TransPCGC: Point Cloud Geometry Compression Based on Transformers.
Algorithms(2023)
摘要
Due to the often substantial size of the real-world point cloud data, efficient transmission and storage have become critical concerns. Point cloud compression plays a decisive role in addressing these challenges. Recognizing the importance of capturing global information within point cloud data for effective compression, many existing point cloud compression methods overlook this crucial aspect. To tackle this oversight, we propose an innovative end-to-end point cloud compression method designed to extract both global and local information. Our method includes a novel Transformer module to extract rich features from the point cloud. Utilization of a pooling operation that requires no learnable parameters as a token mixer for computing long-distance dependencies ensures global feature extraction while significantly reducing both computations and parameters. Furthermore, we employ convolutional layers for feature extraction. These layers not only preserve the spatial structure of the point cloud, but also offer the advantage of parameter independence from the input point cloud size, resulting in a substantial reduction in parameters. Our experimental results demonstrate the effectiveness of the proposed TransPCGC network. It achieves average Bjontegaard Delta Rate (BD-Rate) gains of 85.79% and 80.24% compared to Geometry-based Point Cloud Compression (G-PCC). Additionally, in comparison to the Learned-PCGC network, our approach attains an average BD-Rate gain of 18.26% and 13.83%. Moreover, it is accompanied by a 16% reduction in encoding and decoding time, along with a 50% reduction in model size.
更多查看译文
关键词
point cloud geometry compression,transformers,convolution
AI 理解论文
溯源树
样例
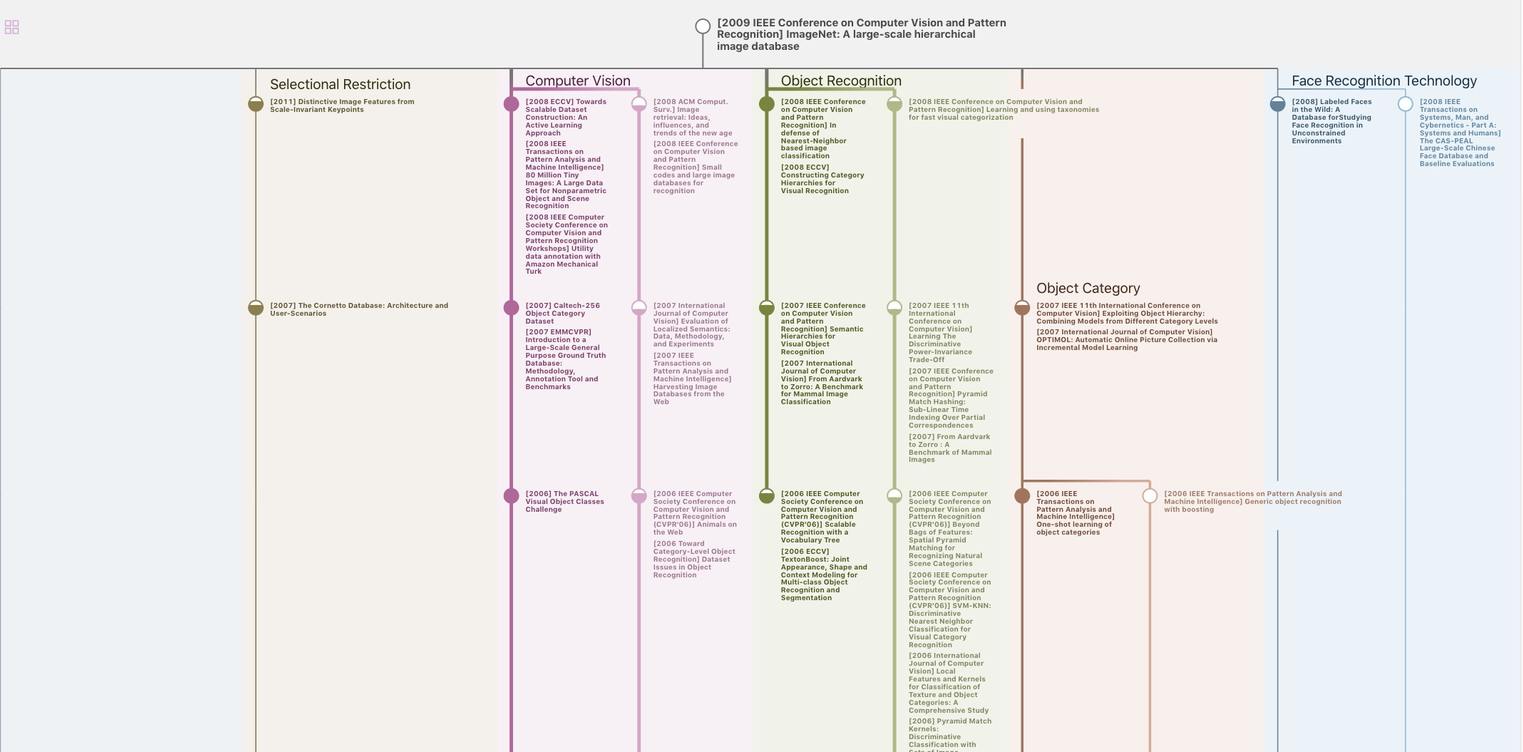
生成溯源树,研究论文发展脉络
Chat Paper
正在生成论文摘要