Computer Assisted Translation with Neural Quality Estimation and Automatic Post-Editing
FINDINGS OF THE ASSOCIATION FOR COMPUTATIONAL LINGUISTICS, EMNLP 2020(2020)
摘要
With the advent of neural machine translation, there has been a marked shift towards leveraging and consuming the machine translation results. However, the gap between machine translation systems and human translators needs to be manually closed by post-editing. In this paper, we propose an end-to-end deep learning framework of the quality estimation and automatic post-editing of the machine translation output. Our goal is to provide error correction suggestions and to further relieve the burden of human translators through an interpretable model. To imitate the behavior of human translators, we design three efficient delegation modules - quality estimation, generative post-editing, and atomic operation post-editing and construct a hierarchical model based on them. We examine this approach with the English - German dataset from WMT 2017 APE shared task and our experimental results can achieve the state-of-the-art performance. We also verify that the certified translators can significantly expedite their post-editing processing with our model in human evaluation.
更多查看译文
AI 理解论文
溯源树
样例
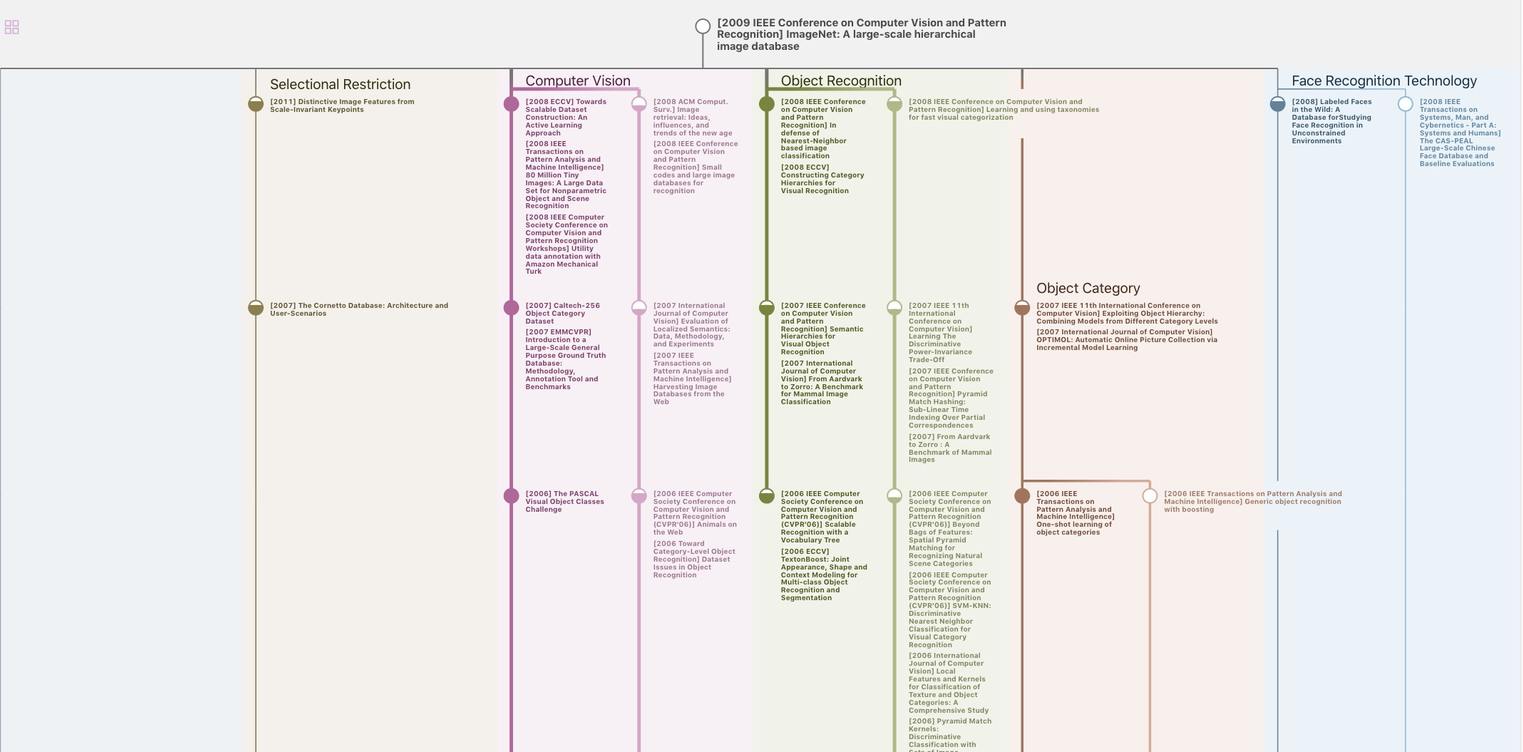
生成溯源树,研究论文发展脉络
Chat Paper
正在生成论文摘要