Q-TART: Quickly Training for Adversarial Robustness and In-Transferability
arXiv (Cornell University)(2022)
摘要
Raw deep neural network (DNN) performance is not enough; in real-world settings, computational load, training efficiency and adversarial security are just as or even more important. We propose to simultaneously tackle Performance, Efficiency, and Robustness, using our proposed algorithm Q-TART, Quickly Train for Adversarial Robustness and in-Transferability. Q-TART follows the intuition that samples highly susceptible to noise strongly affect the decision boundaries learned by DNNs, which in turn degrades their performance and adversarial susceptibility. By identifying and removing such samples, we demonstrate improved performance and adversarial robustness while using only a subset of the training data. Through our experiments we highlight Q-TART's high performance across multiple Dataset-DNN combinations, including ImageNet, and provide insights into the complementary behavior of Q-TART alongside existing adversarial training approaches to increase robustness by over 1.3% while using up to 17.9% less training time.
更多查看译文
关键词
Robustness,Deep Learning,Adversarial Examples
AI 理解论文
溯源树
样例
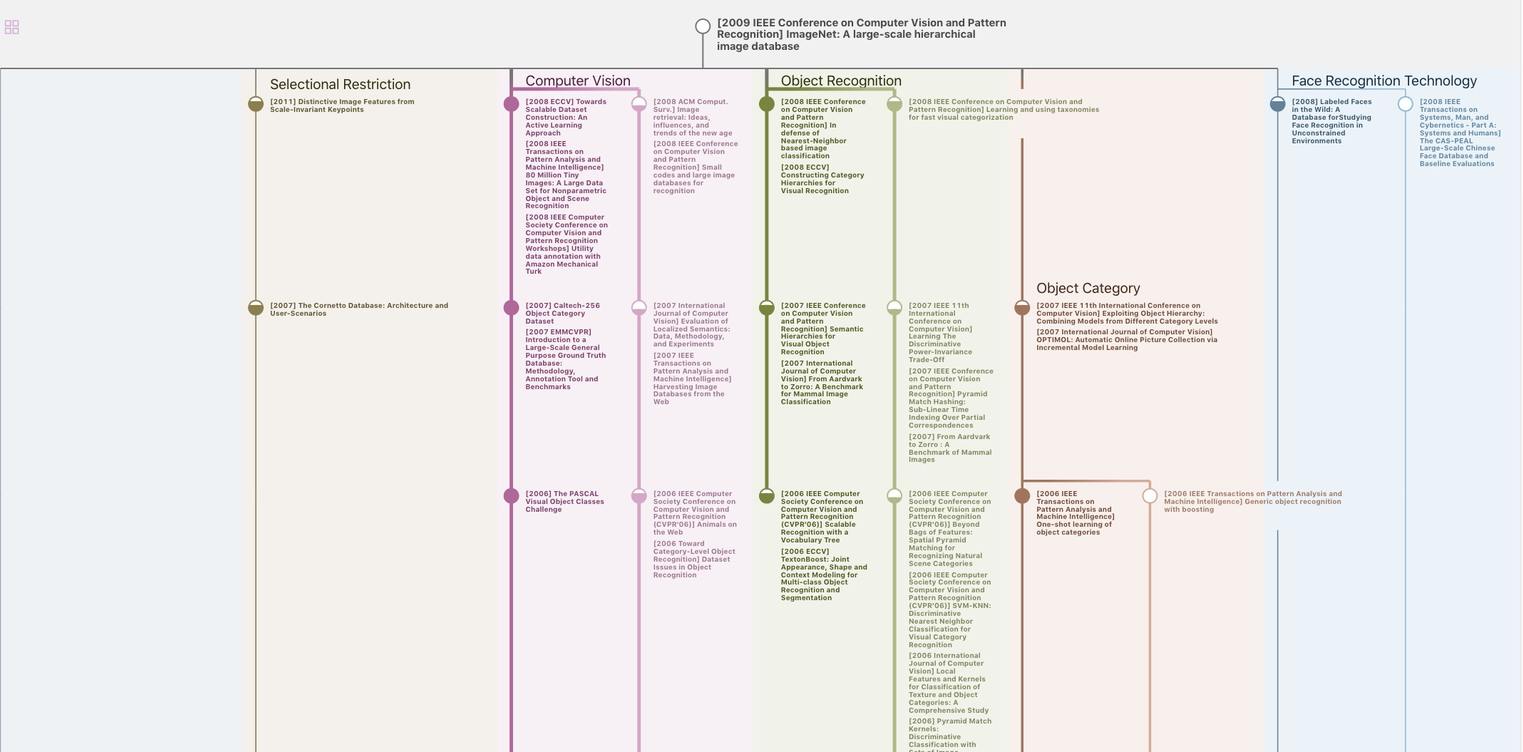
生成溯源树,研究论文发展脉络
Chat Paper
正在生成论文摘要