Multi-Scale Self-Supervised Graph Contrastive Learning With Injective Node Augmentation
IEEE TRANSACTIONS ON KNOWLEDGE AND DATA ENGINEERING(2024)
摘要
Graph Contrastive Learning (GCL) with Graph Neural Networks (GNN) has emerged as a promising method for learning latent node representations in a self-supervised manner. Most of existing GCL methods employ random sampling for graph view augmentation and maximize the agreement of the node representations between the views. However, the random augmentation manner, which is likely to produce very similar graph view samplings, may easily result in incomplete nodal contextual information, thus weakening the discrimination of node representations. To this end, this paper proposes a novel trainable scheme from the perspective of node augmentation, which is theoretically proved to be injective and utilizes the subgraphs consisting of each node with its neighbors to enhance the distinguishability of nodal view. Notably, our proposed scheme tries to enrich node representations via a multi-scale contrastive training that integrates three different levels of training granularity, i.e., subgraph level, graph- and node-level contextual information. In particular, the subgraph-level objective between augmented and original node views is constructed to enhance the discrimination of node representations while graph- and node-level objectives with global and local information from the original graph are developed to improve the generalization ability of representations. Experiment results demonstrate that our framework outperforms existing state-of-the-art baselines and even surpasses several supervised counterparts on four real-world datasets for node classification.
更多查看译文
关键词
Graph contrastive learning,graph representation learning,node augmentation,self-supervised learning
AI 理解论文
溯源树
样例
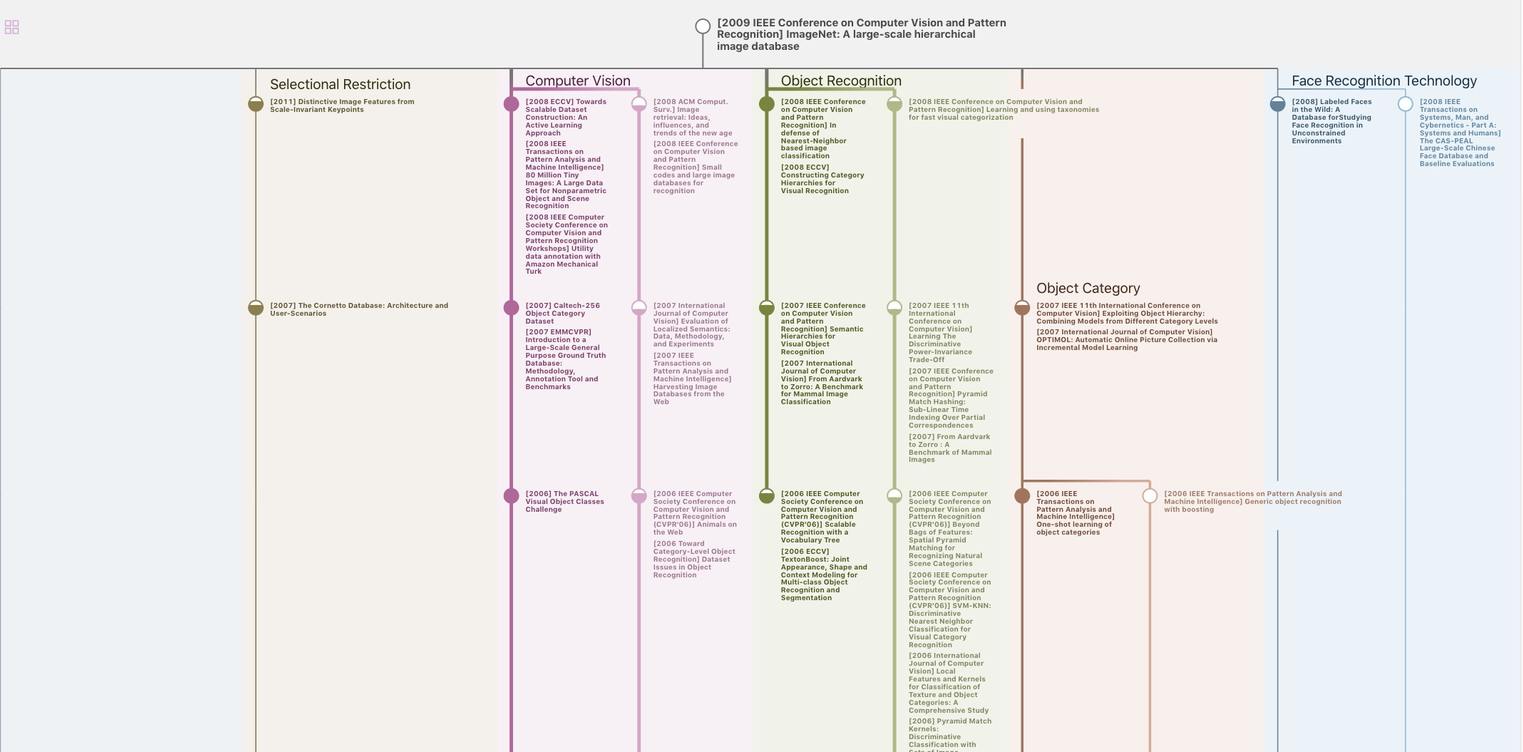
生成溯源树,研究论文发展脉络
Chat Paper
正在生成论文摘要