Proposal-based Multiple Instance Learning for Weakly-supervised Temporal Action Localization
CVPR 2023(2023)
摘要
Weakly-supervised temporal action localization aims to localize and recognize actions in untrimmed videos with only video-level category labels during training. Without instance-level annotations, most existing methods follow the Segment-based Multiple Instance Learning (S-MIL) framework, where the predictions of segments are supervised by the labels of videos. However, the objective for acquiring segment-level scores during training is not consistent with the target for acquiring proposal-level scores during testing, leading to suboptimal results. To deal with this problem, we propose a novel Proposal-based Multiple Instance Learning (P-MIL) framework that directly classifies the candidate proposals in both the training and testing stages, which includes three key designs: 1) a surrounding contrastive feature extraction module to suppress the discriminative short proposals by considering the surrounding contrastive information, 2) a proposal completeness evaluation module to inhibit the low-quality proposals with the guidance of the completeness pseudo labels, and 3) an instance-level rank consistency loss to achieve robust detection by leveraging the complementarity of RGB and FLOW modalities. Extensive experimental results on two challenging benchmarks including THUMOS14 and ActivityNet demonstrate the superior performance of our method. Our code is available at github.com/RenHuan1999/CVPR2023_P-MIL.
更多查看译文
关键词
Action Recognition,Visual Question Answering,Video Analysis,Pose Estimation
AI 理解论文
溯源树
样例
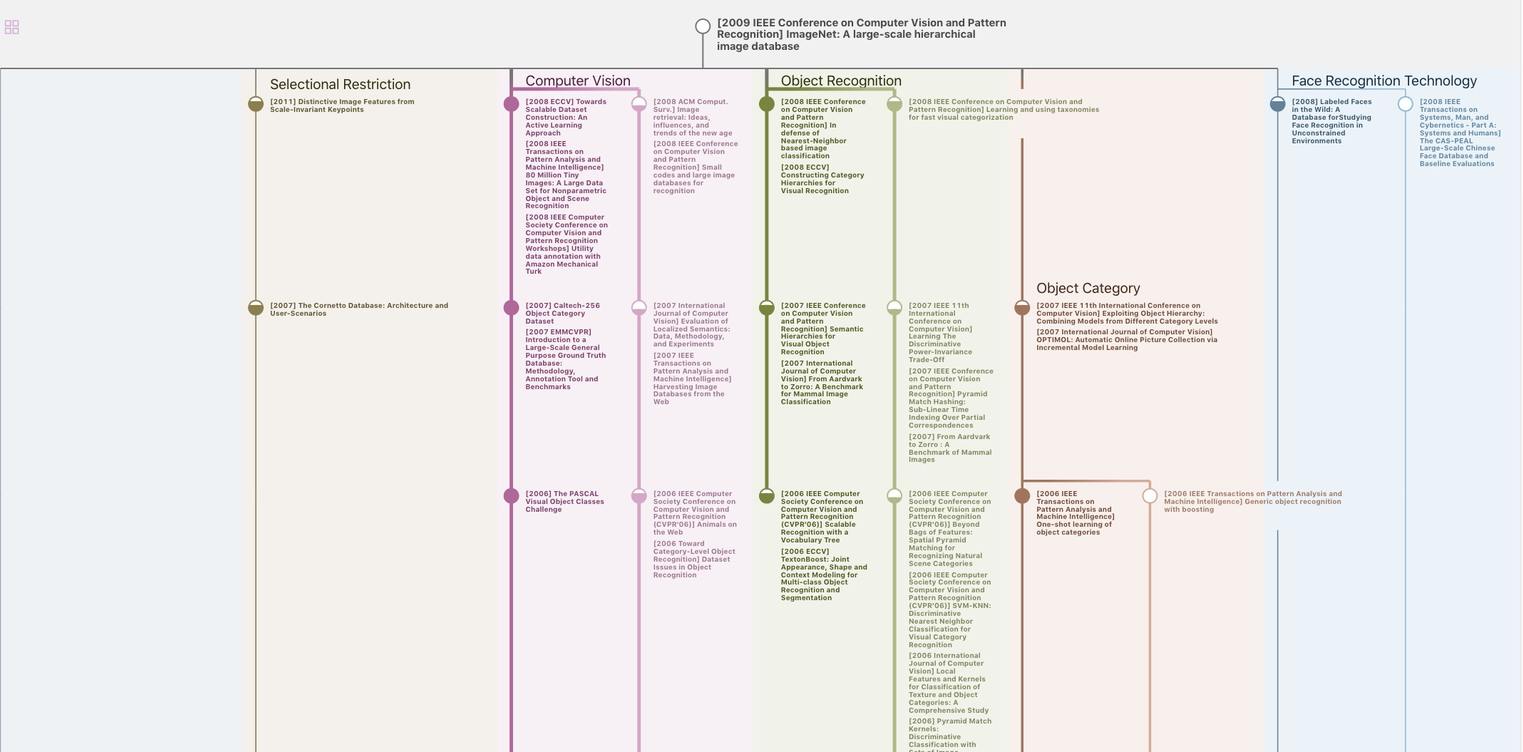
生成溯源树,研究论文发展脉络
Chat Paper
正在生成论文摘要