Improving neural ordinary differential equations via knowledge distillation
IET COMPUTER VISION(2024)
摘要
Neural ordinary differential equations (ODEs) (Neural ODEs) construct the continuous dynamics of hidden units using ODEs specified by a neural network, demonstrating promising results on many tasks. However, Neural ODEs still do not perform well on image recognition tasks. The possible reason is that the one-hot encoding vector commonly used in Neural ODEs can not provide enough supervised information. A new training based on knowledge distillation is proposed to construct more powerful and robust Neural ODEs fitting image recognition tasks. Specially, the training of Neural ODEs is modelled into a teacher-student learning process, in which ResNets are proposed as the teacher model to provide richer supervised information. The experimental results show that the new training manner can improve the classification accuracy of Neural ODEs by 5.17%, 24.75%, 7.20%, and 8.99%, on Street View House Numbers, CIFAR10, CIFAR100, and Food-101, respectively. In addition, the effect of knowledge distillation is also evaluated in Neural ODEs on robustness against adversarial examples. The authors discover that incorporating knowledge distillation, coupled with the increase of the time horizon, can significantly enhance the robustness of Neural ODEs. The performance improvement is analysed from the perspective of the underlying dynamical system. A new training based on knowledge distillation is proposed to construct more powerful and robust Neural ODEs fitting image recognition tasks. Specially, the training of Neural ODEs is modelled into a teacher-student learning process, in which ResNets is proposed as the teacher model to provide richer supervised information. In addition, the effect of both knowledge distillation and time horizon in Neural ODEs on robustness against adversarial examples is also quantitatively discussed.image
更多查看译文
关键词
image classification,neural nets
AI 理解论文
溯源树
样例
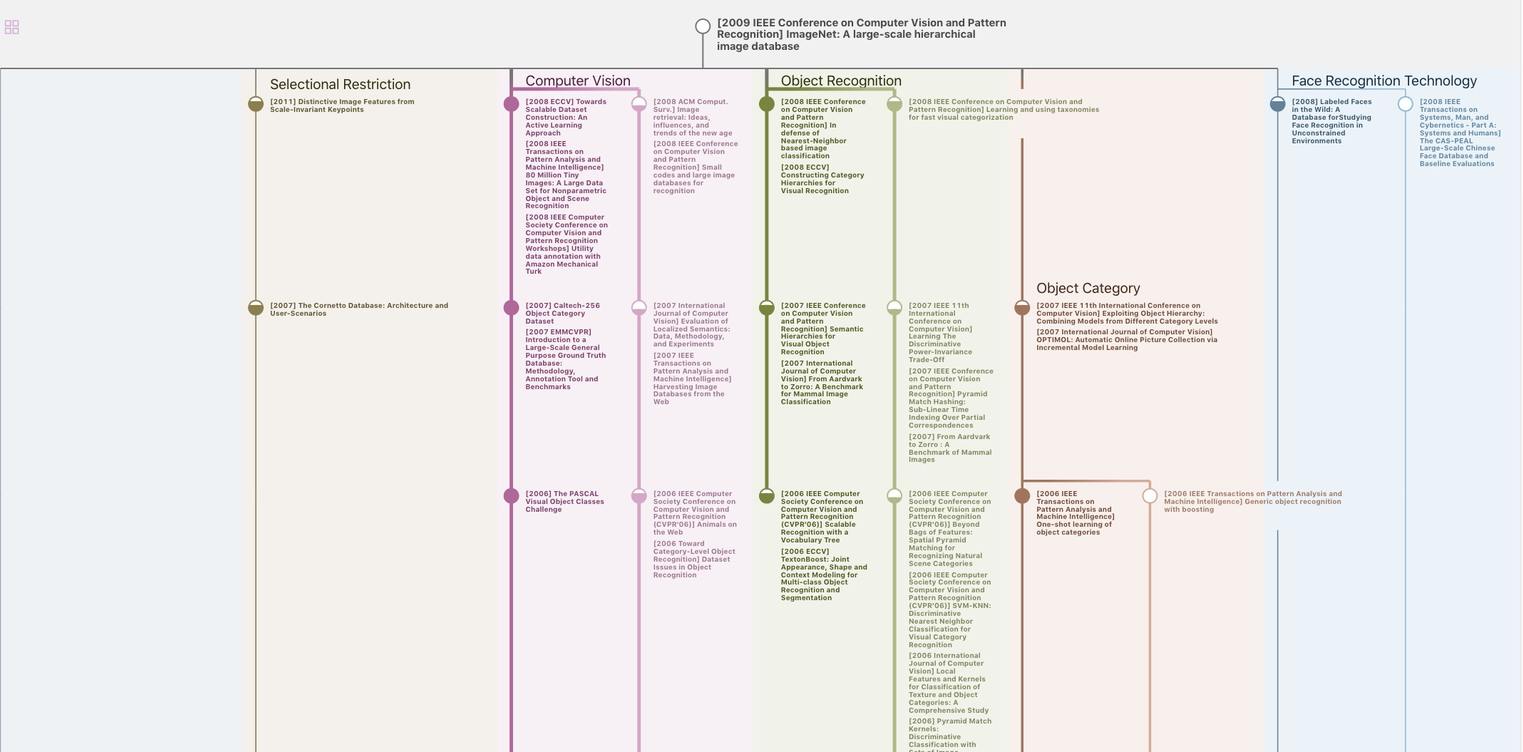
生成溯源树,研究论文发展脉络
Chat Paper
正在生成论文摘要