QTSumm: Query-Focused Summarization over Tabular Data.
EMNLP 2023(2023)
摘要
People primarily consult tables to conduct data analysis or answer specific questions. Text generation systems that can provide accurate table summaries tailored to users' information needs can facilitate more efficient access to relevant data insights.
Motivated by this, we define a new query-focused table summarization task, where text generation models have to perform human-like reasoning and analysis over the given table to generate a tailored summary. We introduce a new benchmark named QTSumm for this task, which contains 7,111 human-annotated query-summary pairs over 2,934 tables covering diverse topics.
We investigate a set of strong baselines on QTSumm, including text generation, table-to-text generation, and large language models.
Experimental results and manual analysis reveal that the new task presents significant challenges in table-to-text generation for future research.
Moreover, we propose a new approach named ReFactor, to retrieve and reason over query-relevant information from tabular data to generate several natural language facts. Experimental results demonstrate that ReFactor can bring effective improvements to baselines by concatenating the generated facts to the model input.
Our data and code are publicly available at https://github.com/yale-nlp/QTSumm.
更多查看译文
关键词
new benchmark,table,query-focused
AI 理解论文
溯源树
样例
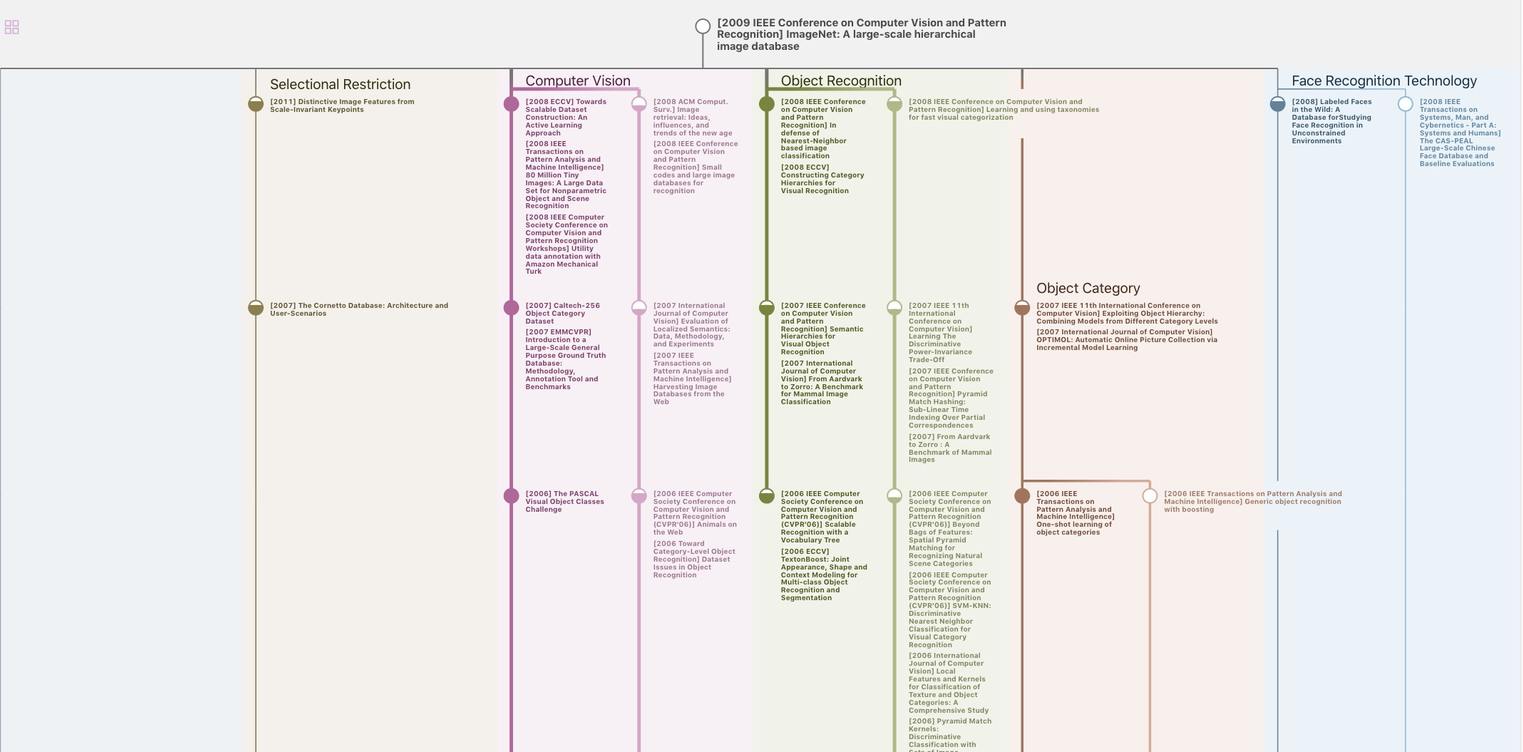
生成溯源树,研究论文发展脉络
Chat Paper
正在生成论文摘要