Learning to Noise: Application-Agnostic Data Sharing with Local Differential Privacy
arXivorg(2020)
摘要
In recent years, the collection and sharing of individuals’ private data has become commonplace in many industries. Local differential privacy (LDP) is a rigorous approach which uses a randomized algorithm to preserve privacy even from the database administrator, unlike the more standard central differential privacy. For LDP, when applying noise directly to high-dimensional data, the level of noise required all but entirely destroys data utility. In this paper we introduce a novel, application-agnostic privatization mechanism that leverages representation learning to overcome the prohibitive noise requirements of direct methods, while maintaining the strict guarantees of LDP. We further demonstrate that data privatized with this mechanism can be used to train machine learning algorithms. Applications of this model include private data collection, private novel-class classification, and the augmentation of clean datasets with additional privatized features. We achieve significant gains in performance on downstream classification tasks relative to benchmarks that noise the data directly, which are state-of-the-art in the context of application-agnostic LDP mechanisms for high-dimensional data sharing tasks.
更多查看译文
关键词
Differential Privacy,Privacy-Preserving Computation,Location Privacy,Anonymization,Attribute-Based Encryption
AI 理解论文
溯源树
样例
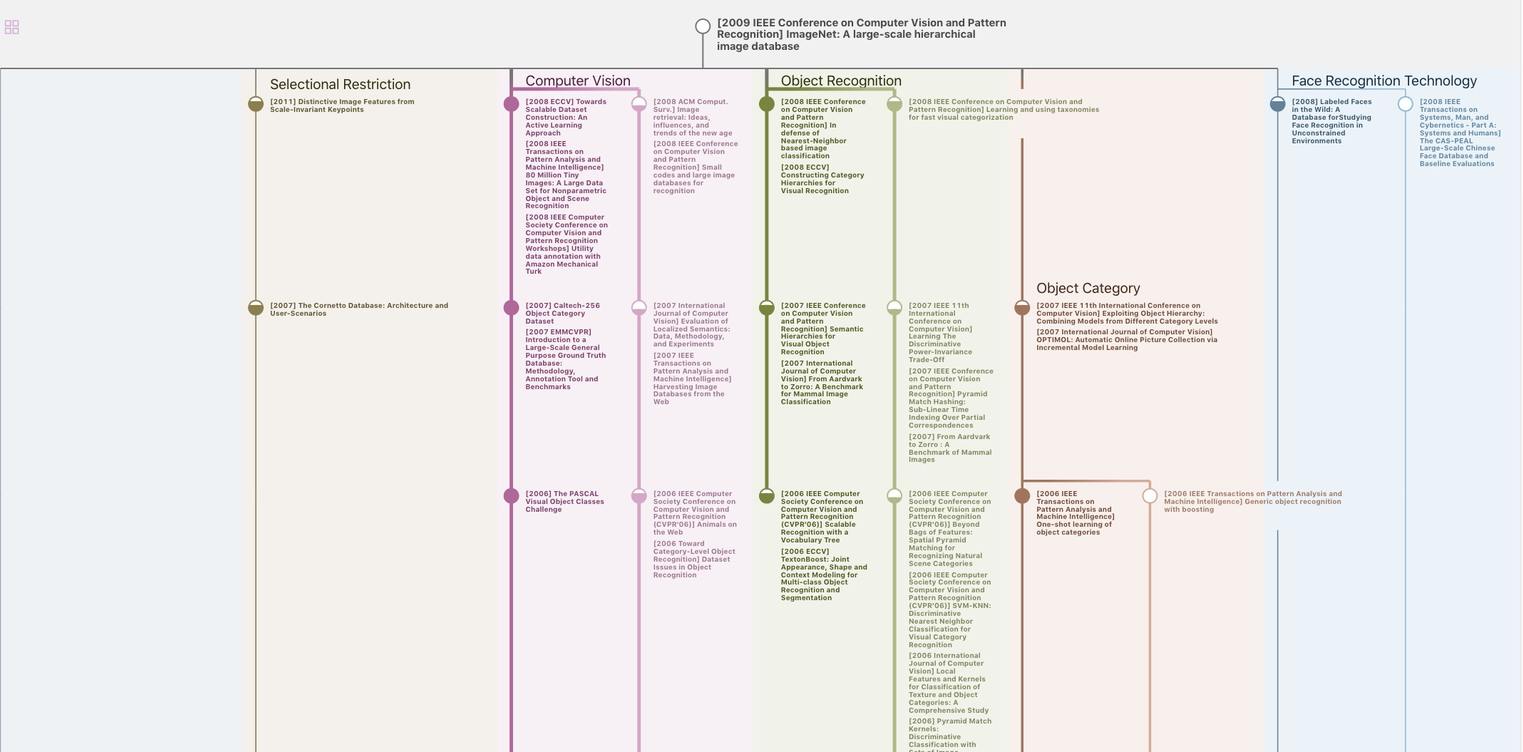
生成溯源树,研究论文发展脉络
Chat Paper
正在生成论文摘要