Customizable Combination of Parameter-Efficient Modules for Multi-Task Learning
ICLR(2024)
摘要
Modular skill learning is an emerging direction in the field of Parameter Efficient Fine-Tuning (PEFT), as it enables neural networks to better organize and clarify various aspects of knowledge, leading to improved knowledge transfer for new tasks. In this paper, we introduce a novel approach that categorizes skills into shared domain skills and specialized skills, with the skill parameters being highly parameterized using low-rank or sparse techniques. Each task is associated with an exclusive specialized skill while also benefiting from shared domain skills. Moreover, tasks can selectively utilize specialized skills from other tasks as needed. To facilitate this approach, we propose a skill assignment matrix that can be jointly learned, and the task network is instantiated based on the skill parameters. To evaluate the effectiveness of our approach, we conducted extensive experiments on the Super Natural Instructions and SuperGLUE datasets. Our results demonstrate that compared to fully-shared, task-specific, or skill-indistinguishable baselines. Modular learning with skill-type discrimination significantly enhances the sample efficiency of multi-task learning. Furthermore, the freezing of a substantial number of base model parameters greatly improves parameter efficiency, leading to boosted training efficiency.
更多查看译文
关键词
Modular skill learning,Multi-task learning,Parameter-Efficient,Fine-Tuning
AI 理解论文
溯源树
样例
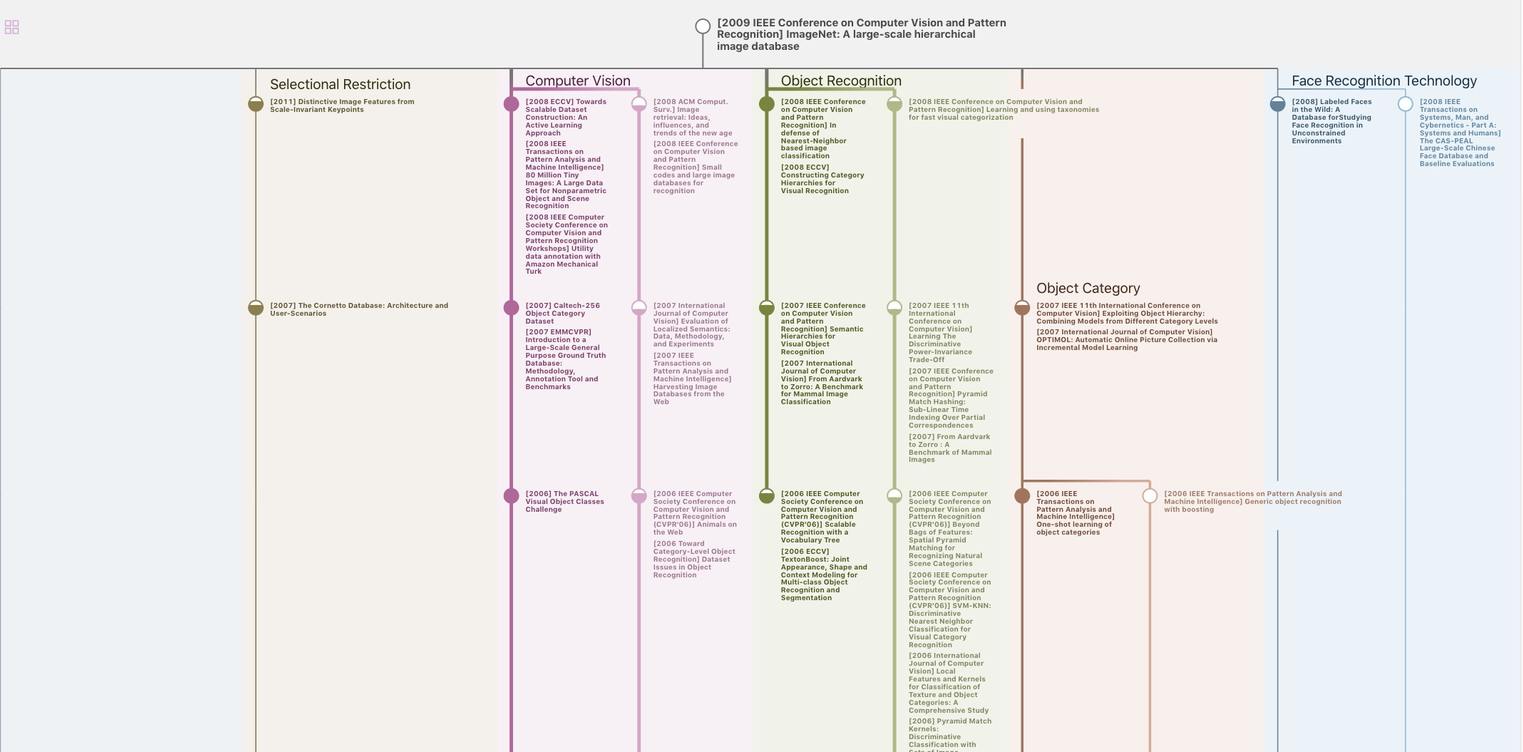
生成溯源树,研究论文发展脉络
Chat Paper
正在生成论文摘要