Research on the Agricultural Pest Identification Mechanism Based on an Intelligent Algorithm
AGRICULTURE-BASEL(2023)
摘要
The use of Internet of Things (IoT) technology for real-time monitoring of agricultural pests is an unavoidable trend in the future of intelligent agriculture. This paper aims to address the difficulties in deploying models at the edge of the pest monitoring visual system and the low recognition accuracy. In order to achieve that, a lightweight GCSS-YOLOv5s algorithm is proposed. Firstly, we introduce the lightweight network GhostNet, use the Ghostconv module to replace the traditional convolution, and construct the C3Ghost module based on the CSP structure, drastically reducing the number of model parameters. Secondly, during the feature fusion process, we introduce the content-aware reassembly of features (CARAFE) lightweight up-sampling operator to enhance the feature integration capability of the pests by reducing the impact of redundant features after fusion. Then, we adopt SIoU as the bounding box regression loss function, which enhances the convergence speed and detection accuracy of the model. Finally, the traditional non-maximum suppression (NMS) was improved to Soft-NMS to improve the model’s ability to recognize overlapping pests. According to the experimental results, the mean average precision (mAP) of the GCSS-YOLOv5s model reaches 90.5%. This is achieved with a 44% reduction in the number of parameters and a 7.4 G reduction in computation volume compared to the original model. The method significantly reduces the model’s resource requirements while maintaining accuracy, which offers a specific theoretical foundation and technological reference for the future field of intelligent monitoring.
更多查看译文
关键词
pest monitoring,GCSS-YOLOv5s,GhostNet,content-aware reassembly of features,SIoU,soft-non-maximum suppression
AI 理解论文
溯源树
样例
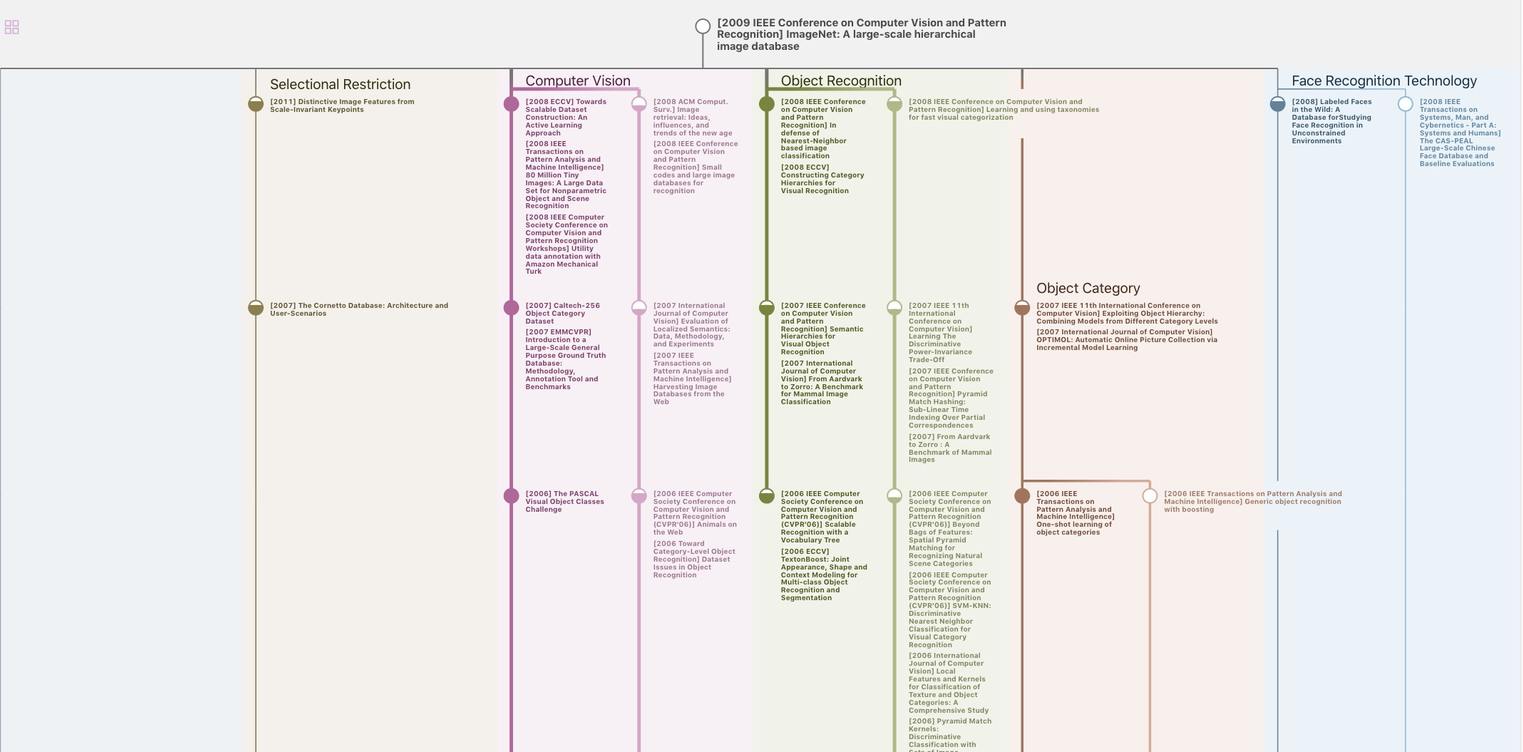
生成溯源树,研究论文发展脉络
Chat Paper
正在生成论文摘要