Performance and Robustness of Machine Learning-based Radiomic COVID-19 Severity Prediction
medRxiv (Cold Spring Harbor Laboratory)(2020)
Abstract
ABSTRACT Objectives This study investigated the performance and robustness of radiomics in predicting COVID-19 severity in a large public cohort. Methods A public dataset of 1110 COVID-19 patients (1 CT/patient) was used. Using CTs and clinical data, each patient was classified into mild, moderate, and severe by two observers: (1) dataset provider and (2) a board-certified radiologist. For each CT, 107 radiomic features were extracted. The dataset was randomly divided into a training (60%) and holdout validation (40%) set. During training, features were selected and combined into a logistic regression model for predicting severe cases from mild and moderate cases. The models were trained and validated on the classifications by both observers. AUC quantified the predictive power of models. To determine model robustness, the trained models was cross-validated on the inter-observer’s classifications. Results A single feature alone was sufficient to predict mild from severe COVID-19 with and (p<< 0.01). The most predictive features were the distribution of small size-zones (GLSZM-SmallAreaEmphasis) for provider’s classification and linear dependency of neighboring voxels (GLCM-Correlation) for radiologist’s classification. Cross-validation showed that both . In predicting moderate from severe COVID-19 , first-order-Median alone had sufficient predictive power of . For radiologist’s classification, the predictive power of the model increased to as the number of features grew from 1 to 5. Cross-validation yielded and . Conclusions Radiomics significantly predicted different levels of COVID-19 severity. The prediction was moderately sensitive to inter-observer classifications, and thus need to be used with caution. Key points Interpretable radiomic features can predict different levels of COVID-19 severity Machine Learning-based radiomic models were moderately sensitive to inter-observer classifications, and thus need to be used with caution
MoreTranslated text
Key words
Predictive Modeling,Radiomics
AI Read Science
Must-Reading Tree
Example
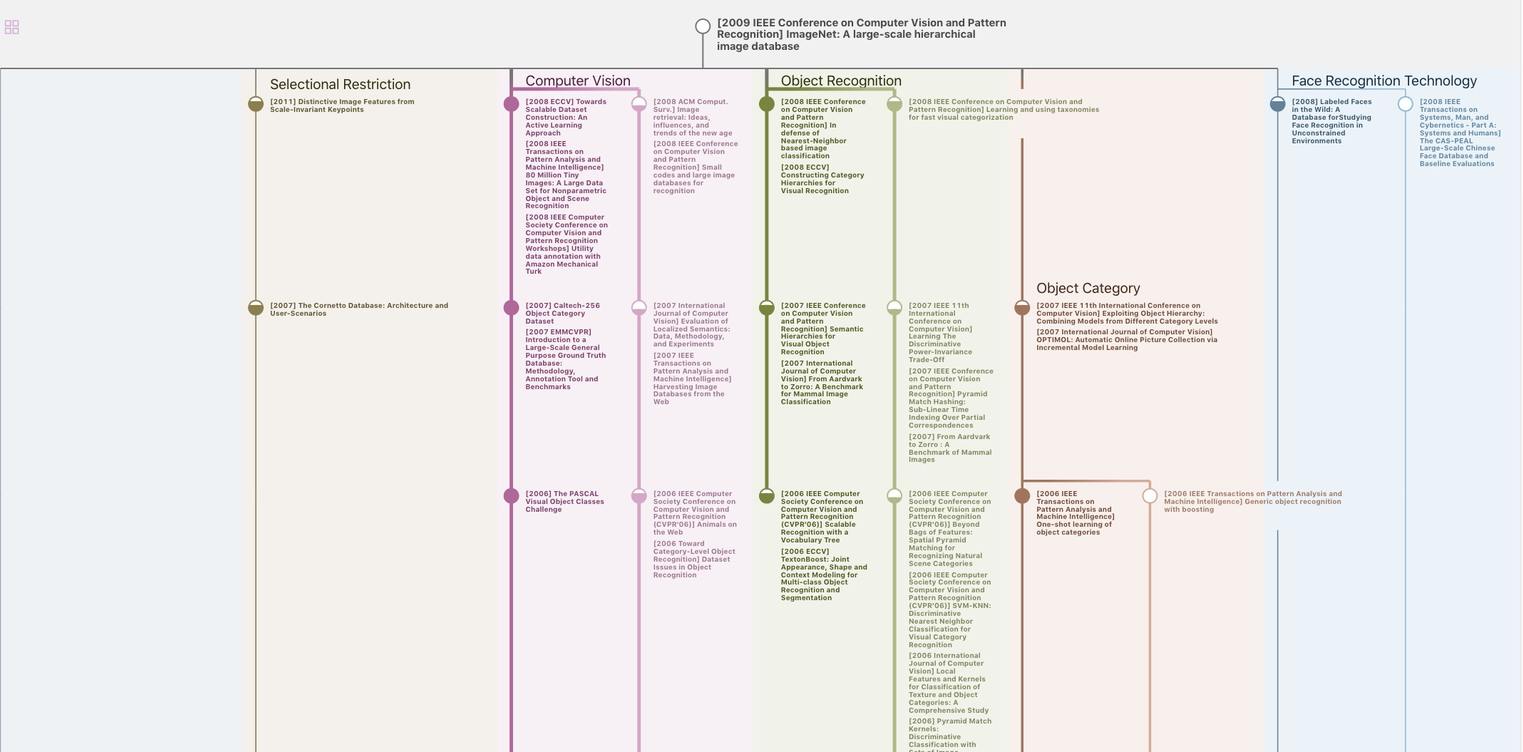
Generate MRT to find the research sequence of this paper
Chat Paper
Summary is being generated by the instructions you defined