SiamPKHT: Hyperspectral Siamese Tracking Based on Pyramid Shuffle Attention and Knowledge Distillation
SENSORS(2023)
Abstract
Hyperspectral images provide a wealth of spectral and spatial information, offering significant advantages for the purpose of tracking objects. However, Siamese trackers are unable to fully exploit spectral features due to the limited number of hyperspectral videos. The high-dimensional nature of hyperspectral images complicates the model training process. In order to address the aforementioned issues, this article proposes a hyperspectral object tracking (HOT) algorithm callled SiamPKHT, which leverages the SiamCAR model by incorporating pyramid shuffle attention (PSA) and knowledge distillation (KD). First, the PSA module employs pyramid convolutions to extract multiscale features. In addition, shuffle attention is adopted to capture relationships between different channels and spatial positions, thereby obtaining good features with a stronger classification performance. Second, KD is introduced under the guidance of a pre-trained RGB tracking model, which deals with the problem of overfitting in HOT. Experiments using HOT2022 data indicate that the designed SiamPKHT achieves better performance compared to the baseline method (SiamCAR) and other state-of-the-art HOT algorithms. It also achieves real-time requirements at 43 frames per second.
MoreTranslated text
Key words
hyperspectral video,target tracking,SiamCAR network,pyramid shuffle attention,knowledge distillation
AI Read Science
Must-Reading Tree
Example
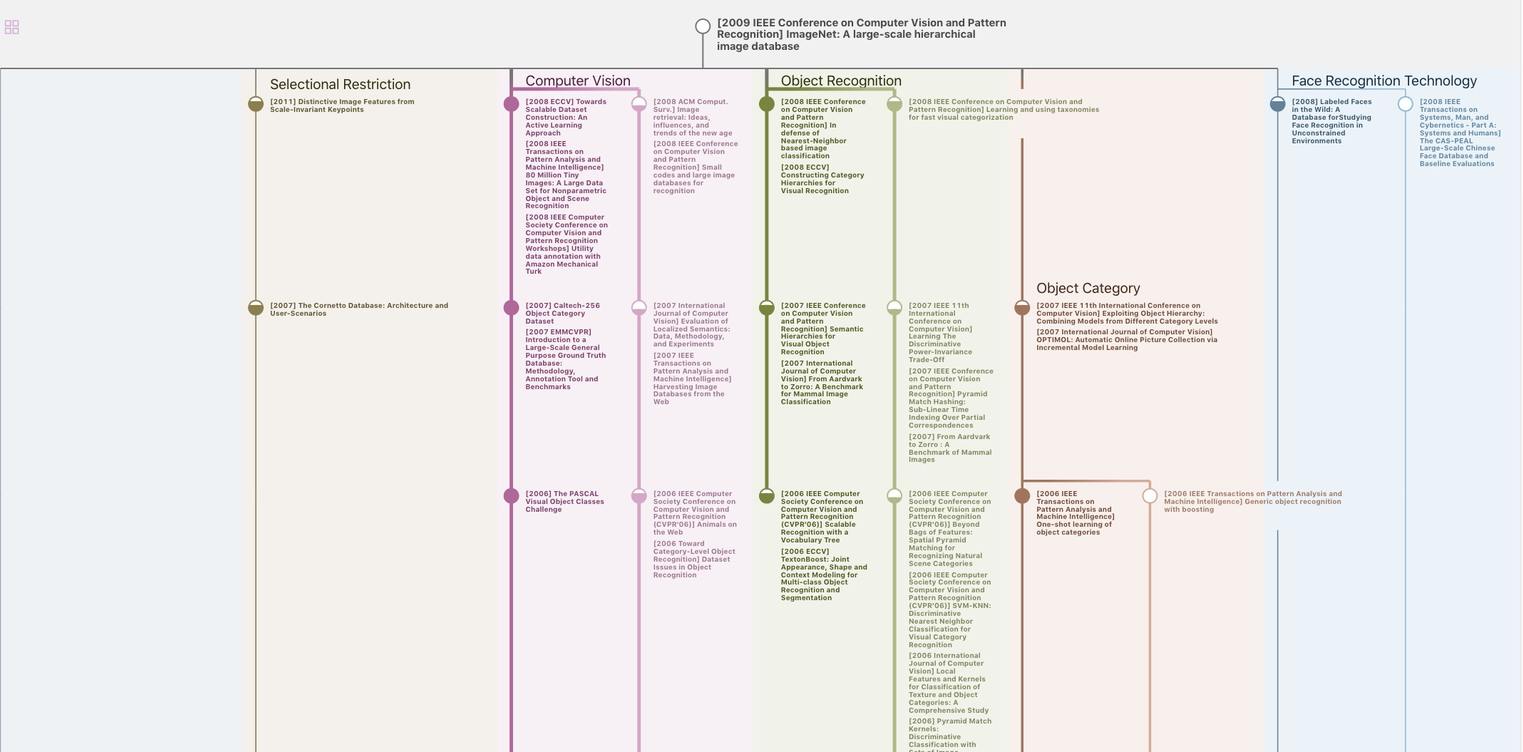
Generate MRT to find the research sequence of this paper
Chat Paper
Summary is being generated by the instructions you defined