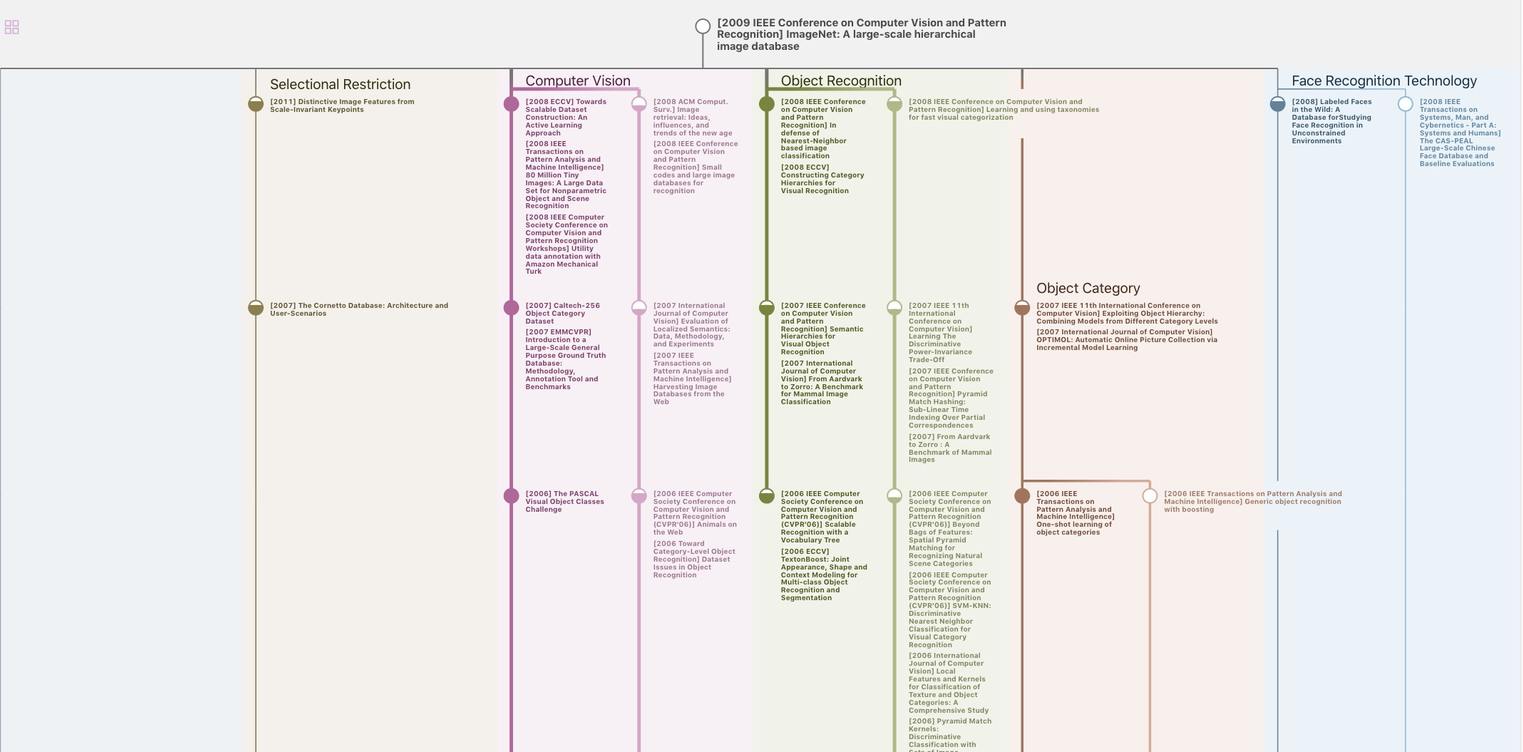
生成溯源树,研究论文发展脉络
Chat Paper
正在生成论文摘要