FlipDA: Effective and Robust Data Augmentation for Few-Shot Learning
arXiv (Cornell University)(2021)
摘要
Most previous methods for text data augmentation are limited to simple tasks and weak baselines. We explore data augmentation on hard tasks (i.e., few-shot natural language understanding) and strong baselines (i.e., pretrained models with over one billion parameters). Under this setting, we reproduced a large number of previous augmentation methods and found that these methods bring marginal gains at best and sometimes degrade the performance much. To address this challenge, we propose a novel data augmentation method FlipDA that jointly uses a generative model and a classifier to generate label-flipped data. Central to the idea of FlipDA is the discovery that generating label-flipped data is more crucial to the performance than generating label-preserved data. Experiments show that FlipDA achieves a good tradeoff between effectiveness and robustness -- it substantially improves many tasks while not negatively affecting the others.
更多查看译文
关键词
robust data augmentation,learning,flipda,few-shot
AI 理解论文
溯源树
样例
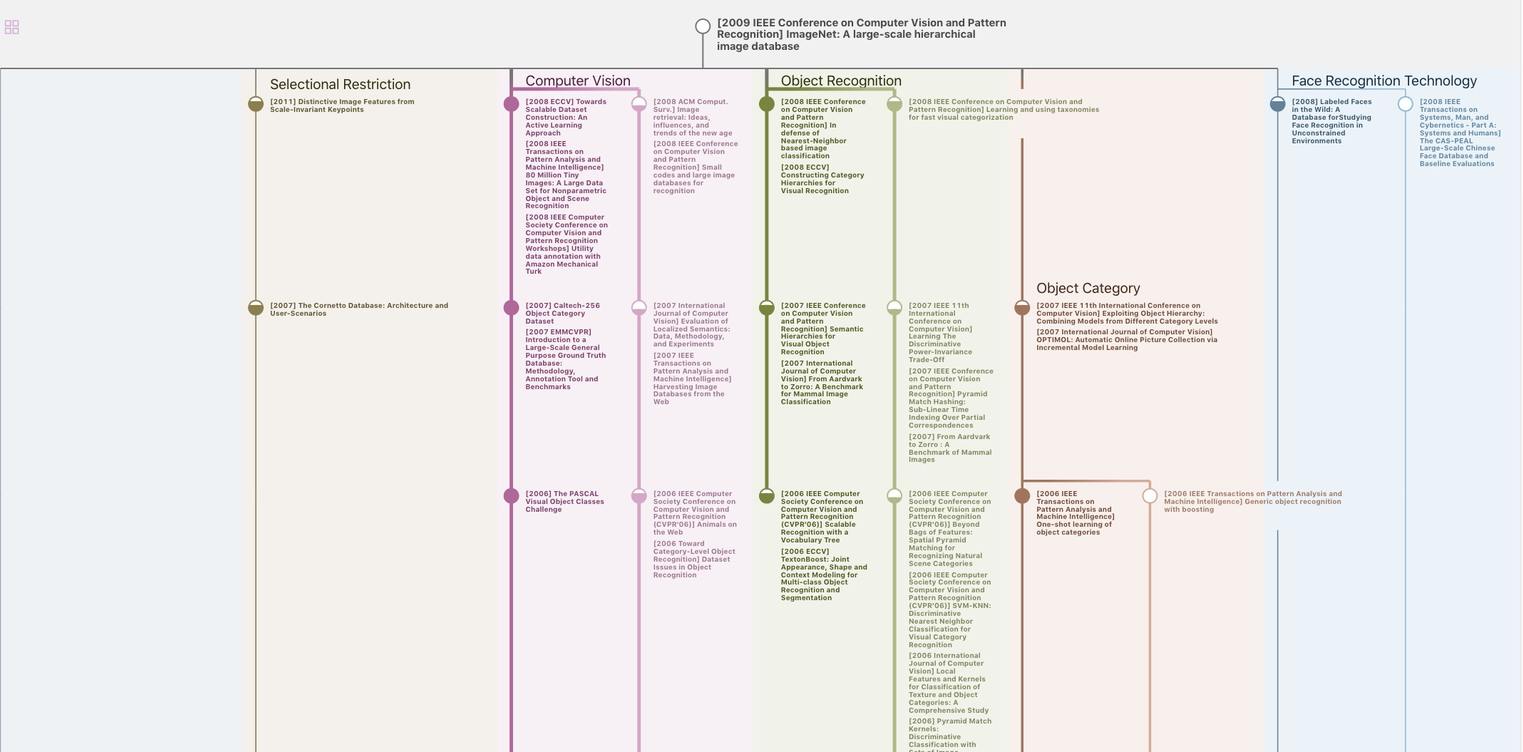
生成溯源树,研究论文发展脉络
Chat Paper
正在生成论文摘要