Development of Maximum Residual Stress Prediction Technique for Shot-Peened Specimen Using Rayleigh Wave Dispersion Data Based on Convolutional Neural Network
Materials(2023)
摘要
Shot peening is a surface treatment process that improves the fatigue life of a material and suppresses cracks by generating residual stress on the surface. The injected small shots create a compressive residual stress layer on the material’s surface. Maximum compressive residual stress occurs at a certain depth, and tensile residual stress gradually occurs as the depth increases. This process is primarily used for nickel-based superalloy steel materials in certain environments, such as the aerospace industry and nuclear power fields. To prevent such a severe accident due to the high-temperature and high-pressure environment, evaluating the residual stress of shot-peened materials is essential in evaluating the soundness of the material. Representative methods for evaluating residual stress include perforation strain gauge analysis, X-ray diffraction (XRD), and ultrasonic testing. Among them, ultrasonic testing is a representative, non-destructive evaluation method, and residual stress can be estimated using a Rayleigh wave. Therefore, in this study, the maximum compressive residual stress value of the peened Inconel 718 specimen was predicted using a prediction convolutional neural network (CNN) based on the relationship between Rayleigh wave dispersion and stress distribution on the specimen. By analyzing the residual stress distribution in the depth direction generated in the model from various studies in the literature, 173 residual stress distributions were generated using the Gaussian function and factorial design approach. The distribution generated using the relationship was converted into 173 Rayleigh wave dispersion data to be used as a database for the CNN model. The CNN model was learned through this database, and performance was verified using validation data. The adopted Rayleigh wave dispersion and convolutional neural network procedures demonstrate the ability to predict the maximum compressive residual stress in the peened specimen.
更多查看译文
关键词
shot peened,residual stress,Rayleigh wave,convolutional neural network,Inconel 718
AI 理解论文
溯源树
样例
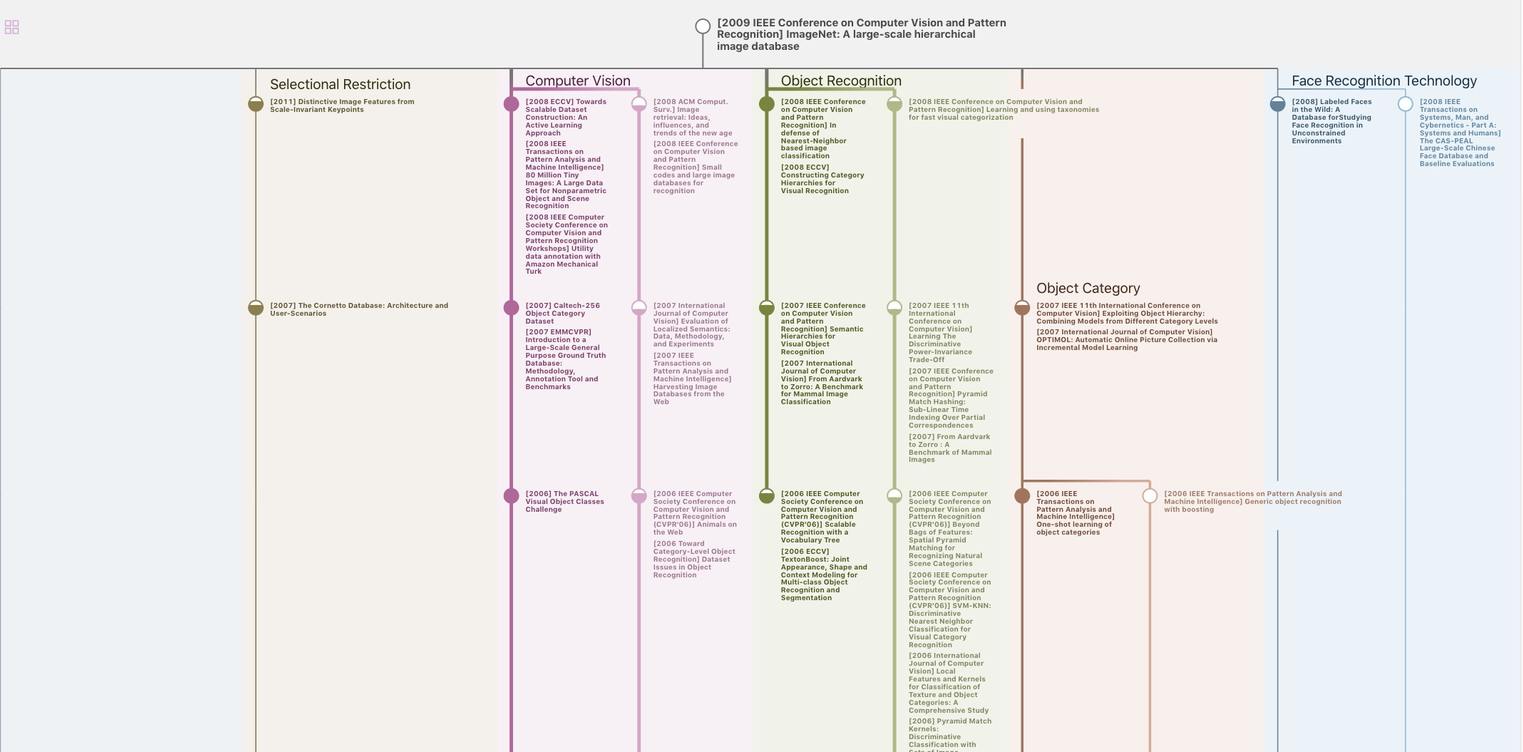
生成溯源树,研究论文发展脉络
Chat Paper
正在生成论文摘要