Graph structure embedded with physical constraints-based information fusion network for interpretable fault diagnosis of aero-engine
ENERGY(2023)
摘要
Fault diagnosis is essential for ensuring the safety and reliability of aero-engines. Current performance-based fault diagnosis methods typically establish a mapping between measurable parameters and engine states, without taking into account the inherent physical constraints of multimodal information. In this study, a novel graph structure embedded with physical constraints is proposed to effectively fuse sensor information and physical-based model (PBM) simulation information. Since the fault information is primarily manifested in the sensor data rather than the PBM data, the probability distribution between these two types of data can serve as a constraint for constructing the edges in the graph, as it reflects the physical association among the sample points. Selected sensor parameters are used as node characteristics in the graph. A self-supervised representation learning training structure based on graph convolutional network and canonical correlation analysis can effectively utilize labeled and unlabeled data. Furthermore, a robust statistics method is embedded into the training architecture to interpret the behavior of the model by identifying the impact of data samples. The verification results indicate that the proposed model can effectively perform multimodal information fusion and achieve more efficient and high-accuracy component-level fault diagnosis.
更多查看译文
关键词
Physical constraint,Graph structure,Probability distribution,Information fusion
AI 理解论文
溯源树
样例
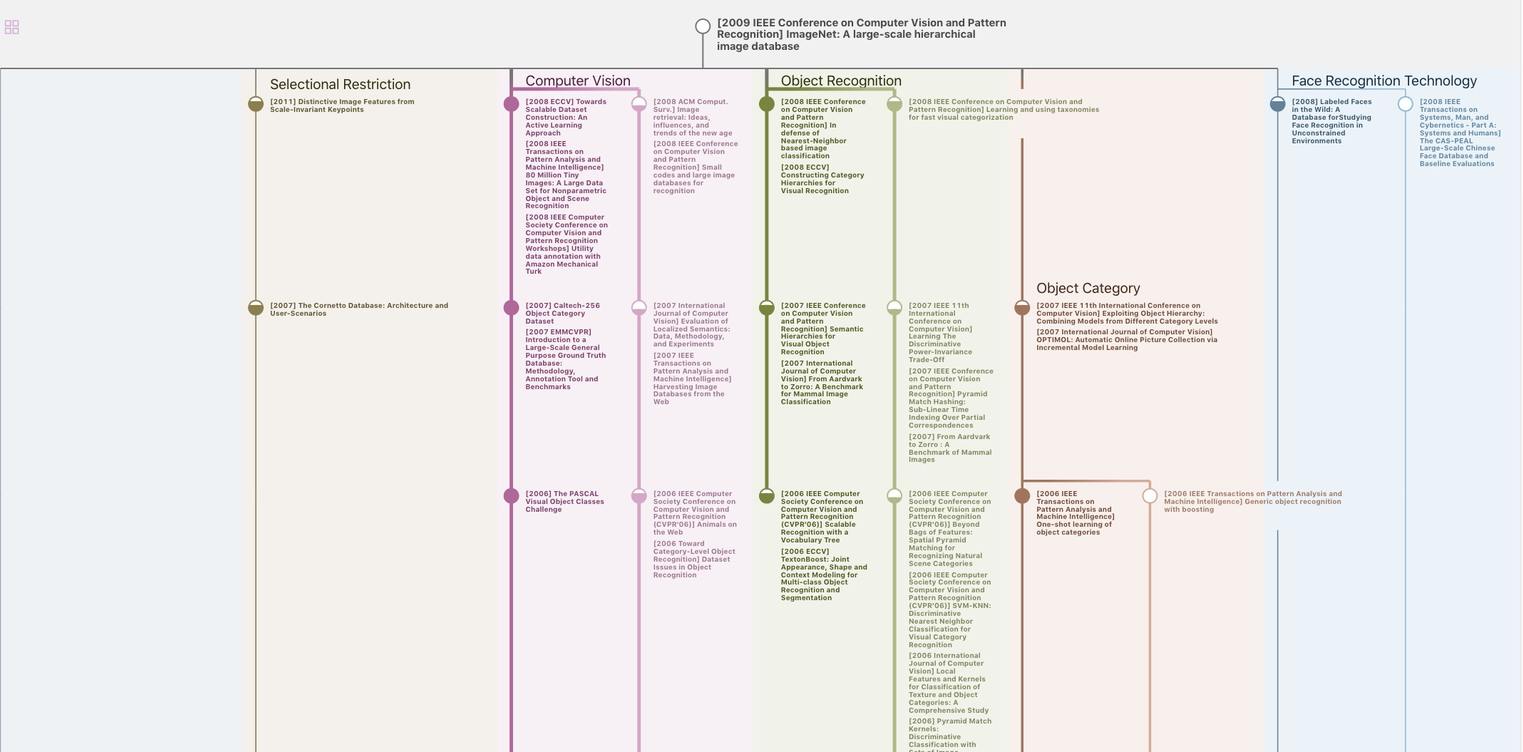
生成溯源树,研究论文发展脉络
Chat Paper
正在生成论文摘要