PUTS: Privacy-Preserving and Utility-Enhancing Framework for Trajectory Synthesization
IEEE TRANSACTIONS ON KNOWLEDGE AND DATA ENGINEERING(2024)
摘要
Vehicle trajectory data is essential for traffic management and location-based services. However, publishing real-life trajectory data has been challenging because vehicle trajectories contain users' sensitive information. Differential privacy addresses such problems by publishing a synthetic version of the input dataset, but existing works always assume the real-world data is absolutely accurate. This assumption no longer holds in trajectory data because it typically contains errors due to inaccurate positioning services, which leads to poor performance of data synthesized by such trajectories. Even worse, existing works may generate unrealistic trajectories due to their coarse data synthesis methods, resulting in low practical utility or even inability to handle complex tasks. In this paper, we propose a Privacy-preserving and Utility-enhancing framework for Trajectory Synthesization (PUTS). Our framework mitigates the impact of data errors in trajectories on differential privacy mechanisms, by exploiting map-matching techniques and real-world road network structure. In PUTS, a two-layer approach from path to trajectory synthesis is proposed to not only guarantee the reality of synthetic trajectories, but also scale up PUTS in real-world applications. Extensive experiments on real-world datasets show that PUTS significantly outperforms existing methods in terms of utility in a range of real-world applications.
更多查看译文
关键词
Differential privacy,Privacy,Urban areas,Task analysis,Publishing,privacy-preserving data publishing,trajectory data mining,trajectory synthesization
AI 理解论文
溯源树
样例
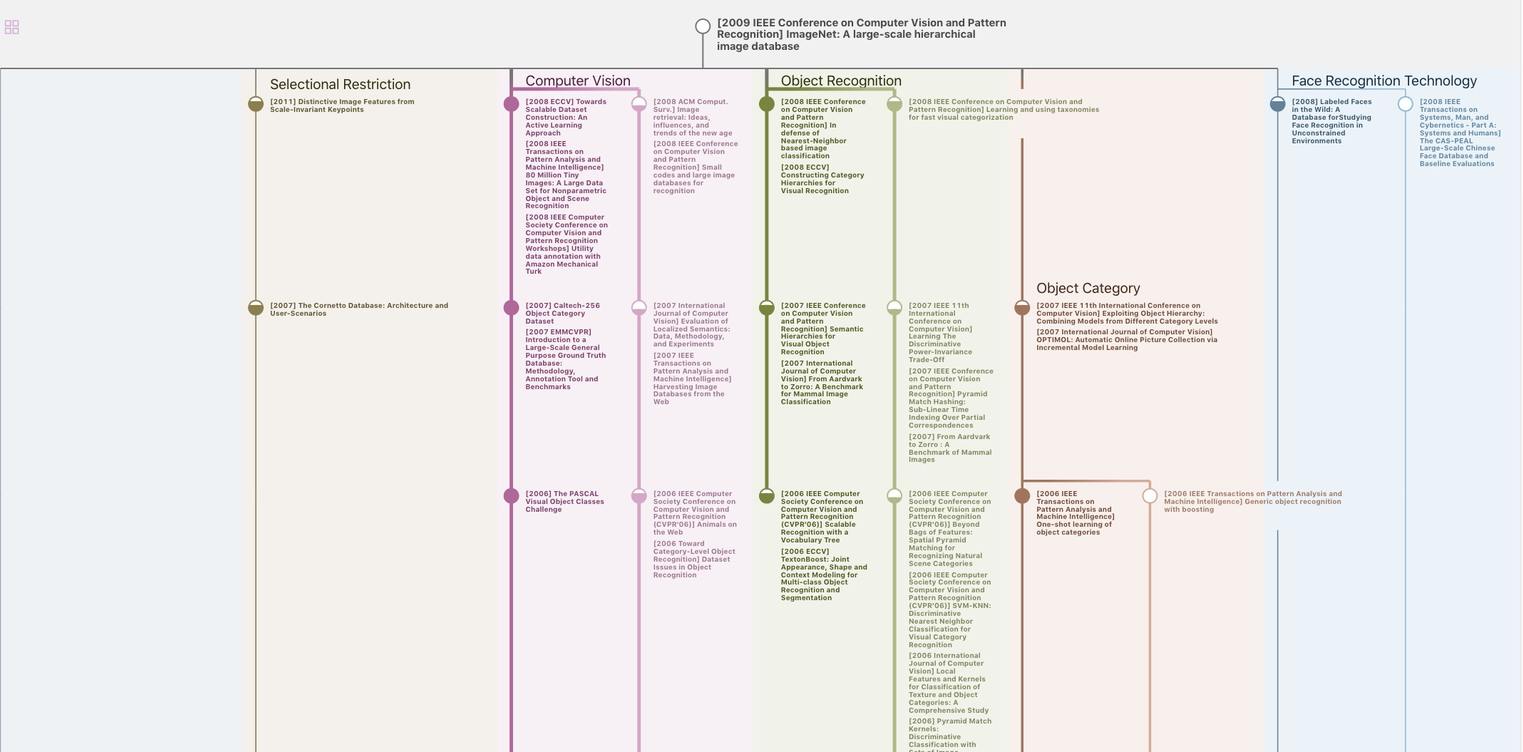
生成溯源树,研究论文发展脉络
Chat Paper
正在生成论文摘要