A Unified Modular Framework to Incorporate Structural Dependency in Spatial Omics Data
bioRxiv (Cold Spring Harbor Laboratory)(2022)
摘要
Abstract Spatial omics technologies can help identify spatially organized biological processes, but existing computational approaches often overlook structural dependencies in the data. Here, we introduce Smoother, a unified framework that integrates positional information into non-spatial models via modular priors and losses. In simulated and real datasets, Smoother enables accurate data imputation, cell-type deconvolution, and dimensionality reduction with remarkable efficiency. In colorectal cancer, Smoother-guided deconvolution revealed plasma cell and fibroblast subtype localizations linked to tumor microenvironment restructuring. Additionally, joint modeling of spatial and single-cell human prostate data with Smoother allowed for spatial mapping of reference populations with significantly reduced ambiguity.
更多查看译文
关键词
structural dependency,spatial,unified modular framework,data
AI 理解论文
溯源树
样例
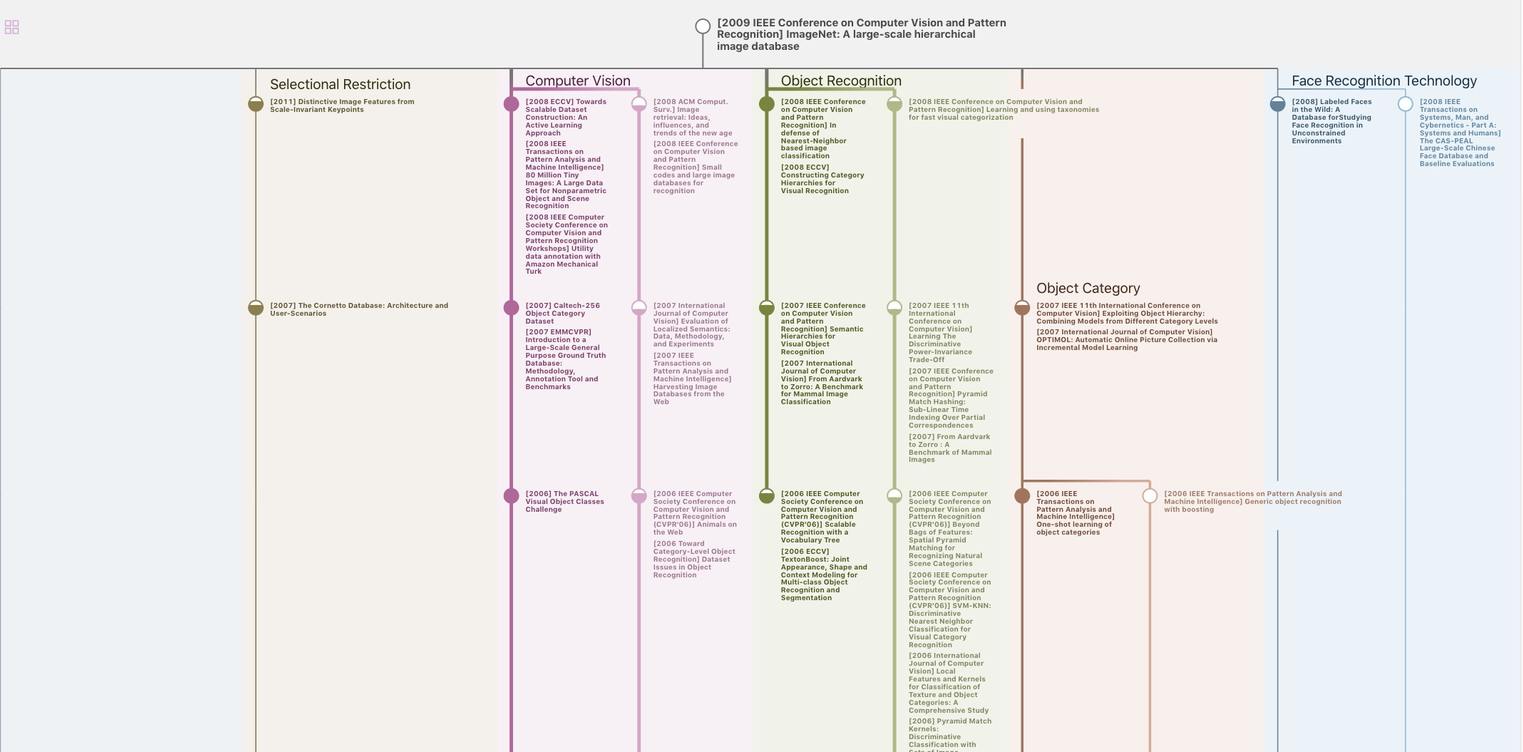
生成溯源树,研究论文发展脉络
Chat Paper
正在生成论文摘要