Benchmarking Modern Named Entity Recognition Techniques for Free-text Health Record Deidentification
AMIA Summits on Translational Science proceedings(2021)
摘要
Electronic Health Records (EHRs) have become the primary form of medical data-keeping across the United States. Federal law restricts the sharing of any EHR data that contains protected health information (PHI). De-identification, the process of identifying and removing all PHI, is crucial for making EHR data publicly available for scientific research. This project explores several deep learning-based named entity recognition (NER) methods to determine which method(s) perform better on the de-identification task. We trained and tested our models on the i2b2 training dataset, and qualitatively assessed their performance using EHR data collected from a local hospital. We found that 1) Bi-LSTM-CRF represents the best-performing encoder/decoder combination, 2) character-embeddings tend to improve precision at the price of recall, and 3) transformers alone under-perform as context encoders. Future work focused on structuring medical text may improve the extraction of semantic and syntactic information for the purposes of EHR deidentification.
更多查看译文
关键词
Named Entity Recognition
AI 理解论文
溯源树
样例
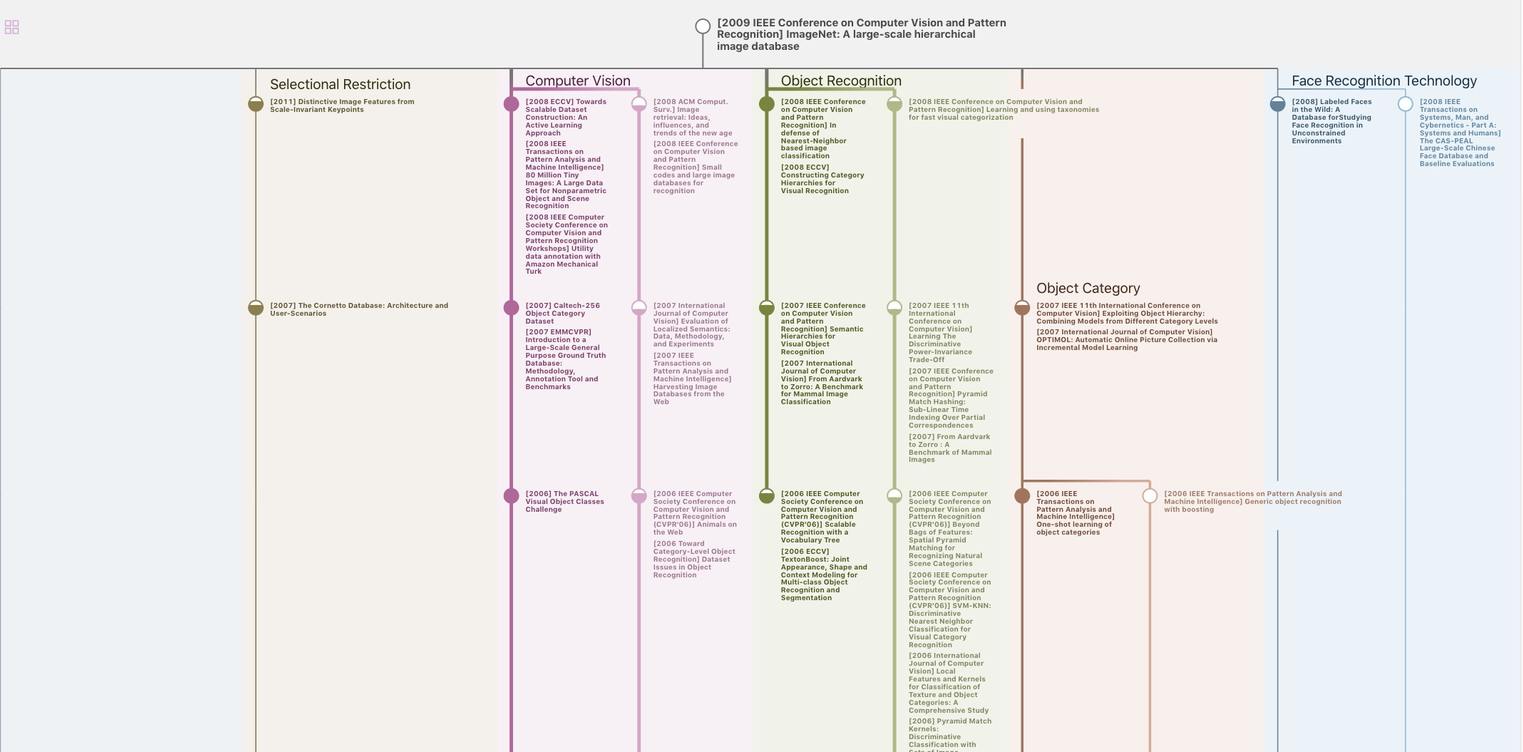
生成溯源树,研究论文发展脉络
Chat Paper
正在生成论文摘要