Design of antimicrobial peptides containing non-proteinogenic amino acids using multi-objective Bayesian optimisation
DIGITAL DISCOVERY(2023)
摘要
Antimicrobial peptides (AMPs) have attracted attention as next-generation antimicrobial drugs. Designing AMPs while considering multiple properties, such as antimicrobial activities and toxicity, requires numerous trials and errors by chemists. In this study, we propose MODAN, a machine learning-assisted AMP design framework based on multi-objective Bayesian optimisation. The primary advantage of MODAN is its ability to handle various non-proteinogenic amino acids, which have recently shown the potential of activity enhancement, and this flexibility has not been achieved by previous studies. In addition, multi-objective Bayesian optimisation enables simultaneous improvement of antimicrobial activity and toxicity. We have succeeded in designing peptides that have potent antimicrobial and low haemolytic activities within two rounds of MODAN recommendation and experimentation, based on a strategy that chemists do not usually consider. MODAN is a multi-objective Bayesian framework for automated design of antimicrobial peptides containing various non-proteinogenic amino acids and side-chain stapling.
更多查看译文
关键词
antimicrobial peptides,amino acids,optimisation,non-proteinogenic,multi-objective
AI 理解论文
溯源树
样例
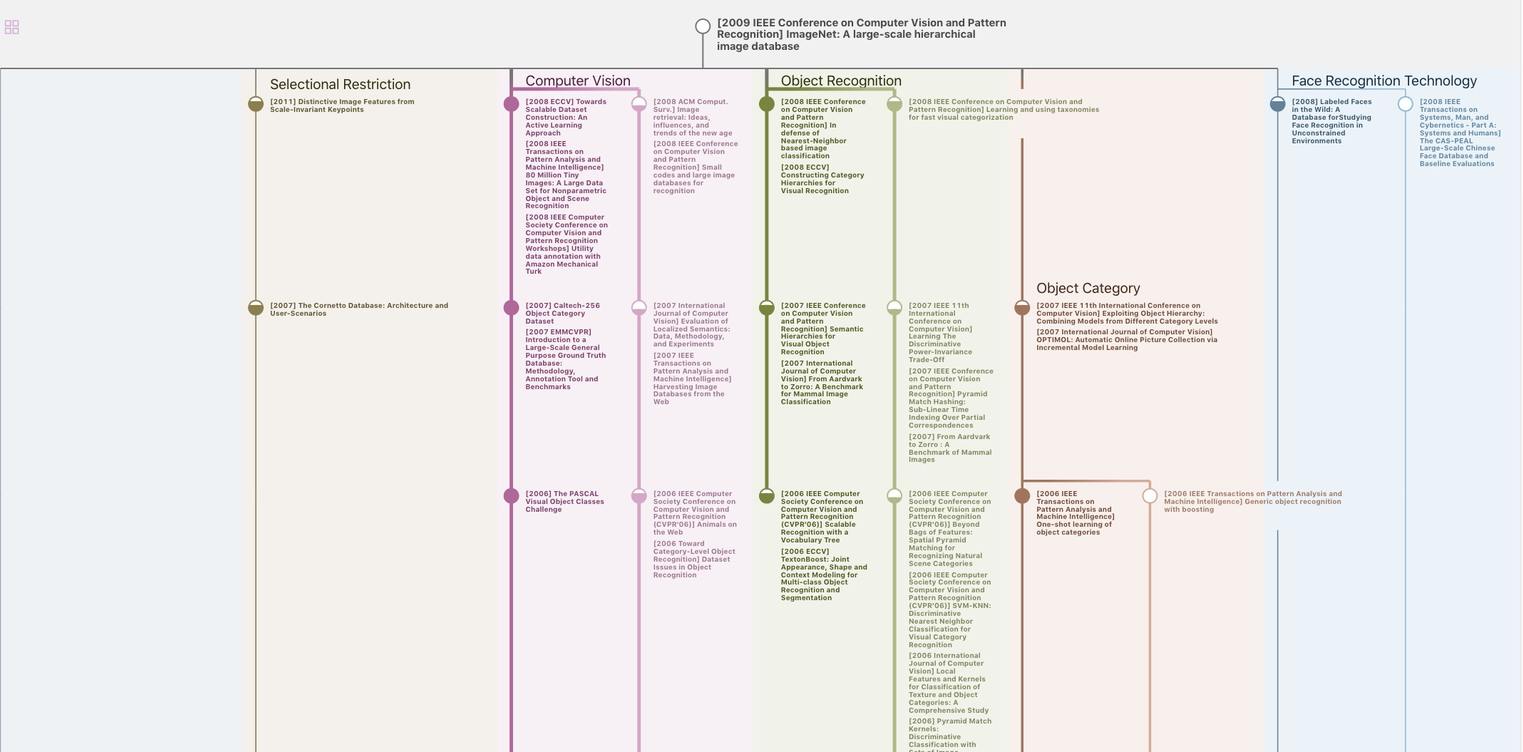
生成溯源树,研究论文发展脉络
Chat Paper
正在生成论文摘要