Physics-constrained machine learning for thermal turbulence modelling at low Prandtl numbers
arXiv (Cornell University)(2022)
摘要
Liquid metals play a central role in new generation liquid metal cooled nuclear reactors, for which numerical investigations require the use of appropriate thermal turbulence models for low Prandtl number fluids. Given the limitations of traditional modelling approaches and the increasing availability of high-fidelity data for this class of fluids, we propose a Machine Learning strategy for the modelling of the turbulent heat flux. A comprehensive algebraic mathematical structure is derived and physical constraints are imposed to ensure attractive properties promoting applicability, robustness and stability. The closure coefficients of the model are predicted by an Artificial Neural Network (ANN) which is trained with DNS data at different Prandtl numbers. The validity of the approach was verified through a priori and a posteriori validation for two and three-dimensional liquid metal flows. The model provides a complete vectorial representation of the turbulent heat flux and the predictions fit the DNS data in a wide range of Prandtl numbers (Pr=0.01-0.71). The comparison with other existing thermal models shows that the methodology is very promising.
更多查看译文
关键词
thermal turbulence,machine learning,modelling,physics-constrained
AI 理解论文
溯源树
样例
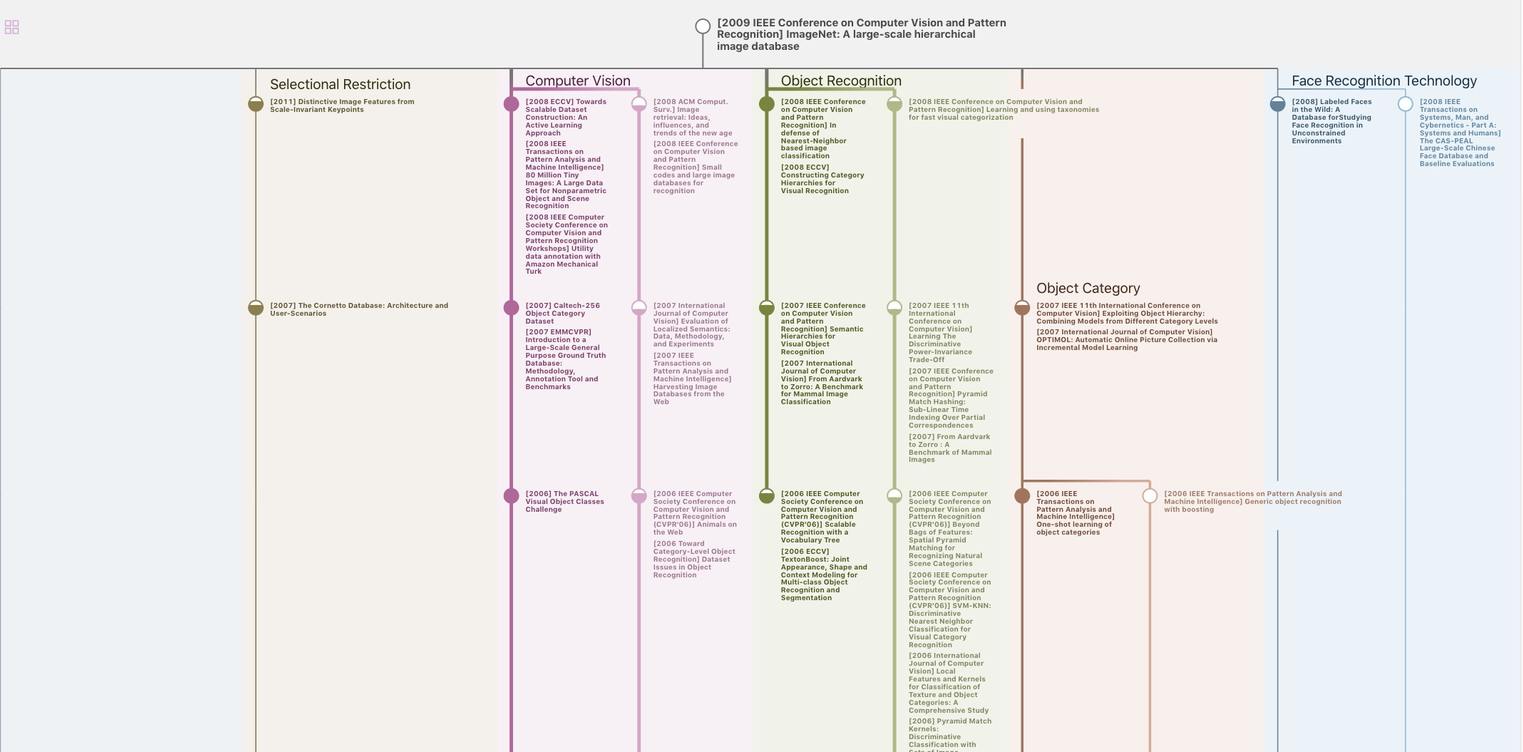
生成溯源树,研究论文发展脉络
Chat Paper
正在生成论文摘要