Time2Feat
Proceedings of the VLDB Endowment(2022)
摘要
Clustering multivariate time series is a critical task in many real-world applications involving multiple signals and sensors. Existing systems aim to maximize effectiveness, efficiency and scalability, but fail to guarantee the interpretability of the results. This hinders their application in critical real scenarios where human comprehension of algorithmic behavior is required. This paper introduces Time2Feat, an end-to-end machine learning system for multivariate time series (MTS) clustering. The system relies on inter-signal and intra-signal interpretable features extracted from the time series. Then, a dimensionality reduction technique is applied to select a subset of features that retain most of the information, thus enhancing the interpretability of the results. In addition, domain experts can semi-supervise the process, by providing a small amount of MTS with a target cluster. This process further improves both accuracy and interpretability, narrowing down the number of features used by the clustering process. We demonstrate the effectiveness, interpretability, efficiency, and robustness of Time2Feat through experiments on eighteen benchmarking time series datasets, comparing them with state-of-the-art MTS clustering methods.
更多查看译文
关键词
time2feat
AI 理解论文
溯源树
样例
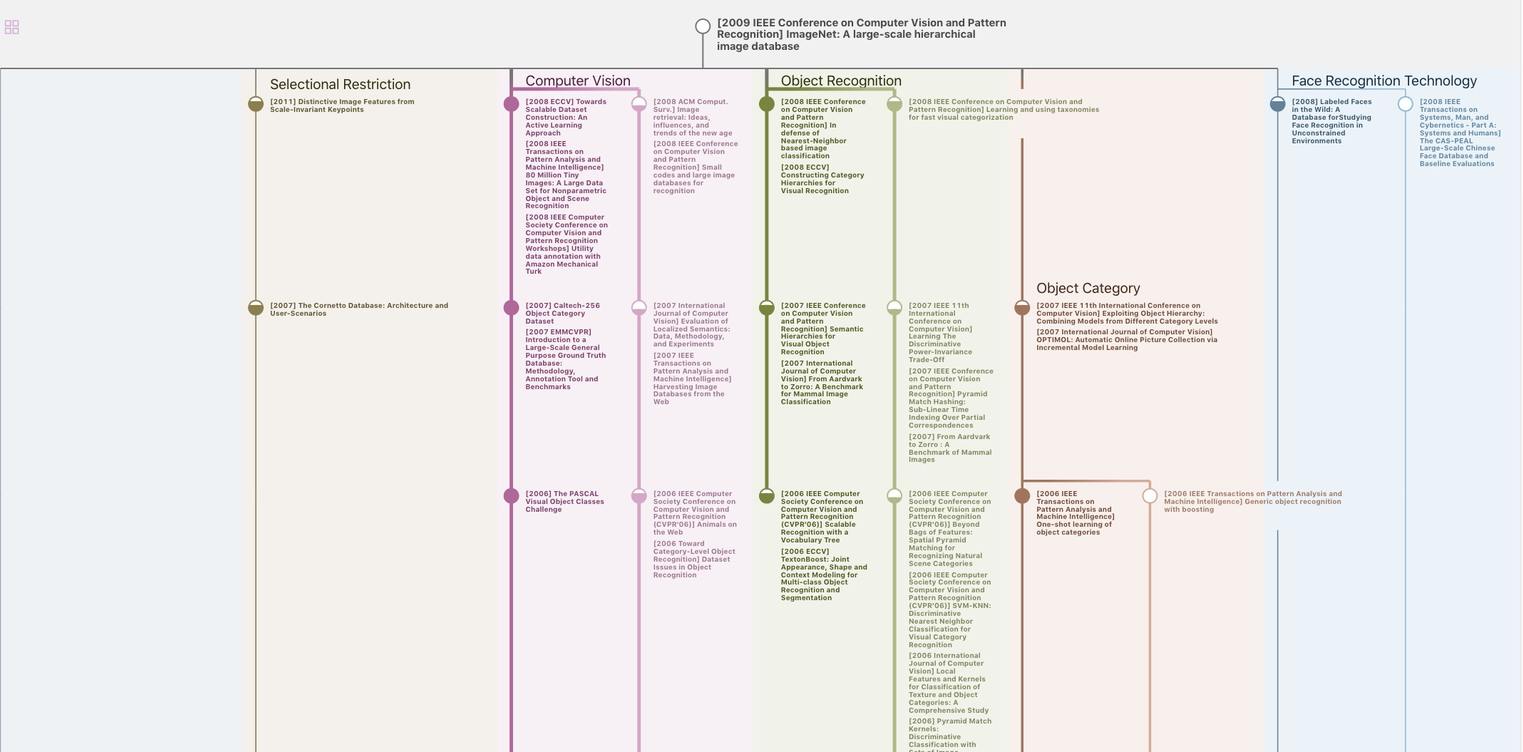
生成溯源树,研究论文发展脉络
Chat Paper
正在生成论文摘要