A Deformable Constraint Transport Network for Optimal Aortic Segmentation from CT Images.
IEEE transactions on medical imaging(2024)
摘要
Aortic segmentation from computed tomography (CT) is crucial for facilitating aortic intervention, as it enables clinicians to visualize aortic anatomy for diagnosis and measurement. However, aortic segmentation faces the challenge of variable geometry in space, as the geometric diversity of different diseases and the geometric transformations that occur between raw and measured images. Existing constraint-based methods can potentially solve the challenge, but they are hindered by two key issues: inaccurate definition of properties and inappropriate topology of transformation in space. In this paper, we propose a deformable constraint transport network (DCTN). The DCTN adaptively extracts aortic features to define intra-image constrained properties and guides topological implementation in space to constrain inter-image geometric transformation between raw and curved planar reformation (CPR) images. The DCTN contains a deformable attention extractor, a geometry-aware decoder and an optimal transport guider. The extractor generates variable patches that preserve semantic integrity and long-range dependency in long-sequence images. The decoder enhances the perception of geometric texture and semantic features, particularly for low-intensity aortic coarctation and false lumen, which removes background interference. The guider explores the geometric discrepancies between raw and CPR images, constructs probability distributions of discrepancies, and matches them with inter-image transformation to guide geometric topology in space. Experimental studies on 267 aortic subjects and four public datasets show the superiority of our DCTN over 23 methods. The results demonstrate DCTN's advantages in aortic segmentation for different types of aortic disease, for different aortic segments, and in the measurement of clinical indexes.
更多查看译文
关键词
Image segmentation,Biomedical imaging,Computed tomography,Feature extraction,Geometry,Topology,Decoding,aortic segmentation,geometric constraint,optimal transport,transformer
AI 理解论文
溯源树
样例
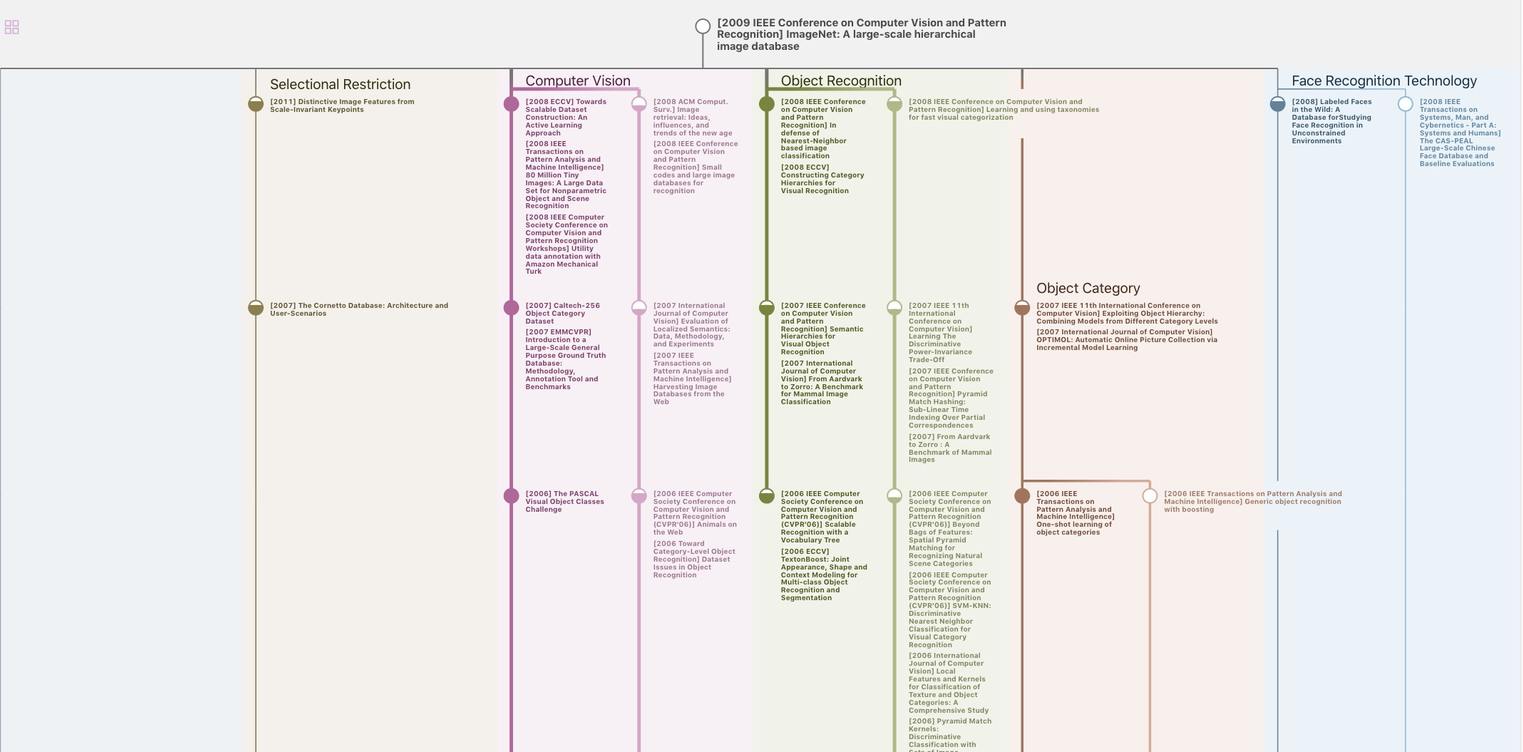
生成溯源树,研究论文发展脉络
Chat Paper
正在生成论文摘要