Tuning parameter selection for nonparametric derivative estimation in random design
STATISTICS(2023)
摘要
Estimation of a function, or its derivatives via nonparametric regression requires selection of one or more tuning parameters. In the present work, we propose a tuning parameter selection criterion called DCp for nonparametric derivative estimation in random design. Our criterion is general in that it can be applied with any nonparametric estimation method which is linear in the observed outcomes. Charnigo et al. [A generalized $ C_p $ Cp criterion for derivative estimation. Technometrics. 2011;53(3):238-253] had proposed a GCp criterion for a similar purpose, assuming values of the covariate to be fixed and constant error variance. Here we consider the setting with random design and non-constant error variance since the covariate values will not generally be fixed and equally spaced in real data applications. We justify DCp in this setting both theoretically and by simulation. We also illustrate use of DCp with two economics data sets.
更多查看译文
关键词
nonparametric derivative estimation,parameter selection
AI 理解论文
溯源树
样例
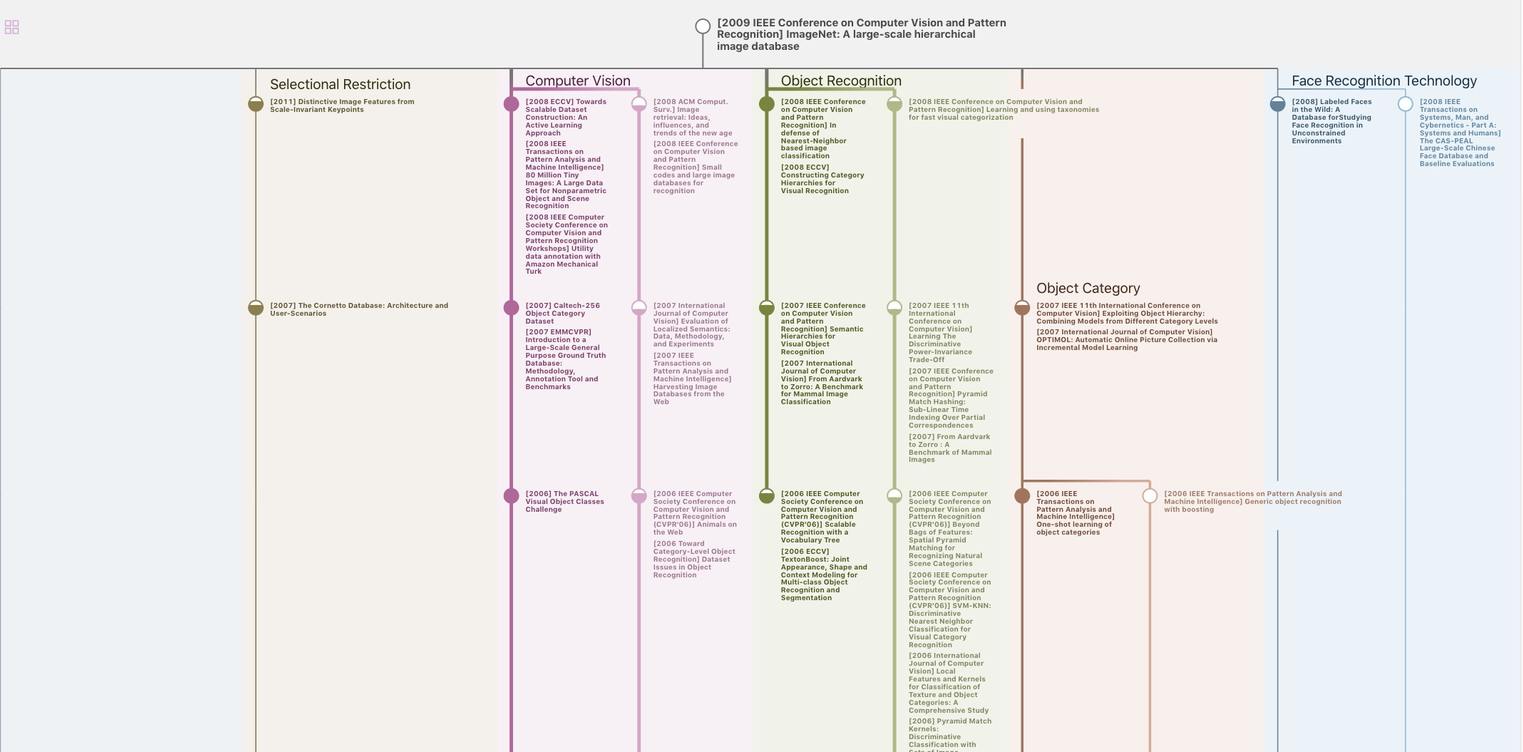
生成溯源树,研究论文发展脉络
Chat Paper
正在生成论文摘要