Beyond Cuts in Small Signal Scenarios - Enhanced Sneutrino Detectability Using Machine Learning
arXiv (Cornell University)(2021)
摘要
We investigate enhancing the sensitivity of new physics searches at the LHC by machine learning in the case of background dominance and a high degree of overlap between the observables for signal and background. We use two different models, XGBoost and a deep neural network, to exploit correlations between observables and compare this approach to the traditional cut-and-count method. We consider different methods to analyze the models' output, finding that a template fit generally performs better than a simple cut. By means of a Shapley decomposition, we gain additional insight into the relationship between event kinematics and the machine learning model output. We consider a supersymmetric scenario with a metastable sneutrino as a concrete example, but the methodology can be applied to a much wider class of supersymmetric models.
更多查看译文
关键词
enhanced sneutrino detectability,small signal scenarios,machine learning
AI 理解论文
溯源树
样例
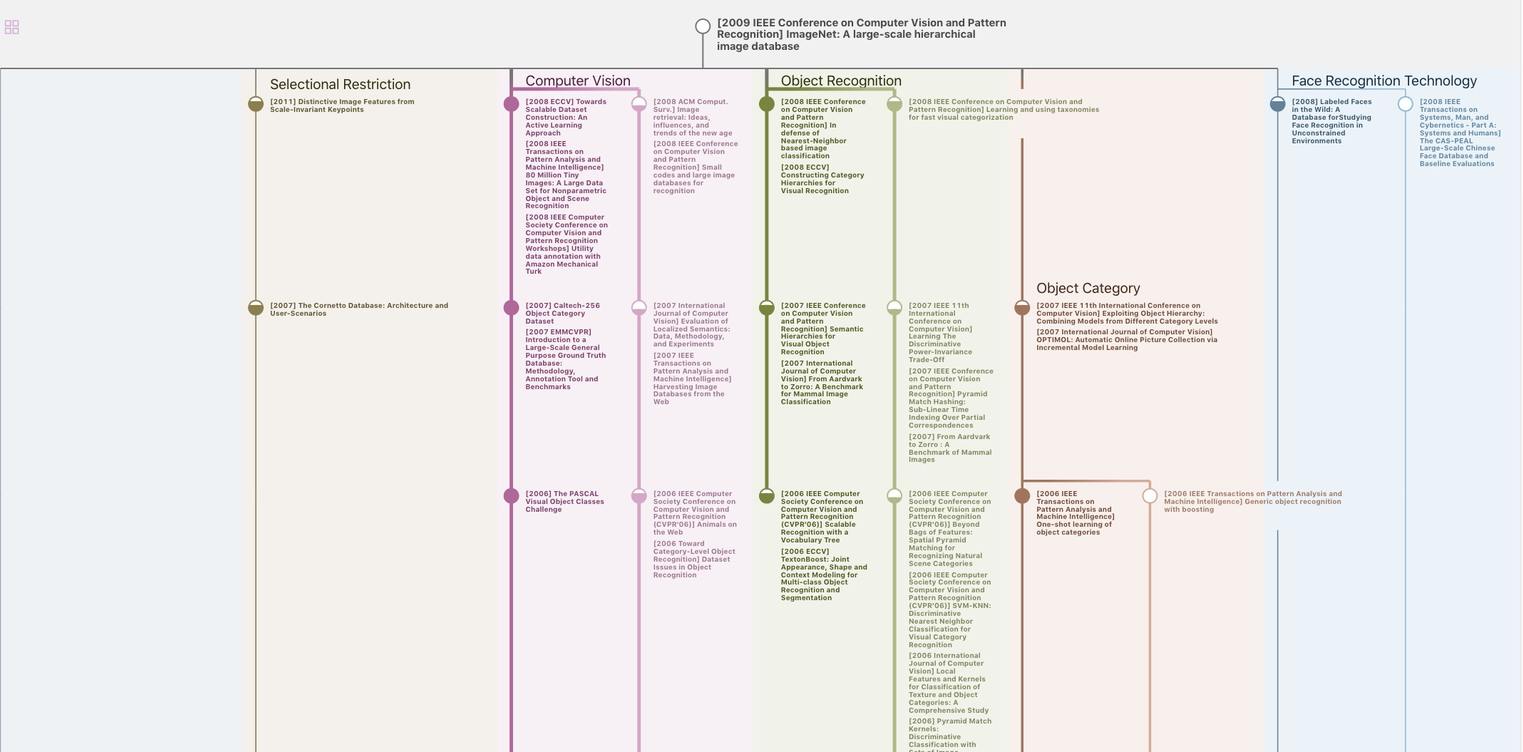
生成溯源树,研究论文发展脉络
Chat Paper
正在生成论文摘要